tumour parcellation defined on a 4-D dynamic contrast-enhanced magnetic
resonance imaging (DCE-MRI) scan based on signal enhancement characteristics.
Article • Smart techniques
Machine learning is starting to reach levels of human performance
Machine learning is playing an increasing role in computer-aided diagnosis, and Big Data is beginning to penetrate oncological imaging.
Report: Mélisande Rouger
However, some time may pass before it truly impacts on clinical practice, according to leading UK-based German researcher Professor Julia Schnabel, who spoke during the last ESMRMB annual meeting. Machine learning techniques are starting to reach levels of human performance in challenging visual tasks. Tools such as the convolutional neural network (CNN or ConvNet), a class of deep neural networks that has been applied to analysing visual imagery, have become instrumental in segmentation tasks.
Analysing such huge data is still a challenge
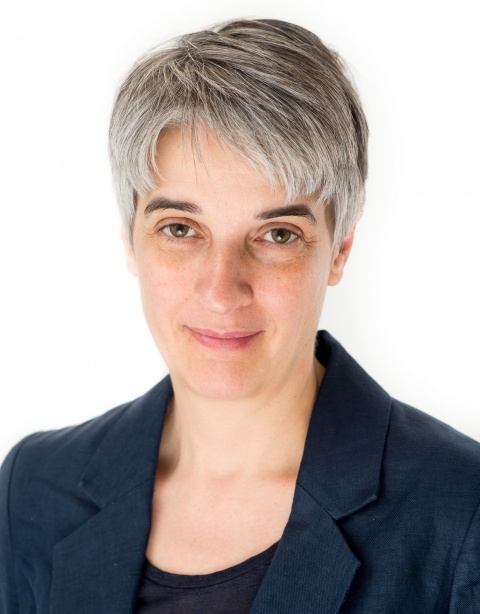
However, a number of obstacles remain before adequate image analysis arrives, starting with the huge amount of data analysts must work with, according to Professor Schnabel, computational imaging expert at King’s College London. ‘In imaging, the challenges are that we work in 3-D or 4-D, and we have a lot of features to deal with. If we’re lucky, we deal with hundreds or thousands, but not millions of images, so we don’t have a high number of image data to work with. We have this whole sample size problem.’
The professor also identified the high associated cost and imperfection of training data. Training data may be wrongly labelled, depending on the expertise of the observer. Furthermore, machine learning is resource-intensive: only specialists and consultants can perform special tasks. ‘I personally couldn’t distinguish a glass nodule from a semi-solid nodule. Only specialist consultants and expert radiologists can do that,’ she pointed out. For a disease such as cancer, the image analysis team needs confirmation from pathology, which is often difficult to obtain.
For brain imaging, where different protocols exist, one sees different appearance of the same disease on different image protocols for the same patient and between patients. ‘Disease location and size of these pathologies may vary quite significantly, and the appearance of disease may be very localised: it may be a very sharp “blob”, or it may be very diffused or infiltrated,’ she explained.
Deep neural networks
There is often an imbalance in the training or test data
Julia Schnabel
The professor shared practical advice on how to work with CNNs appropriately. She stressed the size of the receptive field of a CNN will determine the amount of information that will be obtained. ‘The size of patches used is important, since a large receptive field increases computation and memory requirements, and (max) pooling leads to loss of spatial information. In contrast , if you use very small patches, they are more susceptible to noise.’ As a solution, Schnabel points to using a multi-scale approach, i.e. having smaller patches operating on small filters and larger ones on larger filters, and putting them together in the end.
Oncological image analysis brings challenges of its own. Machine learning-based segmentation often degrades when deployed in clinical scenarios. This is caused by differences between training and test data due to variations in scanner hardware and scanner protocols and sequences, Schnabel explained. ‘There is often an imbalance in the training or test data because of a different ratio of healthy vs. pathological cases, individual patient variability and individual disease variability – also within the same patient. For example, lesions in the liver usually are a secondary cancer, caused by a primary cancer elsewhere, such as in the colorectum.’
Therefore, it is crucial to choose the appropriate network architecture. Currently three models in literature are interesting: DeepMedic, FCN (in Deep Learning Toolkit) and U-Net, which owes its name to its ‘U’ shape. ‘These networks use different approaches and for all these, there is the good, the bad and the ugly,’ she pointed out.
