Image source: Adobe Stock/Lee
Article • Interpreter of maladies
Will AI help realize the promise of precision medicine?
Machine learning and artificial intelligence (AI) have the potential to transform cancer treatment management worldwide. Their ability to rapidly analyse and integrate routinely acquired diverse data will improve the accuracy and effectiveness of precision medical treatments.
Report: Cynthia E. Keen
Anant Madabhushi, PhD, Professor of Pathology, Radiation Oncology, Radiology, and Urology at Case Western Reserve University School of Medicine in Cleveland, Ohio, discussed what the near future may offer in the closing lecture of the Society for Imaging Information in Medicine’s (SIIM) recent annual meeting. The topic of AI’s utilization in diagnostic imaging had dominated the conference. Dr Madabhushi’s lecture, entitled “Interpreter of Maladies: AI for Precision Medicine,” offered a broader perspective.
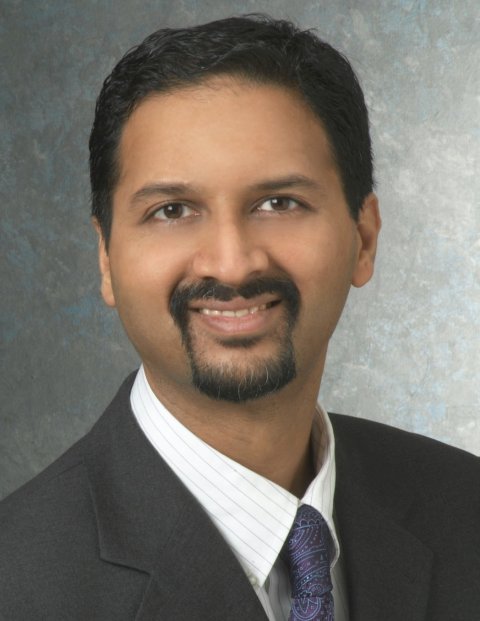
Image credit: Case Western Reserve University
As the director of Case’s Center for Computational Imaging and Personalized Diagnosis, Dr Madabhushi has secured almost US $80 million in grant funding for his team to develop novel AI and machine learning approaches for the diagnosis, prognosis, and prediction of therapy response for a number of disease conditions. These include cancers, cardiovascular disease, kidney disease, and eye disease. ‘Cancers, like prostate cancer, tend to be treated aggressively,’ he explained. ‘Aggressive treatment saves lives, but often at the cost of debilitating toxicities and at a high financial cost to patients and insurance companies alike. If a patient’s cancer could be risk stratified using routinely acquired data, the cancer could be characterized more accurately, and the most appropriate treatment prescribed.’
'AI combined with computational imaging is diagnostic, prognostic, and predictive. AI’s ability to identify presence and type of disease, predict outcome and risk of progression, and predict response to treatment will help truly realize the promise of precision medicine,' Dr Madabhushi emphasized.
Beware of bias
Digital pathology makes it possible to take quantitative measurements of individual cells, and analyse their shape, spatial architecture, and spatial arrangement. AI can identify individual cells, cancer nuclei, and lymphocytes and start to pull out features that provide a quantitative phenotype that a pathologist using a microscope cannot identify.
Dr Madabhushi cautioned that AI programs must
- use transparently engineered software,
- be robust and reproducible across all sites, and
- be diverse population inclusive.
Proprietary software, for example, may not identify changes that occur unnoticed outside data sources, such as an automated computer software update, and different ethnicities may have different biological differences at the cellular scale of a disease. AI algorithms will not be effective if their training data has population bias.
Recommended article
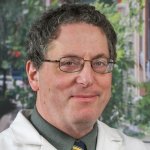
Article • Heard at SIIM 2021
AI in radiology: unexpected benefits, unintended consequences
Artificial intelligence (AI) could match the impact of PACS on radiology. Covid-19 stimulated the development and testing of AI diagnostic-aiding tools in radiology, an unintended consequence of the pandemic. More image data sets have been created to train AI software – an unexpected benefit for radiology research.
Collagen structure identified as prognostic biomarker
Researchers at Case have been using AI for deep phenotyping, using a powerful segmentation and classification approach to do tissue partitioning. As one example, they discovered that the patterns of collagen of patients with prostate cancer could predict risk. Using clinical trial data, the researchers determined that patients with very coherently organized collagen had very short survival outcomes, compared to patients with haphazard collagen orientation.
‘Because collagen potentially represents the “highway” on which cancer cells hitch a ride and migrate, it makes sense that the more ordered and structured the “highway”, the easier it is for cancer cells to move from primary to secondary sites,’ he said. ‘One of the biggest challenges in prostate cancer treatment is to determine which patients after surgical resection are likely to have a recurrence, even though they have clean margins. This knowledge will enable oncologists to identify patients at high risk for recurrence.’
Other applications
AI has the potential to make diagnosis, prognosis, and treatment planning equitable to all economies of the world
Anant Madabhushi
The researchers also created a machine learning classifier to determine risk of progression and recurrence in patients with oropharyngeal head and neck cancer and in early-stage non-small cell lung cancer. This helped identify which patients needed adjuvant chemotherapy and those who did not.
Furthermore, Dr Madabhushi ’s team used AI to differentiate between radiation necrosis and tumour recurrence seen on MRI. ‘This is an issue that challenges radiologists because the inability to definitively differentiate these may require intracranial biopsy. Our group found a new way of characterizing the textual patterns by identifying the dominant edge of every voxel on a voxel-by-voxel basis and creating entropy maps. The higher the entropy, the more associated with recurrent tumours, as validated by clinical trial data.’
Two other very important benefits of AI will be rapid turnaround time, much lower cost, and potentially better performance than laboratory tests which require sending samples to a central location. ‘AI has the potential to make diagnosis, prognosis, and treatment planning equitable to all economies of the world,’ he concluded.
Profile:
Anant Madabhushi, PhD, assumed a new position in July 2022 as Professor of Biomedical Engineering at Emory University. He previously was the Professor of Pathology, Radiation Oncology, Radiology, and Urology at Case Western Reserve University School of Medicine in Cleveland, Ohio, and the Donnell Institute Professor of Biomedical Engineering at Case School of Engineering. Dr Madabhushi also was the director of Case’s Center for Computational Imaging and Personalized Diagnosis.
08.09.2022