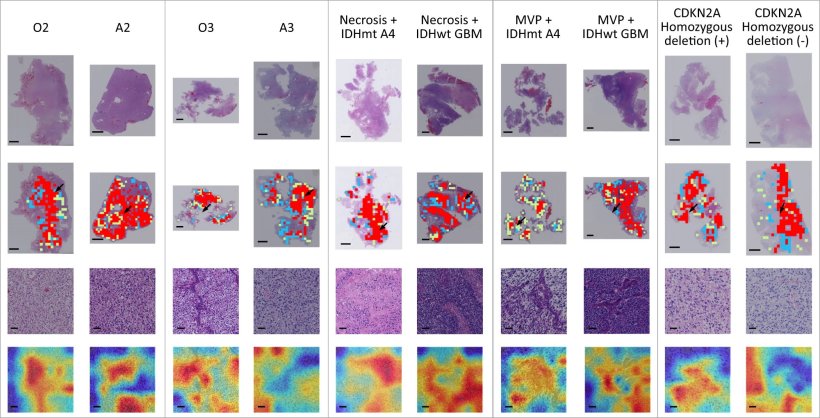
Image source: Wang W et al., Nature Communications 2023 (CC BY 4.0)
News • WSI-based analysis
AI-driven classification of diffuse gliomas skips molecular testing
Diffuse gliomas, which account for the majority of malignant brain tumors in adults, comprise astrocytoma, oligodendroglioma, and glioblastoma. Current diagnosis of glioma types requires combining both histological features and molecular characteristics.
This poses unique challenges in developing an integrated diagnosis model directly from whole-slide images (WSIs) to classify different types of adult-type diffuse gliomas by analyzing WSIs. Additionally, the gigapixel-level resolution of WSI makes original Convolutional Neural Network computationally impossible.
Recently, a researcher team led by Prof. Zhicheng Li from the Shenzhen Institute of Advanced Technology (SIAT) of Chinese Academy Sciences has proposed an integrated diagnosis model for automatic classification of adult-type diffuse gliomas directly from annotation-free standard whole-slide pathological images without requiring molecular test. The model can classify tumors strictly according to the fifth edition of the World Health Organization (WHO) Classification of Tumors of the Central Nervous System (CNS) released in 2021.
Our integrated diagnosis model has the potential to be used in clinical scenarios for automated and unbiased classification of adult-type diffuse gliomas
Zhicheng Li
The study involved the creation of a deep learning model that analyzes WSIs, enabling it to identify and classify gliomas without extensive manual annotation. The model was trained and validated on a dataset of 2624 patient cases from three different hospitals, ensuring a diverse and comprehensive dataset. The effectiveness of the model was measured by its accuracy in classification, sensitivity to different glioma types and grades, and its ability to distinguish between genotypes with similar histological features.
Experimental results showed that the proposed model achieves high performance with area under receiver operator curve all above 0.90 in classifying major tumor types, in identifying tumor grades within type, and especially in distinguishing tumor genotypes with shared histological features. "Our integrated diagnosis model has the potential to be used in clinical scenarios for automated and unbiased classification of adult-type diffuse gliomas," said Prof. Li. "Our future research could focus on expanding this model to include multi-center, multi-racial datasets and further integration of these technologies into everyday medical practice."
Source: Shenzhen Institute of Advanced Technology, Chinese Academy of Sciences
28.11.2023