Article • Innovation in intervention
The promise and reality about AI for interventional oncology
Is artificial intelligence (AI) technology ready to be utilized as a clinical tool by interventional oncologists? Not yet, but when it is, AI technology’s clinical impact may be as profound as advanced imaging is today. This is the consensus of two leading researchers developing AI for interventional oncology (IO) use, who presented back-to-back scientific sessions at ECIO 2021 on both the promise of AI and current concerns and limitations about AI use today.
Report: Cynthia E. Keen
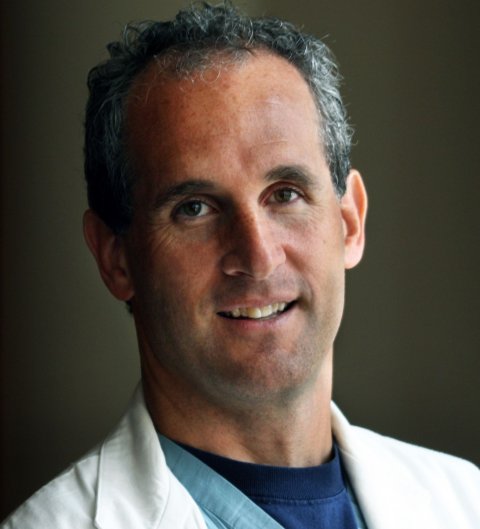
The potential impact of AI in clinical oncology is immense, according to Brad Wood, M.D., director of the National Institutes of Health Center for Interventional Oncology and chief of Interventional Radiology at NIH Clinical Center and the National Cancer Institute in Bethesda, MD. He leads a large multi-disciplinary team of NIH researchers and academic and industry partners that develops devices, software, and navigation approaches for cancer patients via novel local and regional minimally invasive image-guided therapies.
AI will help create accurate predictive models by integrating deep learning to genomic molecular, clinical, histology, imaging and radiomic data. It will be used for staging and response criteria and risk and outcome prediction. AI biomarkers will be used in drug discovery, with the ability to select drugs and predict side effects. This capability will shorten time to drug development, help determine where to biopsy and then correlate image to pathology, explained Dr. Wood.
In imaging, AI will help standardize and automate image interpretation and cancer detection in face of widely variable radiology and pathology human practices. Examples of this include tumor stratification and cost-effective classification, and auto-segmentation and registration for interventions and followup.
Dr. Wood cited an investigation of the impact AI could have on clinical trial eligibility. Researchers Genetech and Stanford University’s Department of Electrical Engineering evaluated AI software called Trial Pathfinder to emulate completed clinical trials of advanced non-small-cell lung cancer using data from electronic health records of over 61,000 patients. AI-defined inclusion criteria doubled the number of eligible patients and improved the hazard ratio, suggesting that many patients who were ineligible to participate in the clinical trials could have potentially benefitted from the treatments.
Recommended article
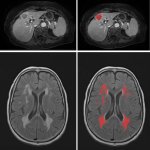
Article • At ECR 2021
AI experts tackle organ segmentation and health economics
AI is revamping workflows and experts showed how radiologists can integrate it into their department to improve daily practice and healthcare at ECR. The panel also discussed the health economics side of AI to help radiologists define which products make more economic sense for their department. The session tackled automated organ segmentation, an interesting application for AI in radiology.
AI has the ability to navigate through a huge number of images that is infeasible for a human researcher and has the potential to make clinical assessments. These will include detection of abnormalities and characterization of them, such as segmentation defining the boundary extent of abnormality for subsequent diagnosis and treatment. AI tools will be able to evaluate and classify abnormalities as malignant or benign, and to stage them into multiple predefined categories. AI will aid change analysis, by tracking object characteristics across multiple temporal scans and across multiple modalities for diagnosis as well as evaluating treatment response. “All this will happen, but it is easier said than done, and there are a lot of pitfalls,” Dr. Wood said.
