Article • Deep Computer-Aided Triage
DeepCAT: An AI tool to improve high volume mammography reading workflow
A new deep learning system to help radiologists improve their workflow efficiency when reading high volumes of screening mammograms is being developed at Johns Hopkins University’s Radiology Artificial Intelligence Lab (RAIL) in Baltimore, MD. DeepCAT (Deep Computer-Aided Triage) is focused on workflow prioritization.
Report: Cynthia E. Keen
Image source: ClaudiaWollesen from Pixabay
“Improvement in the radiologists workflow may be just as important as breast cancer detection,” write the software developers in the February 2021 issue of the Journal of Digital Imaging. “The topic of workflow improvement has received much less attention than breast cancer detection rates.”
DeepCAT is designed to automate prioritization of images likely to contain cancer and to discard images unlikely to contain cancer. It is a triage system that focuses on two key elements for mammogram suspicion scoring: discrete masses and other image features of cancer, such as architectural distortion.
The software has two components, a mammogram classifier cascade which uses AI to classify images as potentially normal, benign, or malignant, and a mass detector. These components are combined in a sequential workflow of image processing to generate an overall priority score. The priority score is used to order studies for review by the interpreting radiologist. DeepCAT’s components were trained using the Digital Database for Screening Mammography (DDSM), a dataset of 3,034 2D film mammography images from 2,620 patients at four U.S. hospitals.
The mass detector was trained to detect benign and malignant masses, and background breast tissue while generating a bounding box around any potential malignant lesions. In addition to classifying images, the mammogram classifier cascade was trained to maximize malignancy recall (sensitivity) and non-malignant precision (positive predictive value).
We are not looking to replace radiologists, but rather to assist with workflow and to provide a second interpretation or consultation. A combined AI system may have the potential to replace a human second reader where double reading is the norm
Lisa Mullen
Lead author Paul H. Yi, M.D., and co-researchers describe the performance and validation of their prototype software using a 595 testing image dataset in the publication. It achieved 100% precision for normal studies, classifying 53% as normal without missing a single cancer.
The authors report that they are currently training a DeepCAT system for DBT and 2D digital mammography. They also are discussing training the system to identify and classify microcalcifications. “Our work is currently focused on radiologist workflow, using AI to modify the reading worklist to prioritize cases more likely to contain a malignancy, and to potential remove normal cases from the worklist. Both of these actions would focus the radiologist’s effort toward higher priority cases, and could decrease time spent on interpretation of normal cases,” co-principal investigator and breast imager Lisa Mullen, M.D. tells Healthcare in Europe. “Combining these workflow enhancements with computer-assisted diagnostic tools would be very powerful. This combination could help the radiologist to be more efficient and may assist the radiologist with interpretation of difficult cases.” She emphasizes that “we are not looking to replace radiologists, but rather to assist with workflow and to provide a second interpretation or consultation. A combined AI system may have the potential to replace a human second reader where double reading is the norm.”
Co-principal investigator Professor Gregory Hager adds, “Personally, I think the right way to think about AI is as a second reader, potentially blinded from the radiologist. We can train algorithms to be complementary to humans -- that is, make them more sensitive to cases the human gets wrong, so that combination of human and machine has maximum value. That, combined with optimizing workload, would have the biggest impact, in my opinion.”
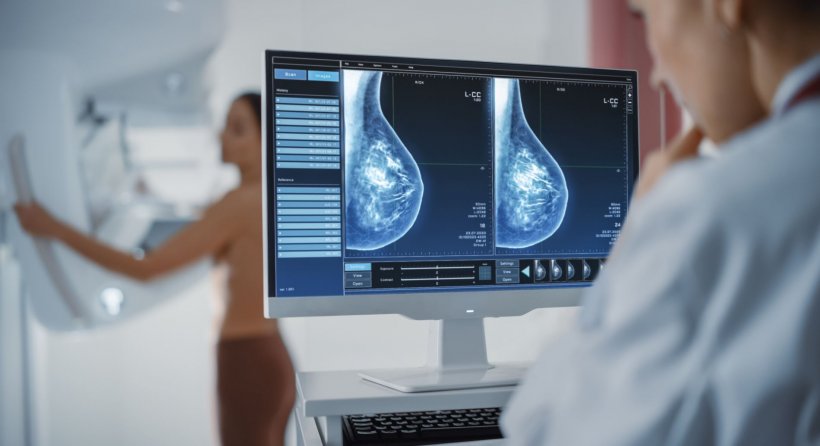
Image source: Shutterstock/Gorodenkoff
Additional training data welcome
The developers invite collaborators so that they can train DeepCAT on a dataset of images from low-resource countries, as they believe these systems could be of great benefit in countries with an inadequate number of breast imaging radiology specialists. (If interested, contact Dr. Yi at pyi10@jhmi.edu).
Profiles:
Gregory D. Hager, Ph.D., is the Mandell Bellmore Professor of Computer Science and founding director of the Malone Center for Engineering in Healthcare at Johns Hopkins University. His research interests include collaborative and vision-based robotics, time-series analysis of image data, and medical applications of image analysis and robotics. Professor Hager is a fellow of the ACM, IEEE and AAAS for his contributions to Vision-Based Robotics, and a Fellow of the MICCAI Society and of AIMBE for his contributions to imaging and his work on the analysis of surgical technical skill.
Lisa A. Mullen, M.D., is an assistant professor of radiology, is the Breast Imaging Division of the Russell H. Morgan Department of Radiology and Radiological Science at Johns Hopkins Medicine. She is also the Director of the Breast Imaging Fellowship Program. Her research interests include breast imaging practice improvement and the application of artificial intelligence to breast imaging.
Paul H. Yi, M.D., is an Instructor of Radiology and Fellow in the Musculoskeletal Imaging Division at Johns Hopkins Medicine and is the founding co-director of the Radiology Artificial Intelligence Lab (RAIL). In July 2021, he will be joining the University of Maryland Department of Radiology in Baltimore as co-director of the University of Maryland Intelligent Imaging Center.
04.03.2021