Image source: Adobe Stock/Alexander Limbach
Article • Technology overview and outlook
AI in healthcare: Significant potential – and serious obstacles
Today, artificial intelligence (AI) is everywhere. The alarm clock that wakes us up in the morning was set by a voice assistant, we unlock our smartphone with face recognition, we watch movies recommended by AI. And the pleasant voice that reads the weather report – and the weather report itself – are generated by AI. While AI is deeply integrated into our lives, we are still waiting for it to bring us that special smoothie in the morning or discuss the book with us that’s sitting on our bedside table. Sure, AI still has a long way go. But maybe one day in the not-so-distant future, AI will provide us with information about our current state of health, such as the number of red blood cells, cholesterol levels, fat percentage, and how many seconds last night's beer will shorten our life expectancy.
By Valentina Endovitskaya and Anton Dolgikh
Unfortunately (or fortunately, depending on your point of view), the human body is such a complex organism that predicting health indicators is much more complicated than retrieving a song or a weather report. I might be happy with Spotify, but most of the diagnostic tools currently available are very disappointing. This is a global phenomenon: in healthcare, AI-based solutions are hitting serious obstacles. There is a huge gap between science and business, there are legal restrictions and a lack of labeled and publicly available data. And we haven’t even talked about user distrust yet.
The world urgently needs intelligent solutions to the problems of modern medicine. Covid-19 has shown that the adoption of machine learning (ML) needs to be accelerated. With the help of AI, many amazing things can be done or created in the near future – medicines, vaccines, and improved clinical services. AI can be used to develop diagnostic tools, expand telemedicine, improve the accuracy of diagnoses, and even overcome logistical challenges. However, these valuable solutions are not yet available to a wider audience.
Recommended article
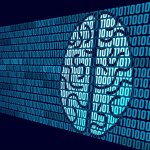
Article • Technology overview
Artificial intelligence (AI) in healthcare
With the help of artificial intelligence, computers are to simulate human thought processes. Machine learning is intended to support almost all medical specialties. But what is going on inside an AI algorithm, what are its decisions based on? Can you even entrust a medical diagnosis to a machine? Clarifying these questions remains a central aspect of AI research and development.
Why do we need AI in healthcare?
Here are some ways AI can bring benefits:
- It can accelerate processes such as drug development, assign patients to trials, process documents, make appointments, automate planning, and optimize schedules. ML models can be trained to recognize mentions of drugs and diseases, find optimal strategies, and identify specific patterns or images.
- AI can improve the decision quality to help with diagnoses and treatments, patient monitoring, and predictions. One of the goals of AI is to provide clinical guidelines and analyze patient outcomes and apply the knowledge learned by producing accurate results.
- AI can alleviate resource scarcity by using symptom checkers and telemedicine.
- AI can reduce human error by detecting unusual medication dosages, prescriptions, or suspicious analysis results.
- AI can help us respond quickly to changing situations by detecting adverse reactions via social media posts and opinion polls. It's crucial to be up to date. The use of social media and various data sources allows healthcare companies to adapt to a new reality easily and quickly.
- AI can trigger innovations and discoveries that might offer more precise diagnoses, gene editing, and even longer life expectancy. By leveraging real-world data sources (RWD), AI can provide new insights, dependencies, and relationships.
Why AI isn't here yet
How can it be that a powerful tool like AI is not already being used in clinical settings? Why don't robots diagnose diseases and suggest treatments? The answer in a nutshell: while there are improvements, there are still obstacles on the way to clinical use. These challenges are not always of a technical nature:
- Cultural and political restrictions. Although its benefits have been proven, AI is still unregulated and no ethical standards have been developed for its use. Many questions about privacy and accountability for AI decisions remain open, thus there are many concerns that are holding back its use in healthcare.
- A huge gap between business and science. Scientists and developers can't simply decide which problems the healthcare industry needs to solve; at the same time, medical staff and management typically don't fully understand ML capabilities. Therefore, any successful team needs to bring together the worlds of science and business.
- Distrust of users. Since most AI models are considered “black boxes”, unlike other software, their internal logic is difficult to understand. Therefore, users consider the results unreliable. Customers need to understand how a model makes decisions, but high-precision models are usually difficult to interpret. To overcome this problem, the General Data Protection Regulation requires the algorithms that manipulate patient data to be explainable. But what exactly does “explainable” mean?
Recommended article
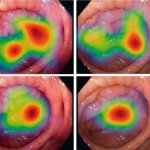
Article • Diagnostic assistant systems
AI in endoscopy: helper, trainer – influencer?
Artificial intelligence (AI) is increasing its foothold in endoscopy. Although the algorithms often detect pathologies faster than humans, their use also generates new problems. PD Dr Alexander Hann from the University Hospital Würzburg points out that the use of AI helpers can affect not only the reporting of findings – but also the person making the findings.
- Lack of publicly available data. The development of AI is driven by data, and the role of data is critical to training models. The industry keeps its data in silos and guards it like treasures. But it's hard, if not impossible, to imagine a productive interaction between data science and business without data sharing.
- Lack of experience in management. While data plays an important role in the development of AI, without competent management and well-established workflows its potential cannot be fully realized.
- Lack of standards. Collecting data from various sources and processing it takes time. A lot of time. With specifications missing, a wide range of tailor-made solutions are created, which makes optimal use of the data difficult. In order to normalize and standardize data and facilitate data exchange, various ontologies have been developed. They cover almost all areas of medicine and science, including SNOMED, NCIT, and AniML.
The way forward
The future of AI/ML is complex and requires effort and support from all stakeholders: scientists, developers, companies and government agencies. Unfortunately, these parties do not always cooperate effectively. It is not possible to transfer the ideas and models developed by scientists/data scientists to the world of users without bias. There is a barrier between scientists and users and they each speak their languages that can be incomprehensible to the other. What is needed is a kind of interpreter who transforms developed models and ideas into sustainable solutions that bring benefits to a company. This is a complex process that involves many experts – developers, designers, business analysts, and others.
While science and business have already been brought together by software development specialists, regulation is still in the making. Who should be held liable for AI decisions and errors? How do we protect personal data used by smart applications? Are there ways to eliminate discrimination and prejudice? Only when these and many other questions have been answered can companies develop solutions that meet legal, ethical and social requirements.
Recommended article
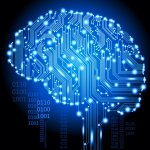
News • WHO global report
The six guiding principles for AI in healthcare
Artificial Intelligence (AI) holds great promise for improving the delivery of healthcare and medicine worldwide, but only if ethics and human rights are put at the heart of its design, deployment, and use, according to new WHO guidance.
The future
By 2030, the artificial intelligence market in healthcare is expected to reach a value of $208.2 billion. The prediction and prevention of pandemics, the in-depth analysis of patients and constant monitoring to control and prevent possible health problems will be within reach, as will the use of VR in the training of medical staff, especially surgeons. But at the same time, problems are also inevitable.
Take home message
No matter how slow and rocky the path to AI adoption in healthcare is, AI still offers a way to integrate science into business. We see the future approaching, step by step. With steady and constant efforts from teams around the globe, AI will make its way into every area of healthcare. There are still many challenges ahead, but the future of AI in healthcare is promising.
Literature upon request by the authors
06.11.2023