Image source: Unsplash/Abdrahim Oulfakir
Article • Experts point out lack of diverse data
AI in skin cancer detection: darker skin, inferior results?
Does artificial intelligence (AI) need more diversity? This aspect is brought up by experts in the context of AI systems to diagnose skin cancer. Their concern: images used to train such programs do not include data on a wide range of skin colours, leading to inferior results when diagnosing non-white patients.
Report: Mark Nicholls
New research, presented at the National Cancer Research Institute’s NCRI Festival in the UK in November, points to most training data for such systems being mainly from patients with lighter skin. Researchers say there is an urgent need to improve upon these datasets on skin cancers and other skin lesions.
While AI is being increasingly used for diagnostic purposes, accuracy depends heavily on the information the system is trained on. Dr David Wen, who led the study published in The Lancet Digital Health, said: ‘AI programs hold a lot of potential for diagnosing skin cancer because it can look at pictures and quickly, and cost-effectively evaluate any suspicious spots on the skin. However, it’s important to know about the images and patients used to develop programs, as these influence which groups of people the programs will be most effective for in real-life settings.’ He added: ‘Research has shown that programs trained on images taken from people with lighter skin types only might not be as accurate for people with darker skin, and vice versa.’
Severe underrepresentation in datasets
The latest findings, which follow previous research in this area by other groups raising concern over the lack of image data representing people of colour, come from a review of 21 accessible datasets on skin lesions around the world, with more than 100,000 pictures. The work conducted by Dr Wen and his colleagues from the University of Oxford showed that pictures of people with darker skin are under-represented in these datasets. Fourteen of the 21 sets gave information on which country the depicted persons came from; of those 14 sets, nine contained images from European countries, but only a small percentage of images included information about skin colour or ethnicity.
Among the 2,436 pictures where skin colour was stated, only 10 were of brown skin and only one was of dark brown or black skin. Among the 1,585 pictures with information on ethnicity, none were from people with African, Afro-Caribbean, or South Asian backgrounds.
Inconsistent information
This highlights the need for quality standards for characteristics and metadata reporting for skin image datasets
David Wen
The researchers also found gaps in other aspects of the training data: they point out inconsistencies in the devices used for image acquisition, images selection, and evidence of ethical approval or patient consent. Dr Wen added: ‘There was limited information on who, how and why the images were taken. This has implications for the programs developed from these images, due to uncertainty around how they may perform in different groups of people, especially in those who aren’t well represented in datasets, such as those with darker skin. This can potentially lead to the exclusion or even harm of these groups from AI technologies. ‘Although skin cancer is rarer in people with darker skins, there is evidence that those who do develop it may have worse disease or be more likely to die of the disease. One factor contributing to this could be the result of skin cancer being diagnosed too late.’
Going forward, Dr Wen and his colleagues hope to create quality standards for health data used in AI development which will include information on who should be represented in datasets and which patient characteristics should be recorded. In their paper, they concluded: ‘This is the first systematic review of open-access skin image datasets, highlighting limited and variable metadata reporting, limited applicability of datasets to real-life clinical settings and restricted representation of populations, with implications for generalisability. This highlights the need for quality standards for characteristics and metadata reporting for skin image datasets. There is an urgent need for publicly available image datasets to be transparent, have a minimum metadata requirement, and to represent the intended populations that they will be deployed in, in order to maximise accuracy and applicability of skin cancer detection algorithms.’
Recommended article
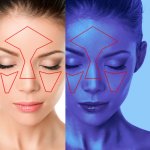
Article • Skin cancer identification
Dermatology & AI: The need to quantify skin tones
Although artificial intelligence (AI) tools and smartphone apps that help identify suspicious moles and potential skin cancers are starting to proliferate, dermatology informatics has far to go before becoming a clinically adoptable technology. Many challenges need to be resolved, not least of which is the need for more image data representing people of colour.
Profile:
Dr David Wen is a National Institute for Health Research (NIHR) dermatology academic clinical fellow at the University of Oxford and Royal Berkshire Hospital, United Kingdom. He completed pre-clinical medical training at the University of Cambridge, clinical training at the University of Oxford, and subsequently undertook general medical training at Ealing and Chelsea and Westminster Hospitals. This was followed by a dermatology fellowship in epidermolysis bullosa at Solihull Hospital, and an MRes in Clinical Health Research at the University of Birmingham.
10.01.2022