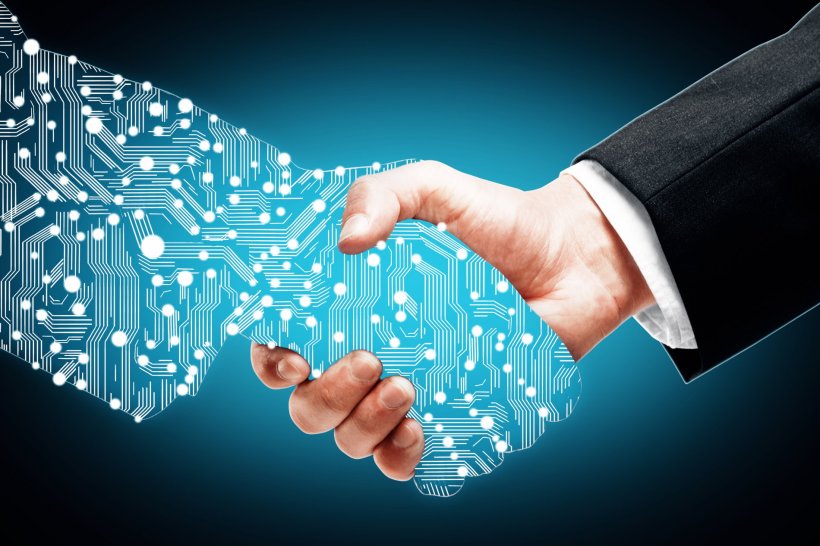
Image source: Adobe Stock/
Article • Diversity, transparency, flexibility
Building trustworthy AI systems for cancer imaging
Building artificial intelligence (AI) tools that clinicians and patients can trust, and easily use and understand, are core to the technology being successfully deployed in healthcare settings. AI expert Dr Karim Lekadir will focus on the importance of these elements during a presentation at ECR in Vienna on July 15, as developers seek to create new AI models to support image-based diagnosis and care.
Report: Mark Nicholls
In a session focusing on “Big data and artificial intelligence in cancer imaging”, Dr Lekadir will concentrate on different methods for building and validating artificial intelligence workflows, and how federated big data repositories can enhance future AI applications in cancer imaging.
Questioning the performance primacy
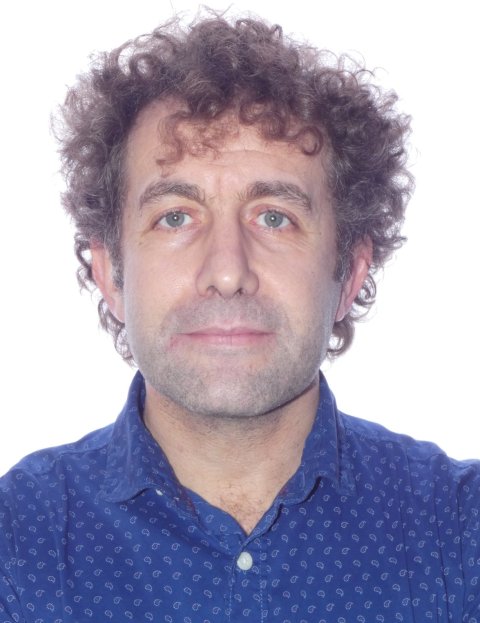
Photo: supplied
Speaking ahead of ECR, he highlighted the creation of trustworthy systems as a key step in AI development. That, he added, has to take into consideration all aspects of the system. ‘I will focus on the value of trust in future AI solutions because it is one thing to build an AI solution that is performant, but quite another to build AI solutions that people can trust and will deploy and use,’ said Dr Lekadir, who is Director of the University of Barcelona’s Artificial Intelligence in Medicine laboratory.
‘Until now, people have focused on the performance in relation to accuracy and robustness, but that is not enough. We need to go beyond that and take in other elements, such as fairness. An AI solution may be robust but may discriminate against certain underrepresented groups. It may work well with white patients but not so much with black patients, for example, or with men but not female patients.’
Recommended article
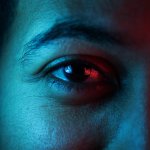
Article • Experts point out lack of diverse data
AI in skin cancer detection: darker skin, inferior results?
Does artificial intelligence (AI) need more diversity? This aspect is brought up by experts in the context of AI systems to diagnose skin cancer. Their concern: images used to train such programs do not include data on a wide range of skin colours, leading to inferior results when diagnosing non-white patients.
Light into the AI black box
He also pointed out the value of usability and explainability, for both clinicians and patients. ‘Because AI models are often complex, users can sometimes be resistant to using them, so we need to make the AI decisions understandable,’ he said. Transparency and traceability are also important in light of constantly changing data and users. Models that do not take these factors into account run the risk of quickly losing their robustness, becoming unusable. ‘We need to monitor and trace these tools over time, because something that makes AI different from other technologies is that it is highly dynamic and changing over time,’ he added.
Inclusive approach to anticipate barriers
The expert argued that adopting a ‘highly-inclusive approach when designing, building, validating and deploying the AI solution’ is pivotal in its successful introduction into a clinical setting. A crucial oversight of the past, he suggested, was that engagement with clinicians and other stakeholders had been minimal when AI tools were being developed. ‘We are realising the importance of having a multi-stakeholder and human-centred approach where we involve developers, clinicians, patients, data experts, ethicists, social scientists, and regulators so we can anticipate some of the implementation barriers,’ added Dr Lekadir. This co-creation enhances technical robustness, applicability, and clinical safety and means problems can be anticipated in advance with appropriate solutions designed and implemented.
Thorough evaluation of AI
‘If you evaluate without paying attention to ethnic diversity, you may run into problems later when the tool is deployed in practice
Karim Lekandir
He emphasised the role of thorough evaluation, such as ensuring a system covers patients of different ethnic backgrounds. ‘If you evaluate without paying attention to ethnic diversity, you may run into problems later when the tool is deployed in practice,’ he cautioned. ‘It may not work well for certain populations.’ External evaluation, in a hospital or centre different to the one where the model was built in, is therefore critical. He also underlined the need for compliance with AI regulations.
‘In my presentation, I will describe a multi-stakeholder, multi-faceted, holistic approach to building AI to take into account clinical, technical, ethical and legal aspects and the importance of engaging all stakeholders and end users, anticipating problems, and risk and mitigation measures,’ he concluded.
*ESR/EIBIR 16 – The big data and artificial intelligence in cancer imaging session (July 15, 4-5pm CEST) will include further lectures looking at the main aspects on data repositories in cancer imaging; EIBIR (European Institute for Biomedical Imaging Research) activities and upcoming funding opportunities, plus a panel discussion examining the question “can causality be inferred from medical images?”
Profile:
Dr Karim Lekadir is from the Department of Mathematics and Computer Science at the University of Barcelona, and Director of the university’s Artificial Intelligence in Medicine laboratory. His research interests focus on big data and AI for biomedical applications.
13.07.2022