Article • AI does not discriminate
Removing bias from mammography screening with deep learning
AI can help tackle inequities and bias in healthcare but it also brings partiality issues of its own, experts explained in a Hot Topic session entitled "Artificial Intelligence and Implications for Health Equity: Will AI Improve Equity or Increase Disparities?" at RSNA. Two leading researchers showcased how deep learning may help bring back all the patients into screening mammography after the pandemic, by reducing risk of bias based on race or other factors.
Report: Mélisande Rouger
An imaging-only model for breast cancer prediction
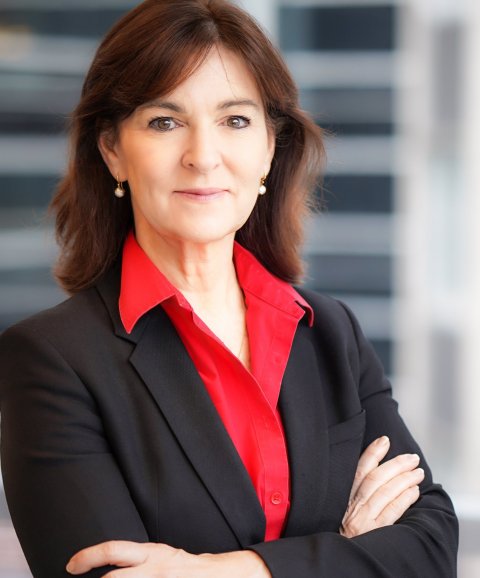
Constance Lehman, a professor of radiology at Harvard Medical School, presented an algorithm she developed with Regina Barzilay, a professor of computer science and electrical engineering at the MIT Institute of Medical Engineering & Science, to help identify women's risk for breast cancer (BC) based on their mammogram alone.
The two teamed up in 2016, to evaluate the power of AI to predict a woman’s future risk of developing breast cancer, as they collected evidence that AI can help improve equity in healthcare. "It is a myth that AI would introduce bias or worsen healthcare disparities, we saw ample evidence in our research that AI could reduce disparities," Lehman said.
The researchers also understood that they could do more with deep learning (DL) than just predict the future development of cancer or not, and look at a mammogram using DL to predict a woman’s risk factors. “About 2M women will be diagnosed with BC and over 600k will die in the US this year, according to the National Cancer Institute. But there’s a marked discrepancy in the impact of BC on women of color vs. Caucasian women, which many refer to this as ‘the perfect storm’,” she explained.
Lehman and Barzilay developed a DL model using an imaging coder that takes the four views of a standard digital analog mammogram and puts it through an image aggregator to combine information across the views. Those rich aggregated mammograms are then used to predict a woman’s risk factors and can also calculate whether the woman can develop cancer in one year or more. “The model does not require access to family history, prior biopsies or reproductive history, but only uses only the mammogram. This imaging only approach performs better than other models and supports equity across the races,” Lehman said.
Fighting the impact of Covid-19
The backlog is significant. Over 100,000 screening mammograms were turned off on a daily basis and we’re working really hard to get these women back in
Constance Lehman
Thousands of mammograms were canceled worldwide due to the pandemic and providers looked at new ways to do screening. Lehman has used AI-boosted risk-based screening at her institutions, to determine which women to bring back in for screening first. “With covid-19, for the first time in our history of screening, screening mammograms were put on hold. This may continue. (…) The backlog is significant. Over 100,000 screening mammograms were turned off on a daily basis and we’re working really hard to get these women back in,” she said.
Lehman is concerned that social determinants are influencing which patient is going back to screening, and she has already noticed a dramatic decrease of women of color among the population returning to screening mammography. AI could help get all the patients back into screening. “If we focus on risk based screening using DL models we could avoid the disparities that have been so inherent to traditional risk models, and we can really change this direction we’re going in of increased morbidity and mortality from breast cancer due to covid-19,” she said.
Building robust AI to support equity
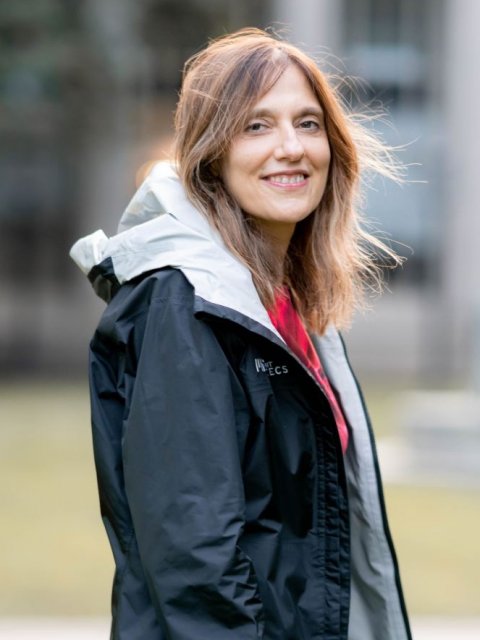
Some phenomena such as nuisance variation have been showed to contribute to bias of AI clinical systems. But there are strategies to fight that problem and the first is to make sure that the data is right, diverse and representative of the whole population, according to Barzilay. “An image based model that is trained on diverse population can very accurately predict risk across different populations in a very consistent way,” she said. Barzilay herself was diagnosed with breast cancer in her 40s, which made her a minority. "It taught me how important it is to create systems that are fair for everyone," she said.
However, testing the model on different demographics is not standard today, so the community should try to make sure to make sure that models are trained across different populations.
A second way to go is to use an algorithmic solution and the AI community is working hard on tools that can work robustly against bias, by making sure that models are trained to be robust in the presence of bias, which may come from the nuisance variation between devices used to take the imaging.
Barzilay and Lehman’s algorithm “enables to encode the image in such a way that even though the images come from different devices, internally the machine treats it in the same way and it cares to distinguish them based on malignancy rather than distinguish them from the source where images were taken,” she said. Humans who are ultimately responsible for making a clinical decision should understand what the machine is doing, to think of all possible biases that the machine can introduce, Barzilay added. “Models that can make their reasoning understandable to humans could help”, she said.
The session also featured talks by Ziad Obermeyer, MD, associate professor of health policy and management at the Berkeley School of Public Health, CA, and Luke Oakden-Rayner, MD, PhD, director of medical imaging research at the Royal Adelaide Hospital in Australia. The discussion was moderated by Judy Wawira Gichoya, MBChB, MS, assistant professor in the Department of Radiology at Emory University School of Medicine, Atlanta.
Profiles:
Constance Lehman, MD, PhD is Professor of Radiology at Harvard Medical School, Director of Breast Imaging, and Co-Director of the Avon Comprehensive Breast Evaluation Center at Massachusetts General Hospital in Boston. She serves on several national committees including the National Cancer Institute’s Breast Cancer and the National Comprehensive Cancer Network steering committees. She has served as principal investigator or co-investigator on numerous single and multi-institution clinical trials in breast MRI, mammography and ultrasound and has authored or co-authored over 200 peer-reviewed scientific publications.
Regina Barzilay is Professor in the Department of Electrical Engineering and Computer Science and a member of the Computer Science and Artificial Intelligence Laboratory at the Massachusetts Institute of Technology. Her research interests are in natural language processing, drug discovery and clinical AI. In collaboration with physicians and her students, she is devising deep learning models that utilize imaging, free text, and structured data to identify trends that affect early diagnosis, treatment, and disease prevention in oncology.
22.12.2020