Article • Workflow optimisation
The potential of AI in breast imaging efficiency
The contribution of Artificial intelligence (AI) has great potential in breast imaging efficiency, Professor Linda Moy MD told attendees at the 2021 Society of Breast Imaging/American College of Radiology (SBI/ACR) Breast Imaging Symposium this April. AI models for breast imaging have focused mainly on the diagnostic classification and detection of breast cancer. However, AI applications for workflow optimisation can provide support for interpretation tasks and increase overall operational efficiencies in all aspects of the breast imaging workflow chain.
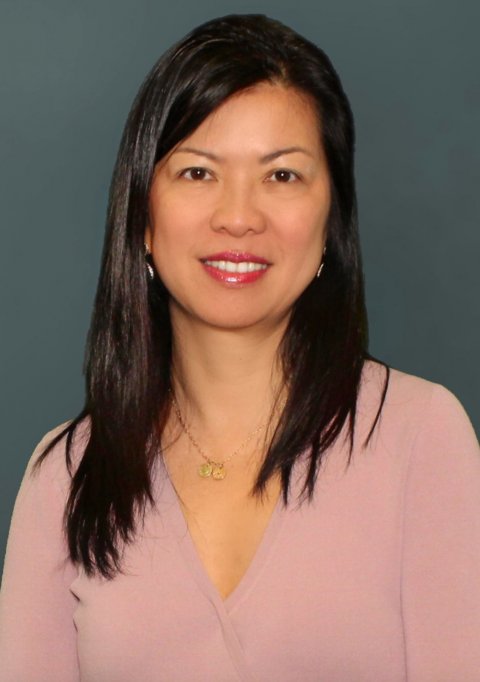
‘There are currently numerous AI applications in development that have the potential to benefit breast imaging, from test ordering to report communication,’ said Moy, who is professor of radiology at New York University’s Grossman School of Medicine. ‘At a time when there’s a shortage of breast imagers, there’s increasing patient volume, and with increased utilisation of digital breast tomosynthesis (DBT) and breast MRI, an increasing number of images in an exam. Radiologists can benefit from intelligent, automated, time-efficient tools to perform or expedite repetitive tasks. These can help increase efficiency for breast imagers.’
The workflow chain in imaging includes multiple segments, starting with an order for the patient. This needs to be reviewed for imaging appropriateness and the protocol to be used, scheduled, performed, managed, reported with results communicated, and billed. A staff member is needed at each step to integrate information and accurately pass the information along to the next step. AI can potentially address every aspect of the workflow, Moy believes, ‘enhancing practice workflow efficiency, reducing variability, and improving quality.’ ‘Most attention for AI in breast imaging goes to pixel-based image analysis, with a focus on narrow AI tasks including detection, classification, segmentation, prepopulating a report, prediction of breast cancer risk, and treatment response prediction,’ she explained. ‘Less attention is being paid to radiology workflow, but I think the greatest potential for AI lies in making these back-end processes more efficient. The current limitation and difficulty are in integrating these tools into our RIS and PACS, because both can be quite inflexible.’ PACS worklists have limited prioritisation capabilities, such as exams flagged as STAT.
Recommended article
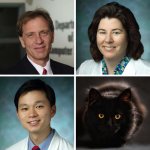
Article • Deep Computer-Aided Triage
DeepCAT: An AI tool to improve high volume mammography reading workflow
A new deep learning system to help radiologists improve their workflow efficiency when reading high volumes of screening mammograms is being developed at Johns Hopkins University’s Radiology Artificial Intelligence Lab (RAIL) in Baltimore, MD. DeepCAT (Deep Computer-Aided Triage) is focused on workflow prioritization.
A deep learning tool with the ability to screen breast studies for potential abnormalities and malignancy could flag these exams as priority for interpretation. Studies have been conducted that show AI can identify such exams as accurately as a radiologist. Moy cited published studies that compared accuracy and interpretation times between radiologists and AI tools in mammography interpretation. As a group, radiologists tended to perform more accurately and efficiently with the aid of an AI interpretation tool.
AI software is being developed for each step of the imaging value chain. Radiologists may potentially leverage these products to provide more value and better patient care in their clinical practices
Linda Moy
However, the effectiveness of such AI tools ultimately depends on how well the individual radiologist can use them. In fact, one study Moy cited revealed that interpretation time and recall rate actually could increase for some radiologists. Natural language processing is being used to perform automated text mining and data analytics of a patient’s electronic health record. This capability combined with AI can help verify the appropriateness of an order, prepopulate or augment clinical information in the order entry process, and ‘harvest’ clinical information relevant to the exam. ‘An unintended consequence of computerised physician order entry is that radiologists don’t receive detailed relevant clinical history that pertains to the exam being ordered, and radiologists don’t have time to review a patient’s medical record to try to identify this information,’ Moy pointed out. ‘An AI tool could do this, and be very beneficial.’ In addition to automating protocols for high frequency, low variability orders, AI tools could also identify patients who in all likelihood will need routine spot magnification images when they are having a mammogram. An example could be patients being followed for previously identified calcifications. Moy explained that this capability could decrease interruptions related to add-on studies.
Another opportunity for AI is to improve patient exam and staff scheduling, and to identify patients most likely to be ‘no shows’ and initiate additional intervention measures. This would benefit human and imaging modality utilisation in multiple ways. Another type of targeted intervention would be to improve follow-up rates of patients having a BI-RAD 3 assessment. And rule-based AI algorithms could check for inaccuracies such as duplicate patient orders or allergy histories and create automated alerts. ‘AI software is being developed for each step of the imaging value chain. Radiologists may potentially leverage these products to provide more value and better patient care in their clinical practices,’ she concluded. (CK)
Profile:
Linda Moy is a Professor of Radiology at the NYU School of Medicine and at the NYU Center for Advanced Imaging Innovation and Research. Her area of expertise is breast MRI and AI. She is the immediate past Chair of the RSNA’s Scientific Program Committee on Breast Imaging and serves as a Deputy Editor of Breast Imaging and Senior Deputy Editor for Radiology. Moy also chairs the ACR Appropriateness Criteria and Practice Parameters on Breast Imaging, is a consultant for the Journal of Breast Imaging, and a member of the SBI Board of Directors.
01.07.2021