Article • Multi-disciplinary approach
Nurturing integrated diagnostics in oncology
Under the roof of integrated diagnostics, radiology, laboratory medicine and pathology are forming a powerful alliance that could pave the way for completely new diagnostic and therapeutical approaches. In our interview, Hedvig Hricak, MD, PhD, Chair of the Department of Radiology at the Memorial Sloan-Kettering Cancer Center, New York, and Professor of Radiology at Cornell University, New York, explains the potential of integrated diagnostics for cancer patients and details the role of radiologists within the multi-disciplinary construct.
Image source: Shutterstock/Mopic
HiE: What is integrated diagnostics?
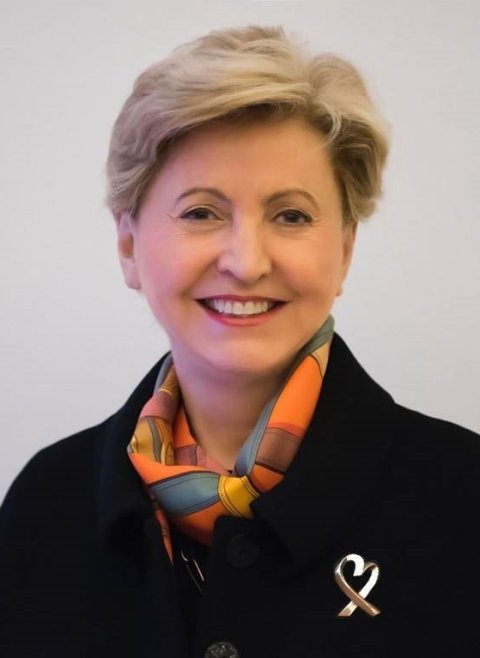
Prof Hricak: ‘Integrated diagnostics refers to the concept of the convergence of imaging, pathology, and laboratory tests with advanced information technology, and it has enormous potential for revolutionising the diagnosis and therapeutic management of many diseases, including cancer. It starts with gathering structured and quantitative data from different diagnostic disciplines and patient demographics, aggregating and placing them on a single platform, and through computational analysis enabling more precise understanding of biochemical-biological characteristics of cancer and thus allowing the development of predictive and prognostic biomarkers essential for pursuing the ultimate goal of precision oncology.’
Why is integrated diagnostics important for radiology?
‘Today, for almost all cancers, diagnostic imaging contributes essential information throughout the care pathway – from initial diagnosis and staging to treatment planning, assessment of treatment response, and long-term follow-up. Thus, it is critically important for the radiology community to take a prominent role in the development of integrated diagnostics.’
How does connecting the data from different disciplines benefit diagnostic performance?
Combining data from multiple disciplines will be essential for making oncology ever more precise and granular
Hedvig Hricak
‘Models built from multiple variables tend to have stronger predictive performance than individual variables. There are already several examples demonstrating that combining variables from multiple diagnostic disciplines is effective. For example, predictive nomograms that combine clinical, laboratory, pathology, and imaging data are commonly used to assess prognosis and aid in treatment selection for patients with prostate and breast cancers. Combining data from multiple disciplines will be essential for making oncology ever more precise and granular.’
Will integrated diagnostics play a role beyond genomics?
‘Discovering cancer genomic mutations has been one of the greatest advances in oncology. We soon realised that every cancer type – for example, breast or lung cancer – is very heterogenous and requires different treatment strategies. New therapies that target specific genetic mutations are the fastest growing class of treatment. However, we also learned that genomic data alone are not sufficient to determine in which patients these therapies will be effective. For example, approximately 13% of patients with non-squamous-cell lung cancer have been found to have an actionable KRAS G12 mutation for which there is a targeted treatment; some people who receive the treatment have a complete response, some have a partial response, while others have none. So clearly, we need to look for features other than a genomic mutation that may be associated with response (or lack of response) to the targeted treatment.’
Recommended article
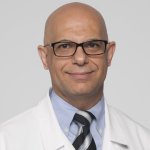
News • Controlling KRAS
New targeted gene therapy could stop lung cancer progression
A newly targeted therapy could help millions of lung cancer patients worldwide keep their cancers from spreading, says an expert at Cleveland Clinic, on the occasion of World Lung Cancer Day. Dr. Khaled Hassan, of the Hematology and Medical Oncology Department at Cleveland Clinic, explains the concept of KRAS targeted therapy – and why the approach should not be mistaken for a cancer cure.
What are the main steps in the development of integrated diagnostics?
