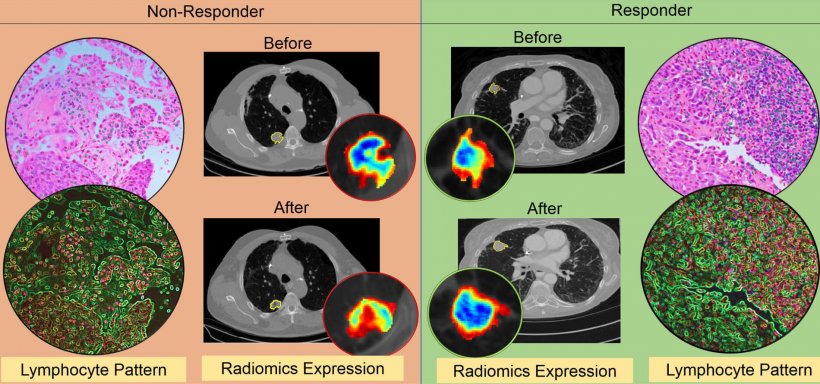
Credit: Case Western Reserve University
News • Personalized diagnostics
AI checks effectiveness of immunotherapy
Scientists from the Case Western Reserve University digital imaging lab use Artificial Intelligence (AI) to predict which lung-cancer patients will benefit from expensive immunotherapy.
This is done by teaching a computer to find previously unseen changes in patterns in CT scans taken when the lung cancer is first diagnosed compared to scans taken after the first 2-3 cycles of immunotherapy treatment. As with previous work from the researchers, those changes have been discovered both inside and outside the tumor, a signature of the lab's recent research. "This research really seems to be reflecting something about the very biology of the disease, about which is the more aggressive phenotype, and that's information oncologists do not currently have," said Anant Madabhushi from the Center for Computational Imaging and Personalized Diagnostics (CCIPD).
The new research, led by co-authors Mohammadhadi Khorrami and Prateek Prasanna, along with Madabhushi and 10 other collaborators from six different institutions was published this month in the journal Cancer Immunology Research.
Even though immunotherapy has changed the entire ecosystem of cancer, it also remains extremely expensive—about $200,000 per patient, per year
Anant Madabhushi
Currently, only about 20% of all cancer patients will actually benefit from immunotherapy, a treatment that differs from chemotherapy in that it uses drugs to help your immune system fight cancer, while chemotherapy uses drugs to directly kill cancer cells, according to the National Cancer Institute.
Madabhushi said the recent work by his lab would help oncologists know which patients would actually benefit from the therapy, and who would not. "Even though immunotherapy has changed the entire ecosystem of cancer, it also remains extremely expensive—about $200,000 per patient, per year," Madabhushi said. "That's part of the financial toxicity that comes along with cancer and results in about 42% of all new diagnosed cancer patients losing their life savings within a year of diagnosis."
Having a tool based on the research being done now by his lab would go a long way toward "doing a better job of matching up which patients will respond to immunotherapy instead of throwing $800,000 down the drain," he added, referencing the four patients out of five who will not benefit, multiplied by annual estimated cost.
Khorrami, a graduate student working at the CCIPD, said one of the more significant advances in the research was the ability of the computer program to note the changes in texture, volume and shape of a given lesion, not just its size. "This is important because when a doctor decides based on CT images alone whether a patient has responded to therapy, it is often based on the size of the lesion," Khorrami said. "We have found that textural change is a better predictor of whether the therapy is working. Sometimes, for example, the nodule may appear larger after therapy because of another reason, say a broken vessel inside the tumor—but the therapy is actually working. Now, we have a way of knowing that."
Recommended article
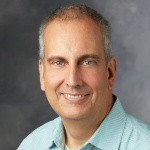
Article • New therapies, new questions
To understand an image is to understand the treatment
Conventional chemotherapy in oncology is increasingly yielding to new procedures such as targeted therapy and immunotherapy. This results in changes for radiologists because new procedures require familiarisation with certain imaging to ensure that the treatment process is interpreted correctly.
Prasanna, a postdoctoral research associate in Madabhushi's lab, said the study also showed that the results were consistent across scans of patients treated at two different sites and with three different types of immunotherapy agents. "This is a demonstration of the fundamental value of the program, that our machine-learning model could predict response in patients treated with different immune checkpoint inhibitors," he said. "We are dealing with a fundamental biological principal." Prasanna said the initial study used CT scans from 50 patients to train the computer and create a mathematical algorithm to identify the changes in the lesion. He said the next step will be to test the program on cases obtained from other sites and across different immunotherapy agents. This research recently won an ASCO 2019 Conquer Cancer Foundation Merit Award.
Additionally, Madabhushi said, researchers were able show that the patterns on the CT scans which were most associated with a positive response to treatment and with overall patient survival were also later found to be closely associated with the arrangement of immune cells on the original diagnostic biopsies of those patients. This suggests that those CT scans actually appear to capturing the immune response elicited by the tumors against the invasion of the cancer—and that the ones with the strongest immune response were showing the most significant textural change and most importantly, would best respond to the immunotherapy, he said.
Source: Case Western Reserve University
26.11.2019