News • New drivers and biomarkers
Cancer: Mapping mutation 'hotspots' reveals key players
Researchers led by bioengineers at the University of California San Diego have identified and characterized a previously unrecognized key player in cancer evolution: clusters of mutations occurring at certain regions of the genome. These clusters contribute to the progression of about 10% of cancers and can be used to predict patient survival.
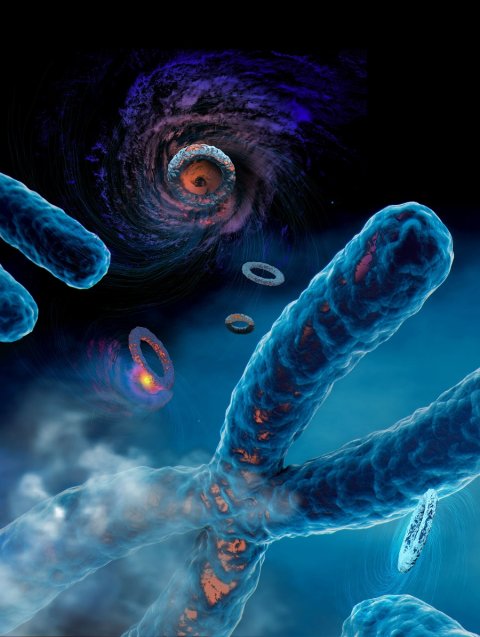
The work sheds light on a class of mutations called clustered somatic mutations—clustered meaning they group together at specific areas in a cell’s genome, and somatic meaning they are not inherited, but caused by internal and external factors such as aging or exposure to UV radiation, for example.
Clustered somatic mutations have so far been an understudied area in cancer development. But researchers in the lab of Ludmil Alexandrov, a professor of bioengineering and cellular and molecular medicine at UC San Diego, saw something highly unusual about these mutations that warranted further study.
“We typically see somatic mutations occurring randomly across the genome. But when we looked closer at some of these mutations, we saw that they were occurring in these hotspots. It’s like throwing balls on the floor and then suddenly seeing them cluster in a single space,” said Alexandrov. “So we couldn’t help but wonder: What is happening here? Why are there hotspots? Are they clinically relevant? Do they tell us something about how cancer has developed?”
“Clustered mutations have largely been ignored because they only make up a very small percentage of all mutations,” said Erik Bergstrom, a bioengineering PhD student in Alexandrov’s lab and the first author of the study. “But by diving deeper, we found that they play an important role in the etiology of human cancer.”
The team’s discoveries were enabled by creating the most comprehensive and detailed map of known clustered somatic mutations. They started by mapping all the mutations (clustered and non-clustered) across the genomes of more than 2500 cancer patients—an effort that in total encompassed 30 different cancer types. The researchers created their map using next-generation artificial intelligence approaches developed in the Alexandrov lab. The team used these algorithms to detect clustered mutations within individual patients and elucidate the underlying mutational processes that give rise to such events. This led to their finding that clustered somatic mutations contribute to cancer evolution in approximately 10% of human cancers.
Taking it a step further, the researchers also found that some of the cancer-driving clusters—specifically those found in known cancer driver genes—can be used to predict the overall survival of a patient. For example, the presence of clustered mutations in the BRAF gene—the most widely observed driver gene in melanoma—results in better overall patient survival compared to individuals with non-clustered mutations. Meanwhile, the presence of clustered mutations in the EGFR gene—the most widely observed driver gene in lung cancer—results in decreased patient survival.
“What’s interesting is that we see differential survival in terms of just having clustered mutations detected within these genes, and this is detectable with existing platforms that are commonly used in the clinic. So this acts as a very simple and precise biomarker for patient survival,” said Bergstrom.
“This elegant work emphasizes the importance of developing AI approaches to elucidate tumor biology, and for biomarker discovery and rapid development using standard platforms with direct line of sight translation to the clinic,” said Scott Lippman, director of Moores Cancer Center and associate vice chancellor for cancer research and care at UC San Diego. “This highlights UC San Diego’s strength in combining engineering approaches in artificial intelligence for solving current problems in cancer medicine.”
A new mode of cancer evolution
In this study, the researchers also identified various factors that cause clustered somatic mutations. These factors include UV radiation, alcohol consumption, tobacco smoking, and most notably, the activity of a set of antiviral enzymes called APOBEC3.
APOBEC3 enzymes are typically found inside cells as part of their internal immune response. Their main job is to chop up any viruses that enter the cell. But in cancer cells, the researchers think that the APOBEC3 enzymes may be doing more harm than good.
The researchers found that cancer cells—which are often rife with circular rings of extrachromosomal DNA (ecDNA) that harbor known cancer driver genes—have clusters of mutations occurring across individual ecDNA molecules. The researchers attribute these mutations to the activity of APOBEC3 enzymes. They hypothesize that APOBEC3 enzymes are mistaking the circular rings of ecDNA as foreign viruses and attempt to restrict and chop them up. In doing so, the APOBEC3 enzymes cause clusters of mutations to form within individual ecDNA molecules. This in turn plays a key role in accelerating cancer evolution and likely leads to drug resistance. The researchers named these rings of clustered mutations kyklonas, which is the Greek word for cyclones.
“This is a completely novel mode of oncogenesis,” said Alexandrov. Along with the team’s other findings, he explained, “this lays the foundation for new therapeutic approaches, where clinicians can consider restricting the activity of APOBEC3 enzymes and/or targeting extrachromosomal DNA for cancer treatment.”
The findings are reported in a paper published in Nature.
Source: University of California - San Diego
11.02.2022