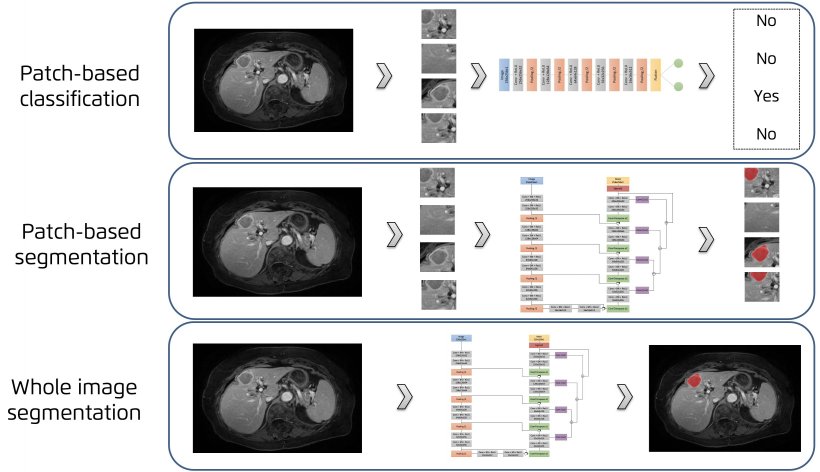
© Quibim
Article • At ECR 2021
AI experts tackle organ segmentation and health economics
AI is revamping workflows and experts showed how radiologists can integrate it into their department to improve daily practice and healthcare at ECR. The panel also discussed the health economics side of AI to help radiologists define which products make more economic sense for their department.
Report: Mélisande Rouger
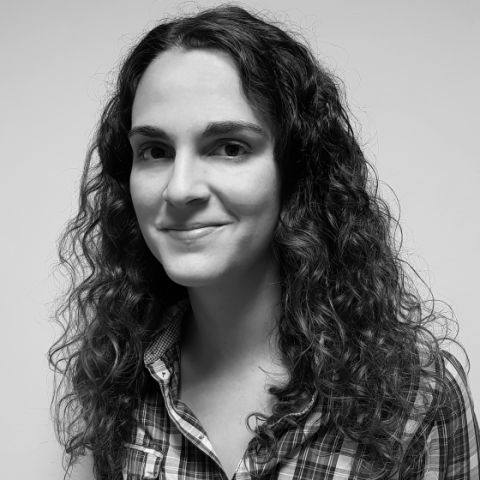
The session tackled automated organ segmentation, an interesting application for AI in radiology. It can optimize the examination in more than one way, by reducing reading time and bringing new information to the table, according to Ana Jiménez Pastor, a telecommunications biomedical engineer and PhD candidate at the Polytechnic University of Valencia, Spain. Jimenez told ECR delegates how she has been working on developing models for automated image analysis to free radiologists from a dull, tedious task. “Segmenting organs manually is time consuming and radiologists often complain about it,” she said. “AI segmentation takes that pain away. It takes about three minutes to segment the prostate on one slide manually, but with AI, you can do that in four seconds.”
Jiménez presented the main AI network architectures that allow for multiple-organs segmentation and the novel techniques for automated lesions segmentation, such as the use of ensembles for refined white matter lesions segmentation or multi-planar configurations to enrich the solution of contextual information.
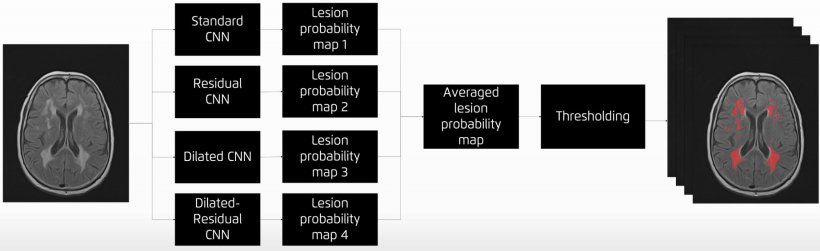
© Quibim
When radiologists have to image patients regularly or with large volumes of sequences, delegating segmentation to an algorithm can help save hours of work, which could be used much more efficiently for diagnostic or training purposes.
Deep learning organ segmentation can also help radiologists make a more impacting contribution to patient management. In clinical practice, radiologists usually don’t have time to manually segment more than one region of interest (ROI) within the organ. But segmenting the whole organ could help them extract imaging data that, once correlated with biological findings, could generate more meaningful insights and optimize the examination, Jiménez explained. “In the liver, fat is distributed in different regions,” she said. “If you don’t segment the whole liver, you might be missing information. You might not even be analyzing the most relevant part of the organ for your examination. This might lead to underestimating fat concentrations and compromise your whole examination.”