‘To integrate data from multiple diagnostic modalities, we must first structure, or annotate, our data to make it machine-understandable. An example of this in radiology is building tumour segmentation datasets that can be used to train automated segmentation models, establishing a continuous data stream or data source. Developing this data infrastructure to include all diagnostic modalities – for example, clinical, radiology, laboratory, pathology, or genomic data – will lay the groundwork upon which multi-omic models can be built.
‘The development of a dashboard with user-friendly data aggregation is an important next step, as it will allow clinicians to view different types of patient data reflecting both the current disease status and longitudinal changes in a single display. However, only through data analysis by applying computational oncology algorithms will we develop prognostic and predictive biomarkers – powerful tools that can be used for treatment decisions and patient counselling. As research and technology progress, this display will increasingly include quantitative biomarkers generated not just from individual patients’ diagnostic data, but from population-level data set analysis from multiple sources.’
What are the main challenges to achieving integrated diagnostics?
‘There are several challenges for this; to begin with – and often underestimated – is the work required for standardisation of data collection, curation, aggregation, and abstraction. That means we need to gather large sets of high-quality data in a standardised, quantified format that can undergo computational analysis.
Recommended article
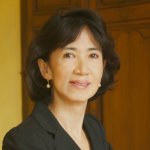
Interview • Hybrid imaging
‘We need to drive standardisation in scanning’
Professor Regina Beets-Tan, President of the European Society of Radiology (ESR), looks on possible future developments in cancer imaging. AI is very promising in this regard, 'However, it has proven to be a problem that the data with which the artificial intelligence is fed must be very homogeneous,' the expert observes.
‘A second challenge is the refinement and application of machine learning and other forms of artificial intelligence (AI) to extract meaningful biomarkers from very high-dimensional data; a third challenge is the development and implementation of rules for data access and governance, and a fourth challenge is ensuring that the results produced through data integration are robust and reproducible.
‘Several other critical challenges lie not only in developing, but in maintaining and continuously updating the data sets. However, I believe the first of the challenges mentioned above is the critical bottleneck, as it requires technical and cultural adaptation. To address it will require resources, people, and intensive communication and collaboration between disciplines, within and across institutions and with industry, both nationally and internationally.’
Which upcoming technologies will, in your opinion, have the biggest impact on the further development of integrated diagnostics?
‘In radiology, we already have vast quantities of digitised data on hand from currently available imaging modalities, and digitisation is also progressing in pathology. Thus, I believe that, rather than new diagnostic technologies, machine learning and other AI tools will have the greatest impact on the further development of integrated diagnostics. Given the great challenge and importance of structuring and annotating our data as a foundational step, I look to AI as a potential tool to accelerate the annotation process and avoid burdening our clinicians with this task.
We need a true team approach with the intense involvement of experts from diverse disciplines
Hedvig Hricak
‘An example is the use of semi-supervised learning, in which a small amount of labelled data is combined with a larger unannotated dataset to train a high-performing AI model that can in turn annotate more data. Thus, the value of AI is not only in extracting biomarkers from our integrated data, but also in helping establish the structured data streams themselves.’
What is the role of computer analysts in the development of integrated diagnostics?
‘The role of computer engineers is essential. Integrated diagnostics is the ultimate example of the horizontal integration and convergence of life science, physical science, and engineering. To quote Shirley Tilghman, the 19th President of Princeton University, “As much as new ideas are fundamental to the advancement of science, technologic innovations are the engine of scientific progress.” To fully develop, advance, and maintain the field of integrated diagnostics, we need a true team approach with the intense involvement of experts from diverse disciplines, including those from physical and data sciences, and physicians from multiple specialties; the latter category will of course include radiologists.’ (WB)
Profile:
Hedvig Hricak, MD, PhD, is Chair of the Department of Radiology at the Memorial Sloan-Kettering Cancer Center, New York, and Professor of Radiology at Cornell University, New York. She is renowned for developing and validating clinical applications of novel imaging technologies to address pressing patient needs, particularly in oncology. She was one of the pioneers in establishing the clinical value of MRI for gynaecologic and urologic cancers. To date, she has published more than 450 original peer-reviewed research articles along with 230 peer-reviewed editorial review manuscripts and 18 books. Prof Hricak received numerous awards including the Marie Curie Award from the Society of Women in Radiology; the gold medals of the International Society for Magnetic Resonance in Medicine, the Association of University Radiologists, the European Society of Radiology, and the RSNA. She also received honorary doctorates from the Ludwig Maximilian University of Munich in Germany and the University of Toulouse III, Paul Sabatier in France.
20.01.2022