News • Deep learning applied to MRI scans
Glioblastoma: Using AI to improve prognosis and treatment
In the first study of its kind in cancer, researchers have applied artificial intelligence to measure the amount of muscle in patients with brain tumours to help improve prognosis and treatment.
Dr Ella Mi, a clinical research fellow at Imperial College London (UK) will tell the NCRI (National Cancer Research Institute) Virtual Showcase, that using deep learning to evaluate MRI brain scans of a muscle in the head was as accurate and reliable as a trained person, and was considerably quicker. Furthermore, her research showed that the amount of muscle measured in this way could be used to predict how long a patient might survive their disease as it was an indicator of a patient’s overall condition.
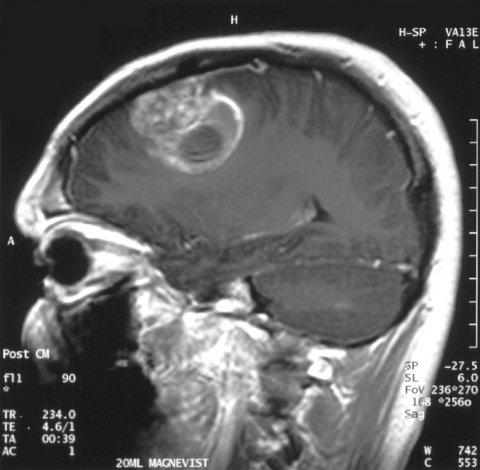
Image source: Christaras A, Glioblastoma - MR sagittal with contrast, CC BY-SA 3.0
Glioblastoma is an aggressive brain tumour that is very difficult to treat successfully. Average survival after diagnosis is 12-18 months and fewer than 5% of patients are still alive after five years. Some patients do better than others, and so the ability to assess objectively patients’ frailty and physical condition provides important information that can improve prognosis and help guide decisions on treatments, diet and exercise. If patients have sarcopenia – degenerative loss of skeletal muscle – they may be unable to tolerate surgery, chemotherapy or radiotherapy as well as patients without the condition. This can lead to adverse reactions to therapy, early discontinuation of treatment, accelerated progression of the disease and death.
Dr Mi said: “Finding a better way to assess patients’ physical condition, general well-being and ability to carry out everyday activities is important in glioblastoma, and indeed in many cancers, because, at present, it’s often evaluated subjectively, resulting in inaccuracy and a high degree of variability depending on who is looking at it. So indicators that can be assessed objectively, such as measures of sarcopenia, are needed.”
Recommended article
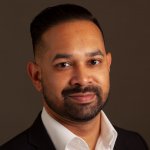
Article • Assessment of brain tumour treatment response
Developing AI algorithms for earlier glioblastoma detection
Novel advanced imaging biomarkers are being developed in a series of studies at several UK centres that may lead to the earlier assessment of treatment response to glioblastoma (GBM) and a better survival rate.
Dr Mi and her colleagues from the Computational Oncology Group at Imperial, led by Dr Matthew Williams, looked at 152 MRI brain scans belonging to 45 patients from two different data sources (The Imperial Tissue Bank and the Diffusion in Glioma Study) who were diagnosed with glioblastoma between January 2015 and May 2018. The scans, which were taken as part of routine clinical care during diagnosis and follow-up of the patients, looked at cross-sections of the head. Dr Mi and colleagues focused on the temporalis muscle – broad, fan-shaped muscles on either side of the head that are used for chewing food – which has previously been identified as a good way to estimate skeletal muscle mass in the body. “We realised that sarcopenia could be identified by quantifying muscle in cross-sectional imaging that cancer patients routinely undergo. This would allow for opportunistic screening of sarcopenia as part of cancer care without additional scanning time, radiation dose or cost,” said Dr Mi.
The researchers trained a convolutional neural network – a type of deep learning or artificial intelligence model – to analyse the scans, and to identify and quantify the cross-sectional area (CSA) of the temporalis muscle at its thickest part. Then they looked at the association of CSA with overall survival and how long patients lived without their disease progressing (progression-free survival). They found that CSA was a significant predictor of overall and progression-free survival. Patients with a high CSA, indicating more muscle, had half the risk of dying and a two-thirds reduction in risk of the disease progressing at any given time compared to patients with a low CSA, indicating a reduced amount of muscle. The effect of CSA on overall and progression-free survival was particularly strong in patients under the age of 55 years and men.
We are the first to show that this measurement of sarcopenia, generated automatically from routine imaging is sufficiently accurate and reliable to be a useful prognostic marker in cancer, while taking substantially less time than trained humans
Ella Mi
Dr Mi said: “The average overall survival for patients in the high CSA group was 21.3 months compared to 14 months for patients in the low CSA group. Recently, we have taken the work forward to take into account important factors such as age, sex, which side of the brain the tumour was on and a genetic characteristic of brain tumours that often predicts response to chemotherapy. We have found that patients with high CSA had around 60% reduction in risk of death and 75% reduction in risk of disease progression compared to patients with low CSA, even when all these other factors are accounted for. To our knowledge, this is the first study, in any cancer, to apply deep learning to muscle segmentation and quantification for sarcopenia assessment, and to demonstrate significant associations with clinical outcomes. We are the first to show that this measurement of sarcopenia, generated automatically from routine imaging is sufficiently accurate and reliable to be a useful prognostic marker in cancer, while taking substantially less time than trained humans.
“We show that higher temporalis muscle area before surgery, chemotherapy or radiotherapy is predictive of significantly longer overall and progression-free survival. This has the potential to improve prognostic estimates and could be used to plan treatments. For instance, previous evidence has shown that frail patients might benefit from shorter courses of radiotherapy, or chemotherapy with temozolomide alone. It could also guide therapeutic interventions for muscle preservation, including nutritional support, exercise therapy and drugs.”
Dr Mi and her colleagues are now validating their results in another, different set of patients, as well as carrying out further studies to characterise changes in the temporalis muscle during follow-up of glioblastoma patients after diagnosis and treatment. They hope to collaborate with other academic centres that have their own repositories of glioblastoma brain MRI scans in order to set up a multi-centre study.
Professor David Harrison, who is Professor of Pathology at the University of St Andrews and chair of the NCRI Cellular Molecular Pathology Initiative, was not involved with the research. He commented: “Doctors who treat cancer patients know that a person’s physical condition and frailty is linked to their ability to tolerate treatment. It also affects how they respond and their long-term survival. However, it has been very difficult to develop objective measurements of patients’ performance status. This is where AI can help. The research by Ella Mi and her colleagues applies AI in a cancer which is particularly aggressive and difficult to treat successfully in order to select the best treatment approach. At this stage their findings show that there is an association between the temporalis muscle size and a patient’s frailty and how their disease progresses. The results do not show that the muscle area actually causes the change in patients’ outcomes. The findings deserve study in a larger group of patients to take account of factors such as changes to the muscle caused by other factors, including surgery or radiotherapy.”
Source: National Cancer Research Institute (NCRI)
29.10.2020