Image source: Adobe Stock/metamorworks
Article • Need for diversity in training datasets
Artificial intelligence in healthcare: not always fair
Machine learning and artificial intelligence (AI) are playing an increasingly important role in medicine and healthcare, and not just since ChatGPT. This is especially true in data-intensive specialties such as radiology, pathology or intensive care. The quality of diagnostics and decision-making via AI, however, does not only depend on a sophisticated algorithm but – crucially – on the quality of the training data.
Article: Dr Christina Czeschik
Artificial intelligence is a buzzword. Underneath the buzz, AI consists of algorithms based on certain machine learning methods. One of these methods, which has received a lot of attention in recent years, is the artificial neural network. The layers of nerve cells that are involved in learning processes in the human brain are algorithmically reproduced (albeit idealized). Highly complex learning tasks require many layers of artificial neurons – this is deep learning, another AI term that has become popular.
A neural network and other forms of AI learn how to perform their task – such as making a specific diagnosis – based on training data. Imagine, the task is to distinguish malignant from benign findings on chest x-rays. In order for the system to be able to make a decision, many thousands or millions of x-rays of benign and malignant tissue changes are needed as training data. The algorithm classifies each of these training images and compares the result with the human-made diagnosis. If the algorithm’s diagnosis was incorrect, the weights assigned to individual connections between the virtual neurons are reweighted to improve accuracy next time.
Once all training data has been processed, a new, smaller data set is used to check the accuracy of the fully trained algorithm. This step is called validation.
Quality of the training data: Garbage in, garbage out
No doubt: The accuracy of the AI algorithm can only be as good as the quality of the training data. If, for example, the training data contains many x-rays, in which a malignant tissue change was mistakenly considered benign by the human expert or vice versa, the AI will learn from false examples – which will affect its accuracy later on.
Obtaining high-quality training data for algorithms, however, often turns out to be difficult since our healthcare system is only slowly being digitalized. Data that has been carefully and manually validated is rare but the algorithm does require a huge amount of training data. When a high volume of digital data can be obtained, it is often from sources of inconsistent quality. This quantity and quality problem is further complicated by privacy issues in healthcare.
Most IT users know the phrase “garbage in, garbage out”: if the input data is garbage, the output will be garbage as well. This also holds true in AT where the accuracy of a classification algorithm always depends on the quality of the training data.
But not all types of low-quality output are immediately recognizable as such. There can be subtle biases in the classifications of an algorithm caused by an unbalanced composition of the training data. The accuracy of diagnostic algorithms, for example, is worse in populations whose data was underrepresented in the original training data.
Well-known examples are algorithms to classify malignant skin tumors which were trained with data from predominantly fair-skinned (Caucasian) individuals. These algorithms show lower diagnostic accuracy when they are supposed to make a correct diagnosis in a dark-skinned person.1
Recommended article
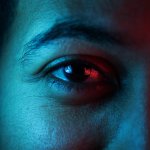
Article • Experts point out lack of diverse data
AI in skin cancer detection: darker skin, inferior results?
Does artificial intelligence (AI) need more diversity? This aspect is brought up by experts in the context of AI systems to diagnose skin cancer. Their concern: images used to train such programs do not include data on a wide range of skin colours, leading to inferior results when diagnosing non-white patients.
The source of the bias is not always obvious: In the US, an AI algorithm was used to estimate which in-patients would need additional care. The training data used the costs patients had previously incurred as a marker for disease severity.2 This led to the fact that for African-American patients additional care was less likely to be recommended because these patients had in the past incurred lower costs. This was not due to their lower disease severity but to their lower access to health care, i.e. a pre-existing systemic disadvantage.
The unbalanced composition of training data is often described with the acronym WEIRD: “white, educated, industrialized, rich and democratic countries” are overrepresented.
Women and the elderly are also disadvantaged
And it’s not just the ethnic and economic background that creates bias. Women are not fairly represented in AI either. For example, researchers suspect that women are overrepresented in the diagnosis of depression because, among other things, diagnostic algorithms query behaviors that are more common in women – regardless of clinical depression.3 In contrast, the Institute of Health Informatics at University College London showed that an AI algorithm for diagnosing liver disease in women had a significantly lower hit rate: it was wrong in 44% of women, but only in 23% of men.4
Recommended article
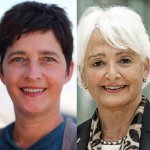
Article • Preprogrammed bias?
AI and the gender gap: Data holds a legacy of discrimination
Technologies based on artificial intelligence (AI) are considered the epitome of progress. However, the data AI algorithms use to draw their conclusions is outdated. It ignores the existence of biological sex and socio-cultural gender and their effects on individual health and disease states. German experts discussed the gender problem in healthcare AI at virtual.MEDICA 2020.
And another factor that can play a role in the development of bias: age. Facial recognition algorithms, for example, are less accurate in an elderly population.5 This is particularly disconcerting in view of the fact that robotics is increasingly being used in geriatric care, for example to inform and entertain elderly people and dementia patients. Particularly in this field there is substantial research being conducted to improve machine recognition of emotions based on facial expressions.
In order to address these biases and ensure equal treatment in a digitalized healthcare system diverse, balanced and high-quality training data sets are imperative. This also requires legal certainty with regard to the use of patient data for research and development. This issue will be addressed in Germany by the Federal Ministry of Health’s proposed laws6 in the context of the digitalization strategy and in the EU with the European Health Data Space.7
References:
- Wen et al.: Characteristics of publicly available skin cancer image datasets: a systematic review; The Lancet Digital Health 2021
- Obermeyer et al.: Dissecting racial bias in an algorithm used to manage the health of populations; Science 2019
- Cirillo et al.: Sex and gender differences and biases in artificial intelligence for biomedicine and healthcare; NPJ Digital Medicine 2020
- Straw I, Wu H: Investigating for bias in healthcare algorithms: a sex-stratified analysis of supervised machine learning models in liver disease prediction; BMJ Health & Care Informatics 2020
- Stypińska J, Franke A: AI revolution in healthcare and medicine and the (re-)emergence of inequalities and disadvantages for ageing population; Frontiers in Sociology 2022
- Bundesgesundheitsminister legt Digitalisierungsstrategie vor: „Moderne Medizin braucht digitale Hilfe“; Press release from the German Federal Ministry of Health, March 2023 (in German)
- European Health Data Space (EHDS); Overview from the European Commission
02.11.2023