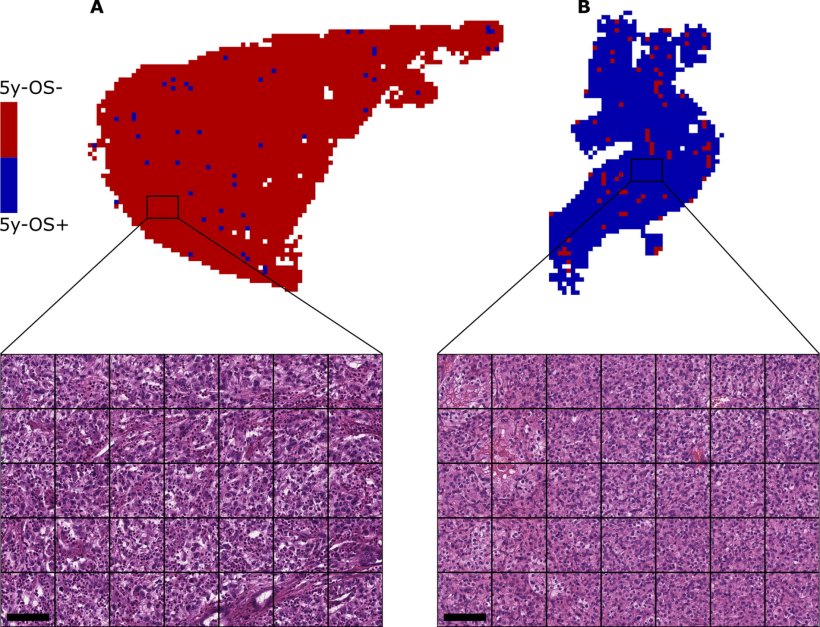
Image source: Wessels et al., PLOS One 2022 (CC BY 4.0)
CNN applications in pathology
‘Deep learning can predict survival directly from histology in clear cell renal cell carcinoma’
Renal cell carcinoma is among the fifteen most common cancers worldwide. Surgical removal of the tumour is recommended for localized stages and oligometastatic cases, while non-resectable metastases require systemic therapy and optional cytoreductive nephrectomy.
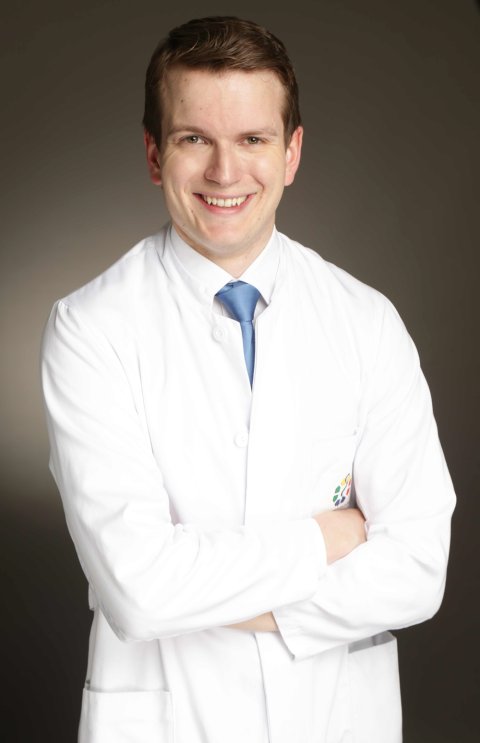
With clear cell renal cell carcinoma, risk-dependent diagnostic and therapeutic algorithms are routinely implemented in clinical practice. ‘Artificial intelligence-based image analysis has the potential to improve outcome prediction and thereby risk stratification. Thus, we looked at whether a convolutional neural network (CNN) can extract relevant image features from a typical H&E-stained slide to predict 5-year overall survival,’ said Dr Titus Brinker, head of Digital Biomarkers for Oncology group at the German Cancer Research Center (DKFZ) in Heidelberg.
His CNN-based prognostication of overall survival demonstrated promising results in a study published in August, with a mean weighted accuracy of 72%, sensitivity of 72.4% and specificity of 71.7%. Its generalizability can be combined with existing clinical pathology parameters. ‘While this widely applicable technique shows the potential of AI in image-based outcome prediction, further research is needed to fine-tune this method and improve robustness,’ Brinker concludes.
20.10.2022