Article • AI structure
Machine Learning: into the pumpkin patch
Standardised and well-structured data, as well as the definition of clear objectives, are indispensable prerequisites for artificial intelligence implementation into clinical processes.
Report: Michael Krassnitzer
Source: Pixabay/birgl
‘Ask not what artificial intelligence can do for you but what you can do for artificial intelligence!’ This variation on John F Kennedy’s famous quote comes from Dr Ben Glocker, Senior Lecturer in Medical Image Computing at Imperial College London, UK. The occasion was a lecture given by the computer scientist at the European Radiology Congress (ECR 2018) on how machine learning can be routinely implemented in medical image analysis.
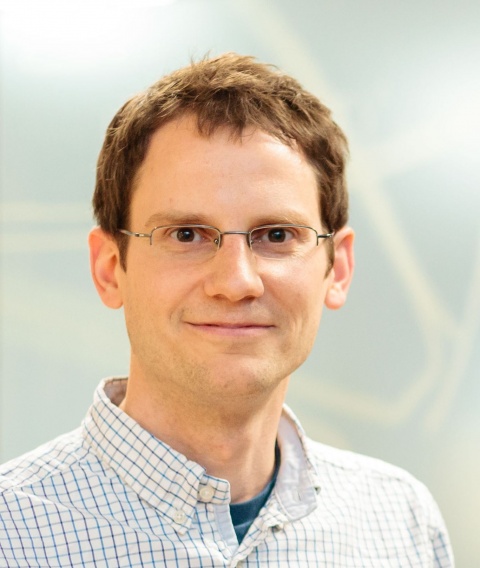
Artificial Intelligence (AI) was among the main topics at the congress; this also includes machine learning and its subdivision ‘deep learning’, which is based on so-called neural networks. AI entails an artificial network learning from examples and recognising inherent patterns and regularities by itself. Based on this, numerous applications are already used in imaging, such as image segmentation, where an algorithm precisely marks out certain anatomic or pathological structures from their environment (e.g. healthy brain tissue from tumour tissue in MRI brain scans, or whole body MRI scans of organs). Machine learning also facilitates the detection of new disease patterns, the extraction of clinically relevant information from multi-dimensional, multimodal image data that would otherwise remain concealed.
‘It’s basically a mathematical function,’ says Glocker explaining machine learning. ‘This function is fairly complex, and we also cannot say exactly what is looks like, but like every function it has an input and an output.’ According to this UK-based German computer scientist, there are frequently misleading ideas around the necessary input. ‘The approach “We have a lot of data and are going to do something with it with the help of machine learning” is not the best starting point.’ Therefore, Glocker’s first advice is: If you want to use machine learning successfully you need to start with a clearly defined objective. ‘Machine learning is always geared towards a very specific task,’ he emphasises.
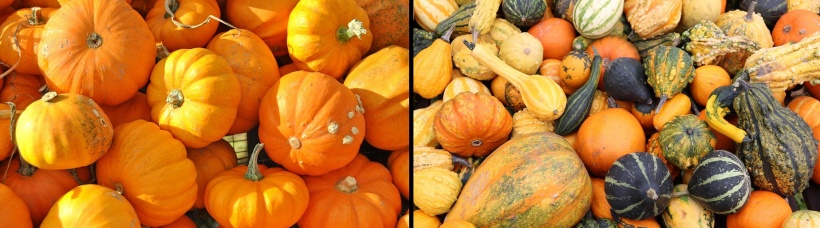
Source: Pixabay/emiller5605/birgl
Machine learning can only begin when data is usable and when a specific task has been determined
Ben Glocker
Another common obstacle to the use of machine learning is the quality of data. Glocker explains that, on many occasions, clinicians promise him homogenous data sets for respective projects, which actually turn out to be different, insufficiently structured data. He compares this to pumpkins: ‘We are expecting a box of these vegetables of equal size, equal shape and equal colour, but what we actually receive is a delivery of pumpkins of different sizes and different varieties.’ For data to be used for machine learning it not only needs to be correct and labelled but most definitely also standardised and labelled in a standardised way.
‘Machine learning can only begin when data is usable and when a specific task has been determined,’ Glocker concludes. The development of an AI application also needs the relevant medical expertise for its use: ‘The experts must be involved in all stages of development,’ the computer scientist emphasises. He means that medics and computer scientists must work together in interdisciplinary teams. He also offers other inevitably important advice: ‘Artificial Intelligence applications must be integrated seamlessly into clinical processes.’
Session: Ben Glocker, Machine learning for analysing medical images, ECR 2018
Profile:
Ben Glocker PhD is a senior lecturer in medical image computing at Imperial College London, UK, and deputy head of its biomedical image analysis group. He gained his PhD from the Technical University Munich, Germany. He was then a post-doc at Microsoft Research and a research fellow at the University of Cambridge, United Kingdom. In his research he specialises on applying machine learning and artificial intelligence to automate image analysis.
30.10.2018