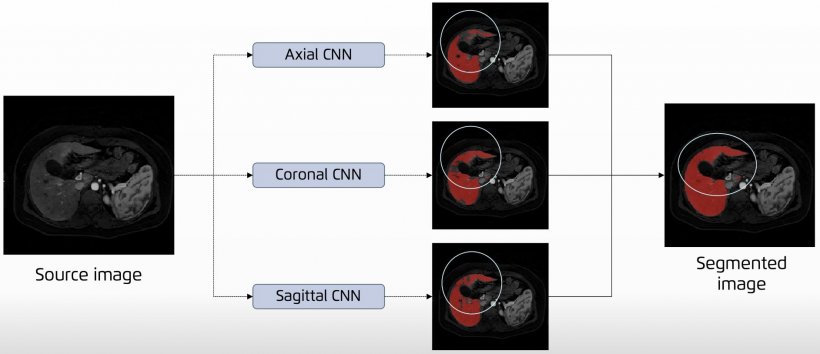
© Quibim
Automatic segmentation of whole tissues is particularly useful in metabolic and hepatic diseases and oncology, especially at the time of administrating pharmaceutical treatment. “Being able of measuring obesity or fat/muscle deficit is crucial to determine drug dose based on the patient’s information,” said Jiménez, who is also working on advancing knowledge of pediatric tumors and prostate cancer with AI in the EU-funded PRIMAGE and ProCancer I projects.
Recommended article
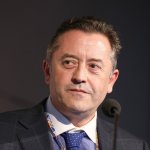
Article • PRIMAGE project
Aiming AI at lethal paediatric tumours
La Fe University and Polytechnic Hospital in Valencia, Spain, is coordinating EU-funded program PRIMAGE, which uses precision information from medical imaging to advance knowledge of the most lethal paediatric tumours, by establishing their prognosis and expected treatment response using radiomics, imaging biomarkers and artificial intelligence (AI).
Hugh Harvey, a consultant for digital solutions in healthcare near London, UK, talked about the health economics side of AI, by focusing on the key items a company should check to get its product into the market. “You need to have a good idea, it’s going to be proven to work through clinical studies and trials, you need regulatory approval and your intellectual property must be all sorted out,” he said. “But then you also need to know how much your solution is going to cost and save to your customers and the healthcare system overall.”
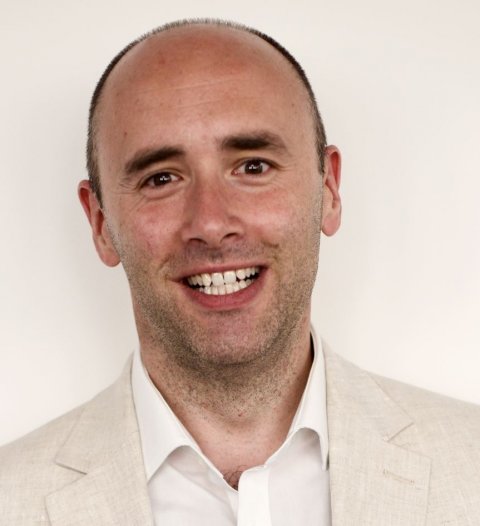
Managers should have a good idea what an AI tool can help save to their department and how much it’s going to benefit other departments as well. “The CE mark doesn’t say it makes economic sense to buy it,” Harvey said. “The radiology department needs to know what it’s going to cost them, especially when others may benefit from it.” Measuring the cost-benefit ratio can be broken down into quality of life (QoL) units, cost models and human factor analysis, and then ranged into different scenarios that will highlight the tenuous links between a disease and its context.
Other speakers in the session (Tim Leiner from Utrecht/NL and Bram Stieltjes from Basle/CH) focused on how different AI systems can be integrated to support workflow and how AI can be used in detection and classification in workflow prioritisation.
Profiles:
Ana Jiménez Pastor is a telecommunications engineer with a master’s degree in biomedical engineering from the Polytechnic University of Valencia, where she is completing her PhD in the field of AI and imaging biomarkers for the study of metabolic and hepatic diseases. Since 2017 she has worked as an R&D Engineer at Quibim, a medtech company that extracts imaging biomarkers from medical images using radiomics and AI. She focuses on the development of algorithms for the analysis and quantification of medical images from clinical question to integration in clinical practice. She is an active collaborator in the Biomedical Imaging Research Group (GIBI230), La Fe Hospital Health Research Institute in Valencia. She has authored and co-authored four publications and two book chapters, and has presented her research in several oral communications worldwide.
Hugh Harvey is managing director at Hardian Health, a consulting agency for digital solutions in healthcare, in Surrey, UK. He acts as a consultant and advisory board member for AI start-ups in the UK, EU and America within the imaging and healthcare space, and sits on several AI and academic committees, including the KSS Academic Health Science Network AI Core Advisory Group. Harvey worked as a consultant radiologist in the NHS (GSTT) and sat on the Royal College of Radiologists committee for medical imaging informatics as well as the AI working group. He qualified as a radiologist and completed his MD research degree in computational analysis of prostate MR at the Institute of Cancer Research in 2016. He has co-authored several white papers and reports on AI in healthcare and serves as an associate editor at Nature: Digital Medicine. He was the lead for regulatory affairs at Babylon and clinical director at Kheiron Medical, where he helped develop deep learning software to improve breast cancer screening.
11.03.2021