Article • Finding solutions for radiology tasks
Putting AI algorithms under the microscope
With a growing number of Artificial Intelligence (AI) algorithms to support medical imaging analysis, finding the best solution for specific radiology tasks is not always straightforward. Many are cloaked in secrecy and commercial sensitivity and while an algorithm may perform well in one area, evidence of performance and adaptability in another environment is not always available.
Report: Mark Nicholls
Image source: Unsplash/Walkator
One approach to tackle these uncertainties and deliver a fair comparison of algorithms on the same data are AI challenges – or competitions – which act as a type of testbed for AI algorithms and scrutinize their adaptability across different environments and scenarios.
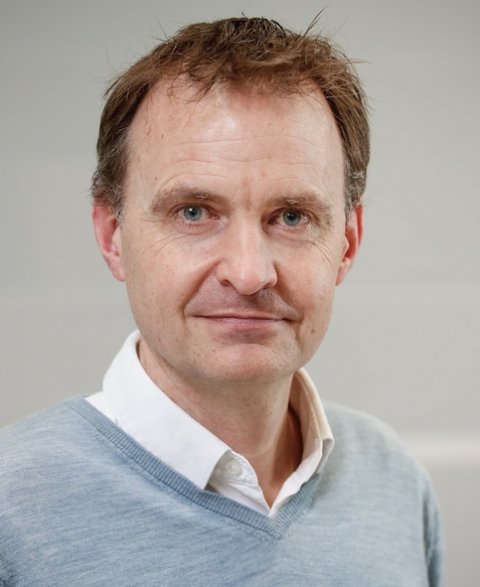
Professor Bram van Ginneken - who has organised many of these challenge events - will highlight this approach in his ECR 2021 presentation “Benefits of AI challenges to clinical practice.” In it, he will discuss AI challenges and their organisation, offer an understanding of what happens with the data collected, and point out algorithms which won AI challenges and made it to the clinic. In addition, the work Professor van Ginneken and his associates are doing in this field is also enabling the development of other algorithms, often in very specialist areas that would not normally attract the development interest of major vendors.
Speaking ahead of the ECR virtual session, he said the challenge events create an opportunity to compare algorithms for particular tasks. With some 150 products for AI in radiology with CE certification for use in Europe, commercial sensitivity among vendors means the content of some algorithms remains unclear, said Professor van Ginneken, who is Professor of Medical Image Analysis at Radboud University Medical Center in The Netherlands. Van Ginneken’s group created a platform – grand-challenge.org – where challenges and the algorithms are hosted. Some are so effective that they have made it to market. He emphasised that the competitions are primarily aimed at the research community, and make datasets available for researchers who want to work on medical data.
Recommended article
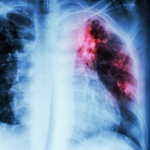
Article • The impact of AI
Radiology and radiologists: a painful divorce
AI-based applications will replace radiologists in some areas, the physicist Bram van Ginneken predicts. ‘The profession of radiologist will change profoundly,’ predicts Gram van Ginneken, Professor of Medical Image Analysis at Radboud University Medical Centre. The cause is automatic image analysis by computers (first published in a paper in 1963) and deep learning.
A key aspect lies in comparing the algorithms on the same test data with like-with-like comparisons and checking how an algorithm that has been tested on a dataset for one scenario such as in a hospital, for example, works in different populations. While the quality control aspect of Professor van Ginneken’s work is important, he also underlines the value of making high-quality training datasets available that can lead to other algorithms being developed.
Some of the challenges involve working with vendors, though not all are comfortable with participating because of the risk of an algorithm not performing well when tested in different contexts. But algorithms that do come out of these competitions can be licensed to companies and support product development. Examples Professor van Ginneken will discuss include an algorithm developed for the CO-RADS scoring system in the first wave of the coronavirus pandemic in May, which under the rapid certification scenario surrounding the pandemic, was on the market by June. Another example is the outcome from a 2017 competition to predict the likelihood of patients developing lung cancer within a year from a CT scan of the lungs. A liver segmentation algorithm will also be highlighted.
While AI development looks like a big breakthrough, there are still lots of areas where there is no software solution
Bram van Ginneken
The challenges mean researchers can compare different approaches on the same data set, and they also address the broader issue of scalability for AI in radiology. Professor van Ginneken says despite the range of AI products on the market, AI only covers a small element of radiology work and developing products for rare cases and disease has limited commercial appeal to major companies. “There are rare tasks for which we also need solutions and I think these challenges/competitions can provide that,” he said. “This is where there will be a role for algorithms that are developed in the research community.”
Sharing data remains an issue and can be hampered by privacy considerations and getting permission to use datasets for research. “While AI development looks like a big breakthrough, there are still lots of areas where there is no software solution,” he said, “so these challenges are one element that can really help to broaden the scope, the repertoire of tools that radiologists can use.”
The session will also look at presentations exploring the value of AI in chest x-ray prioritisation, and discuss the French DRIM AI study.
Profile:
Bram van Ginneken is Professor of Medical Image Analysis at Radboud University Medical Center and chairs the Diagnostic Image Analysis Group. He is also a founder of Thirona, a company that develops software and provides services for medical image analysis. A member of the Editorial Board of Medical Image Analysis, he pioneered the concept of challenges in medical image analysis.
05.03.2021