© Alex_Traksel – stock.adobe.com
News • Research on large foundation models
Reducing bias in pathology AI
Advanced artificial intelligence (AI) systems have shown promise in revolutionalizing the field of pathology through transforming the detection, diagnosis, and treatment of disease; however, the underrepresentation of certain patient populations in pathology datasets used to develop AI models may limit the overall quality of their performance and widen health disparities.
A new study led by investigators from Mass General Brigham highlights that standard computational pathology systems perform differently depending on the demographic profiles associated with histology images, but that larger “foundation models” can help partly mitigate these disparities. Findings, published in Nature Medicine, emphasize the need for more diverse training datasets and demographic-stratified evaluations of AI systems to ensure all patient groups benefit equitably from their use.
“There has not been a comprehensive analysis of the performance of AI algorithms in pathology stratified across diverse patient demographics on independent test data,” said corresponding author Faisal Mahmood, PhD, of the Division of Computational Pathology in the Department of Pathology at Mass General Brigham. “This study, based on both publicly available datasets that are extensively used for AI research in pathology and internal Mass General Brigham cohorts, reveals marked performance differences for patients from different races, insurance types, and age groups. We showed that advanced deep learning models trained in a self-supervised manner known as ‘foundation models’ can reduce these differences in performance and enhance accuracy.”
Recommended article
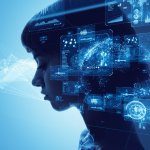
Article • Need for diversity in training datasets
Artificial intelligence in healthcare: not always fair
Machine learning and AI are playing an increasingly important role in medicine and healthcare, and not just since ChatGPT. This is especially true in data-intensive specialties such as radiology, pathology or intensive care. The quality of diagnostics and decision-making via AI, however, does not only depend on a sophisticated algorithm but – crucially – on the quality of the training data.
Based on data from the widely used Cancer Genome Atlas and EBRAINS brain tumor atlas, which predominantly include data from white patients, the researchers developed computational pathology models for breast cancer subtyping, lung cancer subtyping, and glioma IDH1 mutation prediction (an important factor in therapeutic response). When the researchers tested the accuracy of these models using histology slides from over 4,300 patients with cancer at Mass General Brigham and the Cancer Genome Atlas, and stratified the results by race, they found that the models performed more accurately in white patients than Black patients. The models the team tested for subtyping breast and lung cancers and predicting IDH1 mutation in glioma found respective disparities of 3.7%, 10.9%, and 16% in producing correct classifications.
Overall, the findings from this study represent a call to action for developing more equitable AI models in medicine
Faisal Mahmood
The researchers sought to reduce the observed disparities with standard machine learning methods for bias-mitigation, such as emphasizing examples from underrepresented groups during model training; however, these methods only marginally decreased the bias. Instead, disparities were reduced by using self-supervised foundation models, which are an emerging form of advanced AI trained on large datasets to perform a wide range of clinical tasks. These models encode richer representations of histology images that may reduce the likelihood of model bias.
Despite the observed improvements, gaps in performance were still evident, which reflects the need for further refinement of foundation models in pathology. Furthermore, the study was limited by small numbers of patients from some demographic groups. The researchers are pursuing ongoing investigations of how multi-modality foundation models, which incorporate multiple forms of data, such as genomics or electronic health records, may improve these models.
The emergence of AI tools in medicine has the potential to positively reshape the delivery of care. It is imperative to balance the innovative potential of AI with a commitment to quality and safety. Mass General Brigham is leading the way in responsible AI, conducting rigorous research on new and emerging technologies to inform the incorporation of AI in medicine.
“Overall, the findings from this study represent a call to action for developing more equitable AI models in medicine,” Mahmood said. “It is a call to action for scientists to use more diverse datasets in research, but also a call for regulatory and policy agencies to include demographic-stratified evaluations of these models in their assessment guidelines before approving and deploying them, to ensure that AI systems benefit all patient groups equitably.”
Source: Mass General Brigham
19.04.2024