An ensemble of multiple models and architectures
All three networks use CNN based approaches with good performance, but there are a lot of meta-parameters – more than input cases –, and the architecture and configuration influence performance and behaviour. The ugly part is that chosen models and parameters may be suboptimal of other data and applications. ‘Results and conclusions may therefore be strongly biased,’ she said. One solution could be to use an ensemble of networks; one such example is ‘EMMA’ (ensemble of multiple models & architectures), for which performance is insensitive to suboptimal configuration and behaviour is unbiased by architecture and configuration.
For segmentation in colorectal cancer with DCE MRI, Schnabel and team extracted variability of normalised signal intensity curves from the dataset using principal component analysis. ‘It’s a very simple technique. We just looked at the mean signal intensity of curves embedded within an over-segmentation approach, called superpixels or supervoxels in 3-D. We then classified perfusion supervoxels in unseen cases using support vector machines to obtain tumour segmentations.’ This automated method was found to perform within the inter-rate agreement of two expert observers, but a correction step was needed when transferring the segmentation mask from the T2W to T1W DCEMRI sequence, she noted.
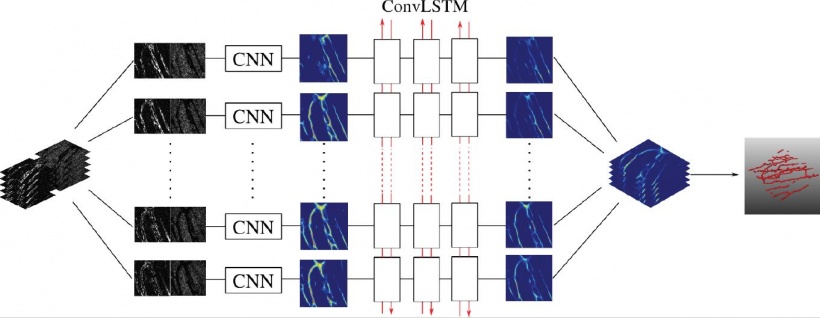
Gaining a more accurate tumour segmentation
‘Tumours have considerable variation in shape, so we need to bring in some anatomical context and, to do so, we have developed a graphical representation of the neighbouring anatomy. We can improve segmentation by taking into account both local and global relationships; so we know where the bladder, lumen, etc. are, and build them into a pieces-of-parts model. By classifying these pieces of parts, we can reduce false positives and obtain a more accurate final tumour segmentation,’ she explained.
Schnabel and team also performed segmentation inside the tumour using a technique called tumour parcellation, to extract locally meaningful, contiguous perfusion subregions from DCE-MRI scans. Ten female CBA mice with subcutaneously implanted CaNT tumours were scanned over eight days to monitor tumour growth and clustering of derived perfusion supervoxels. Imaging casculature helped to look even deeper inside the tumour. ‘We used 3-D fluorescence confocal microscopy, an imaging technique that has very anisotropic voxels of a few microns size. Endothelium and tumour cells were both fluorescently labelled, and approximately 60 slices where acquired in the z-direction. Vasculature was visible up to 30 slices from the surface.’ In lung cancer image analysis, most efforts concentrate on lung nodules or lymph node detection in lung CT. Deep learning is now largely replacing conventional CADe and CADx methods, which were based on texture analysis, handcrafted features and simple classification techniques. According to Schnabel, using deep learning, detection rate is generally very high and the main focus is now on false positives reduction.
High dimensional multimodality datasets are not big data
Machine learning is a promising tool in oncological imaging and image analysis, and the challenge is to find the right model parameters for good estimation and generalisation. ‘We have high dimensional and multi-modality datasets, but it’s not really big data, it’s rather dense data. Cohort studies which collect large amounts of data ,’ she added, ‘will help a lot in that sense.’
Profile:
Julia Schnabel PhD joined King’s College London, in the UK in July 2015 as Chair in Computational Imaging at the Division of Imaging Sciences & Biomedical Engineering, taking over the Directorship of the EPSRC Centre for Doctoral Training in Medical Imaging, which is jointly run by King’s College London and Imperial College London. She is also Visiting Professor in Engineering Science, at the University of Oxford.
29.01.2018