Image source: Unsplash/ThisisEngineering RAEng
News • Discerning good algorithms from bad ones
Medical AI evaluation is surprisingly patchy, study finds
In just the last two years, artificial intelligence has become embedded in scores of medical devices that offer advice to ER doctors, cardiologists, oncologists, and countless other health care providers.
The Food and Drug Administration has approved at least 130 AI-powered medical devices, half of them in the last year alone, and the numbers are certain to surge far higher in the next few years. Several AI devices aim at spotting and alerting doctors to suspected blood clots in the lungs. Some analyze mammograms and ultrasound images for signs of breast cancer, while others examine brain scans for signs of hemorrhage. Cardiac AI devices can now flag a wide range of hidden heart problems. But how much do either regulators or doctors really know about the accuracy of these tools? A new study led by researchers at Stanford, some of whom are themselves developing devices, suggests that the evidence isn’t as comprehensive as it should be and may miss some of the peculiar challenges posed by artificial intelligence.
Quite surprisingly, a lot of the AI algorithms weren’t evaluated very thoroughly
James Zou
Many devices were tested solely on historical — and potentially outdated — patient data. Few were tested in actual clinical settings, in which doctors were comparing their own assessments with the AI-generated recommendations. And many devices were tested at only one or two sites, which can limit the racial and demographic diversity of patients and create unintended biases. "Quite surprisingly, a lot of the AI algorithms weren’t evaluated very thoroughly," says James Zou, the study’s co-author, who is an assistant professor of biomedical data science at Stanford University as well as a faculty member of the Stanford Institute for Human-Centered Artificial Intelligence (HAI).
In the study, the Stanford researchers analyzed the evidence submitted for every AI medical device that the FDA approved from 2015 through 2020. In addition to Zou, the study was conducted by Eric Wu and Kevin Wu, PhD candidates at Stanford; Roxana Daneshjou, a clinical scholar in dermatology and a postdoctoral fellow in biomedical data science; David Ouyang, a cardiologist at Cedars-Sinai Hospital in Los Angeles; and Daniel E. Ho, a professor of law at Stanford as well as associate director of Stanford HAI.
Recommended article
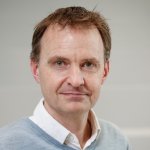
Article • Finding solutions for radiology tasks
Putting AI algorithms under the microscope
With a growing number of Artificial Intelligence (AI) algorithms to support medical imaging analysis, finding the best solution for specific radiology tasks is not always straightforward. Many are cloaked in secrecy and commercial sensitivity and while an algorithm may perform well in one area, evidence of performance and adaptability in another environment is not always available.
In sharp contrast to the extensive clinical trials required for new pharmaceuticals, the researchers found, most of the AI-based medical devices were tested against “retrospective” data — meaning that their predictions and recommendations weren’t tested on how well they assessed live patients in real situations but rather on how they might have performed if they had been used in historical cases. One big problem with that approach, says Zou, is that it fails to capture how health care providers use the AI information in actual clinical practice. Predictive algorithms are primarily intended to be a tool to assist doctors — and not to substitute for their judgment. But their effectiveness depends heavily on the ways in which doctors actually use them.
The researchers also found that many of the new AI devices were tested in only one or two geographic locations, which can severely limit how well they work in different demographic groups. “It’s a well-known challenge for artificial intelligence that an algorithm may work well for one population group and not for another,” says Zou.
The researchers offered concrete evidence of that risk by conducting a case study of a deep learning model that analyzes chest X-rays for signs of collapsed lungs. The system was trained and tested on patient data from Stanford Health Center, but Zou and his colleagues tested it against patient data from two other sites — the National Institute of Health in Bethesda, Md., and Beth Israel Deaconess Medical Center in Boston. Sure enough, the algorithms were almost 10 percent less accurate at the other sites. In Boston, moreover, they found that their accuracy was higher for white patients than for Black patients.
AI systems have been famously vulnerable to built-in racial and gender biases, Zou notes. Facial- and voice-recognition systems, for example, have been found to be much more accurate for white people than people of color. Those biases can actually become worse if they aren’t identified and corrected.
Recommended article
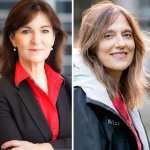
Article • AI does not discriminate
Removing bias from mammography screening with deep learning
AI can help tackle inequities and bias in healthcare but it also brings partiality issues of its own, experts explained in a Hot Topic session entitled "Artificial Intelligence and Implications for Health Equity: Will AI Improve Equity or Increase Disparities?" at RSNA.
Zou says AI poses other novel challenges that don’t come up with conventional medical devices. For one thing, the datasets on which AI algorithms are trained can easily become outdated. The health characteristics of Americans may be quite different after the Covid-19 pandemic, for example.
We don’t want things to be overregulated. At the same time, we want to make sure there is rigorous evaluation especially for high-risk medical applications
James Zou
Perhaps more startling, AI systems often evolve on their own as they incorporate additional experience into their algorithms. “The biggest difference between AI and traditional medical devices is that these are learning algorithms, and they keep learning,” Zou says. “They’re also prone to biases. If we don’t rigorously monitor these devices, the biases could get worse. The patient population could also evolve.”
“We’re extremely excited about the overall promise of AI in medicine,” Zou adds. Indeed, his research group is developing AI medical algorithms of its own. “We don’t want things to be overregulated. At the same time, we want to make sure there is rigorous evaluation especially for high-risk medical applications. You want to make sure the drugs you are taking are thoroughly vetted. It’s the same thing here.”
Source: Stanford University
21.04.2021