AI: A “data wrangler” of the future
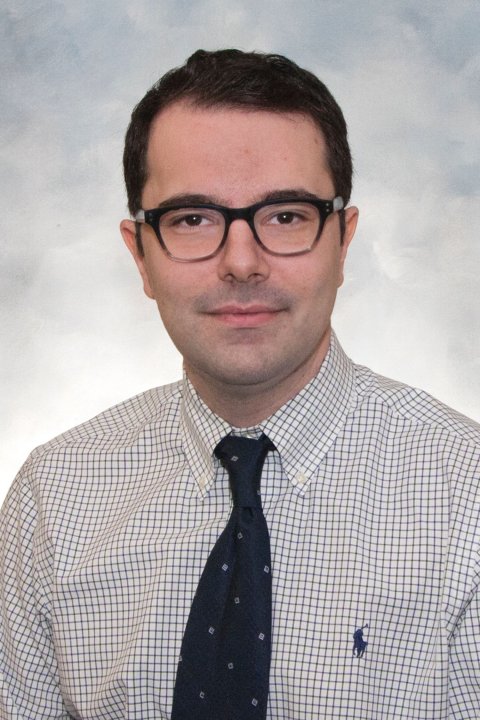
Julius Chapiro, M.D., Ph.D., co-director of the Yale Interventional Oncology Research Lab of the Department of Radiology and Biomedical Imaging at the Yale University School of Medicine in New Haven, CT, agrees. He leads an interdisciplinary team of basic, translational, and clinical scientists who are developing new quantitative imaging biomarkers, predictive instruments, and imaging techniques for the diagnosis, characterization and image-guided therapy of hepatocellular carcinoma (HCC).
Tumor treatment management today is based on inadvertent biased interpretation of evidence. Decisions are made based on evidence accrued from experience and knowledge reflective of an individual physicians’ perceptions. There are more than 25 unclear, varying, and inconsistent diagnostic imaging guidelines. No unified staging system for HCC exists, and the wide variety of loco-regional therapy options are all supported by different data. “The hypothesis of utilizing AI is that it is exclusively data driven and therefore neutral to the personal cognitive bias of a physician. But is this really true? Can AI help us break out of the vicious unintended circle of bias? The problem is that any AI decision support tool will only be as good as the data that we use to train it,” Dr. Chapiro explained.
Large amounts of high-quality, annotated curated data are needed from multiple sources. Selecting the data to use is very complex; the quality of data is key. A large data volume alone does not guarantee success. The question of explaining what decisions are made, why the AI tool does what it does, and why it is needed also must be addressed. No algorithm will be clinically applicable unless it can be independently verified. “Is the problem that an AI tool resolves worth solving?” queried Dr. Chapiro. “AI cannot solve every problem in medicine, and certainly not in IO. AI tool developers need to start with problems that have sufficient data to begin with, of frequently encountered pathologies with high incidence rates rather than rare findings.
Evaluating an AI software tool
Other questions to ask include when evaluating an AI tool for IO include:
- Was standardized reporting used?
- Is the study methodology respecified?
- What are the sources of data and what were the measurement benchmarks for quality?
- How is data split between AI tool development and validation?
- Was the dataset used in model training reflective of clinical reality and intended use case?
- Was the output of the model interpretable?
- Is the performance reproducible and generalizable?
Dr. Wood discussed similar issues. “To realize the promise of AI in IO, we must define the clinical task, which is easier said than done. We must determine where AI is most likely to have a positive impact. We need multi-disciplinary teams to create AI tools. And most importantly, uniformly standardized data needs to be shared throughout the world, through registries, research databases, and reporting.
Federated learning is one method to train AI models that are generalizable and reproducible with data from multiple sources while maintaining anonymity of the data. Dr. Wood cites a collaboration by 20 institutions to create an AI tool that predicts future oxygen requirements of patients infected with Covid-19. He also explained how a CT AI Covid-19 detection and classification model was trained to be generalizable across nations. Simple AI models can be building blocks that feed larger blocks to create more complex AI models, he emphasized. This is happening now.
Recommended article
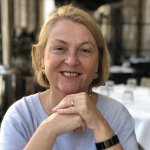
Article • Coronavirus imaging
Covid-19: Is CT more sensitive than PCR testing?
Covid-19 causes characteristic changes in lung tissue visible in CT scans and chest radiographs, known as “ground-glass” opacities. Imaging is now considered a valid alternative, possibly even superior to RT-PCR. ‘This sparked an international debate about the role of CT in the diagnostic work-up of Covid-19,’ said radiologist Professor Cornelia Schäfer-Prokop.
Like diagnostic imaging, AI tools offer immense promise to improve IO. This is starting to happen now, and probably will steeply accelerate in ensuing years. Right now, however, it’s important to keep knowledge about AI utilization in perspective.
06.05.2021