Article • Assessment of brain tumour treatment response
Developing AI algorithms for earlier glioblastoma detection
Novel advanced imaging biomarkers are being developed in a series of studies at several UK centres that may lead to the earlier assessment of treatment response to glioblastoma (GBM) and a better survival rate.
Report: Mark Nicholls
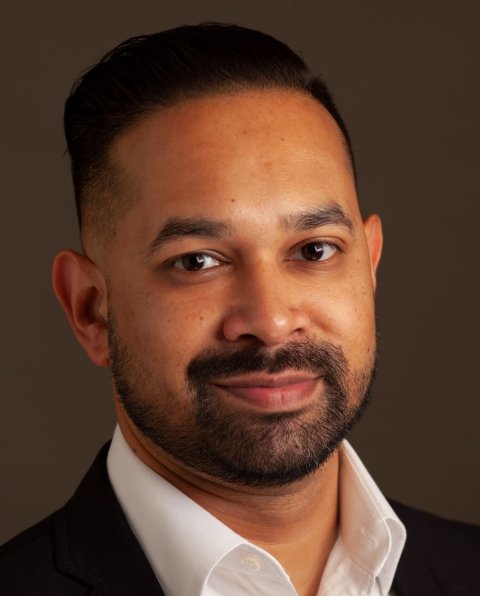
Through a number of clinical trials – and the application of artificial intelligence (AI) to retrospective data sets – the aim is to highlight approaches that will enable clinicians to recognise much sooner when treatments are failing and thus switch patients to a second therapy before too late.
Neuroradiologist Shah Islam, a clinical research fellow at Imperial college London (ICL), who is completing a PhD in advanced computational brain imaging, has become a key figure in the research to develop the robust imaging biomarkers needed to detect early treatment response in glioblastoma cases. ‘The aim is to see whether, by using the novel MRI sequence, we can predict which patients will respond to treatment at the mid-radiotherapy stage,’ he said.
Five centres and three time points
Among the trials is the Diffusion in Glioma Study (DIG), in association with the Brain Tumour Charity. This involves five UK neuroscience centres – Charing Cross Hospital, London; Addenbrooke’s Hospital, Cambridge; The Royal Infirmary, Edinburgh; the Walton Centre, Liverpool, and the National Hospital for Neurology and Neurosurgery at Queen Square, London – where advanced Diffusion Weighted Imaging (DWI) MRI sequences are performed at three time points: pre-, mid- and post-radiotherapy.
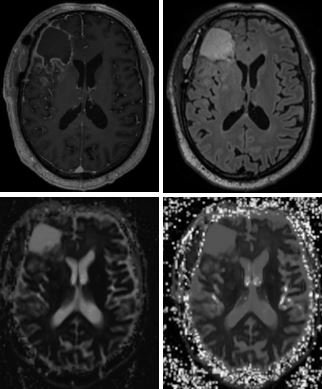
With current imaging reliance on T1 contrast enhancement to look for disease, the researchers are unsure whether this is sensitive enough to appreciate subtle changes in the underlying tumour. This has seen use of advanced DWI to extrapolate the most sensitive part of diffusion imaging that can help researchers to accurately identify changes in the tumour microenvironment. ‘What is important about the DIG study is that it is multi-centre and uses scanners from multiple manufacturers, making any positive results more robust,’ Islam pointed out.
A second study – the 18F FPIA PET MRI in Glioma, funded by the Medical Research Council – uses a new radio tracer developed at Imperial College London combined with hybrid PET/MRI scanning. ‘The radio tracer has been developed to specifically detect tumour cells in the brain, by looking for fatty acid metabolism, which healthy brain cells do not exhibit,’ Islam explained. ‘The idea is that we can look for any residual disease following the surgical resection, and also explore its use as treatment response marker following radiotherapy.’ As 18F FPIA is designed to detect only tumour cells, the healthy brain should not demonstrate any signal, he added.
Islam is also on the steering committee of the Tessa Jowell* Brain Matrix (TJBM) – a 10-centre government-led study aimed at improving outcomes in patients with aggressive brain tumours – which endeavours to curate all GBM data prospectively, including imaging data, histological and molecular data, and genetic data, to allow the successful development of an AI approach.
Earlier detection of treatment response
There is a small window to change people to second-line therapies; if we can identify those that won’t do well on first-line treatment, we can then change them to another treatment
Shah Islam
‘The lifeblood of any AI in imaging,’ Islam continued, ‘is the data used to create algorithms. With the TJBM, we have the volume of data coming through, which is labelled perfectly and combined with patient metadata. This will allow us to develop AI algorithms that can detect treatment response earlier.’
A current problem when patients go on first-line treatment for GBM – surgery, radiotherapy and adjuvant temozolomide (chemotherapy drug) – is that clinicians do not know which patients will do well and those that won’t until it is too late. ‘There is a small window to change people to second-line therapies; if we can identify those that won’t do well on first-line treatment, we can then change them to another treatment,’ he said. ‘The average life expectancy is approximately 18 months and we haven’t seen a positive trial result since the introduction of temozolomide 15 years ago; so we are failing these patients. We hope a benefit will be to allow new drugs to be tested in clinical trials using the new biomarkers to appropriately validate the new drug.’
Preliminary results of the trials were presented at RSNA 19, but Islam confirmed that, although they ‘demonstrate early promise’, they are still a long way from clinical translation into routine imaging.
Profile:
Neuroradiologist Dr Shah Islam, who runs the DIG and FPIA studies, is a clinical research fellow at Imperial College London. Having graduated from Barts and The London School of Medicine and Dentistry, he underwent combined clinical academic training at Barts and was an NIHR academic clinical fellow in Radiology at The Royal Marsden NHS Foundation Trust. His research focuses on developing novel imaging biomarkers to detect early treatment response in glioblastoma using advanced imaging techniques achieved through a number of clinical trials and advanced computational methods involving AI.
* Baroness Tessa Jowell was a UK politician who died of GBM and worked to raise awareness of the condition.
12.03.2020