Article • “I, Algorithm“
Applying the 3 laws of robotics to AI in radiology
Ethical considerations continue to fuel the discussion around artificial intelligence (AI), a panel of experts showed at ECR 2022.
By Mélisande Rouger
Image source: Adobe Stock/envfx
When science fiction author Isaac Asimov devised a set of rules for robotics in 1942, little did he know how relevant they would still be 80 years later. The rules, or laws, famously featured in the novel “I, Robot”, state that:
- a robot may not injure a human being or, through inaction, allow a human being to come to harm;
- a robot must obey orders given by human beings except where such orders would conflict with the First Law; and
- a robot must protect its own existence as long as such protection does not conflict with the First or Second Law.
These three laws could also apply to AI, suggested Federica Zanca, Founder at Palindromo Consulting, who opened the ECR session.
AI may not injure a human being
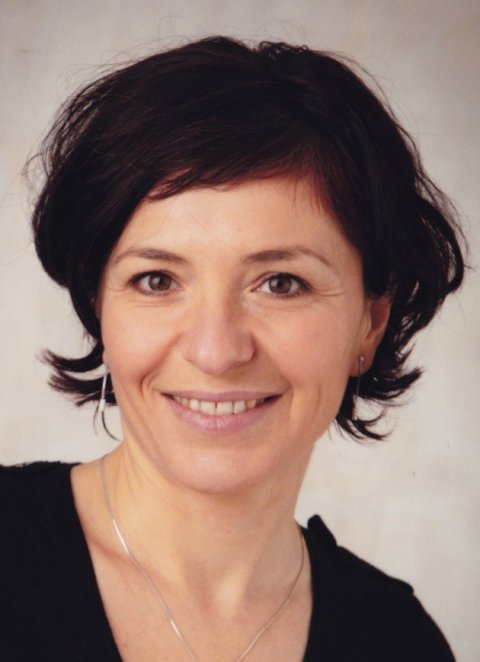
Image source: Palindromo Consulting
‘Radiologists should not trust an algorithm blindly and they should be aware of any issues related to the algorithm that have a negative impact on patient care,’ she told the audience. For example, some AI tools might be subject to what is called external drifting of the model: the data input may change over time (e.g. from the introduction of a new scanner, or changes in population mixture) and therefore models developed on limited sample sizes may be subject to degradation of the model performance over time. ‘Most AI models today are locked,’ Zanca said. ‘They have been trained with specific data to get a certain output and are not learning from new data to adapt accordingly.’
Solutions to remedy that situation are in the pipeline and the FDA has already worked on regulation to approve self-learning tools. However, radiologists need to beware of self-learning models turning against them and errors sneaking in that compromise the wellbeing of patients, she insisted. ‘Quality assurance should be performed with each imaging medical device including AI software,’ suggested Zanca, who is working on an application to track operational and clinical AI over time.
‘We want to know what happens when humans use AI’
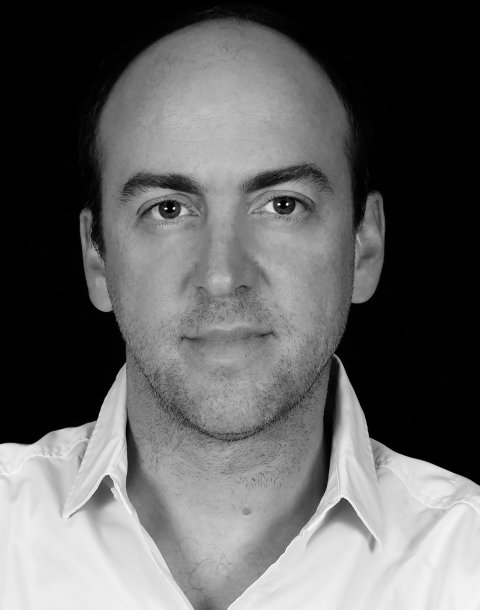
Photo: supplied
Gaspard d’Assignies, Co-founder and Director of the Medical Strategy at Incepto Medical, is also involved in the project. Radiologists need to know how to accept, reject or modify an algorithm, he told delegates in the next talk. ‘We need to understand how these algorithms are going to modify patient care,’ he said. ‘So we need prospective, real-life studies that compare reader performance of man with machine vs. man and machine alone over time. We want to know what happens when humans use AI.’
The central question that all these considerations should revolve around, he added, is: How can AI improve workflow and accuracy according to the clinical context? ‘The design purpose must be very clear, and studies must be conducted to validate these improvements.’ When creating an AI solution, a doctor should always be in the loop to check when the machine is right or not. ‘We don’t want a black box effect and we also must make sure that our algorithm is not biased and includes all the people it is supposed to represent,’ he said.
When should AI disobey humans?
This is where the second of Asimov’s laws comes into play: AI should not obey the radiologist when he or she is wrong, Zanca carefully suggested as she presented the results of a study on bone age assessment. ‘In that survey, if you asked a radiologist to do the measurement, you would possibly get ten different values. This may have consequences on treatment and the patient’s life. The machine is doing much better, and it always gives the same result for this type of measurements on the same image. For this indication, I think the machine is better suited,’ she concluded.
AI puts safeguards that may help prevent human errors, d’Assignies added. ‘For example, with a pulmonary nodule detection tool, the nodule threshold can't be set at 20mm, otherwise nothing will be detected,’ he explained. Radiation dose could be another instance where the machine should disobey an ill-advised order. ‘AI could warn when a radiologist would use too high a dose,’ Zanca said. ‘Today, we have a simple limit protection that is already embedded in the system. But AI could be used to drive exposure parameters.’ So, if the human wanted to go beyond a certain radiation dose, the system could refuse, as this would be harmful for the patient.
Recent reader performance studies focus on augmented AI, i.e., comparing human performance with the machine vs. human performance alone. However, radiologists should beware the risk of deskilling that comes with augmented AI; when professionals get used to a system doing something for them, that run the risk of losing the ability to perform this task themselves. ‘It’s essential that training radiologists also learn to detect lesions without AI,’ she said. ‘If the system fails, radiologists still need to be able to interpret images without AI.’
Profiles:
Federica Zanca is a senior clinical medical physicist who has been conducting research in medical imaging for the past 22 years. As former professor at KU Leuven and Chief Scientist and director at GE Healthcare, she is familiar with both academic and industrial settings. Her research interests include mammography, CT imaging, interventional and general radiology, medical imaging perception and AI in medical imaging. Through investigation of equipment performance, organization of quality control in imaging systems, control of radiation and contrast hazard, her research aims at delivering high-quality care of patients undergoing imaging procedures. She currently works as a consultant to the industry and hospitals through her company, Palindromo Consulting.
Gaspard d'Assignies is a radiologist, former resident and fellow at the Paris hospitals. He specializes in oncology and interventional radiology. He is currently a practitioner at the Havre Hospitals, where he leads the interventional imaging unit. As an active member of the Telediag teleradiology network, he is part of one of the first telemedicine groups in France. He is Co-founder and Director of Medical Strategy at Incepto Medical, a company that provides a platform for co-creation and distribution of AI tools in medical imaging.
12.09.2022