Image source: Prof Nik-Zainal
Article • Clinical computational tools
Managing cancer more effectively
Computational approaches are being applied on enormous amounts of data from sequencing technologies to develop tools to help clinicians manage cancer more effectively. Professor Serena Nik-Zainal explained that her team in Cambridge, UK, is working to further understand the physiology of mutagenesis to advance cancer diagnosis and care.
Report: Mark Nicholls
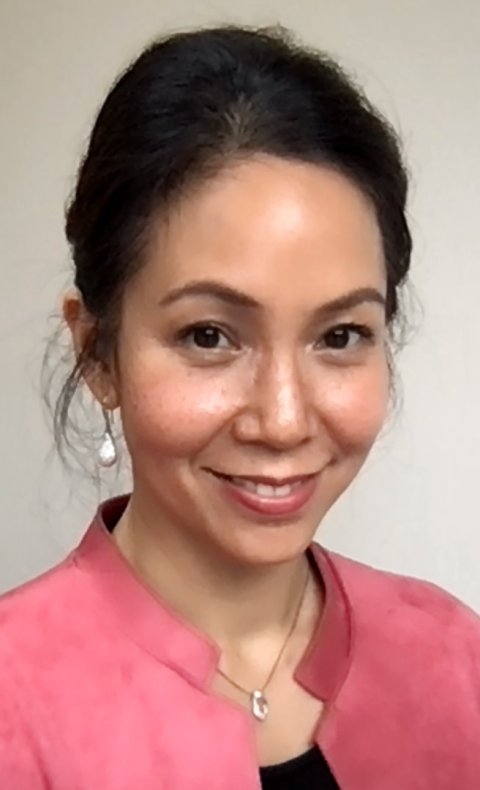
Image source: Cancer Research UK
Advances in the ability to read the human genome have made it possible to sequence the entire 3000 million base pairs of the human genome in a single experiment. ‘My team at Cambridge uses this accelerated whole genome sequencing technology to read all the mutations that are present in human cancers,’ explained Professor Nik-Zainal, who is NIHR Research Professor of Genomic Medicine and Bioinformatics and Honorary Consultant in Clinical Genetics at the University of Cambridge.
She explained that human cancers are full of mutations that serve as an historic report of anything that has gone wrong in the cells as they turned into cancer cells. ‘If we can truly understand what those mutations signify, then we come closer to understanding the causes of each person’s cancer, and how to inform clinical decision-making for individual patients,’ she added.
With computational methods critical in studying the large mutation datasets, she explained that the two main groups of mutations to try to understand are:
- the small handful of “driver” or causally-implicated mutations, which tend to happen in the body of genes, because these driver mutations can become targets for therapeutic intervention; and
- the large swathe of mutations that form patterns across the genome, which are called mutational signatures.
‘Both of these types of mutations, drivers and mutational signatures, are important, because they help us to understand cancer development,’ the expert said.
Her team is particularly interested in mutational signatures and performs laboratory experiments to understand how and why those mutational signatures arise. ‘Some of these signatures are like a signal, a biomarker, of a biological pathway that is awry, and sometimes those can be targetable,’ she continued. ‘For example, we can discern patterns of mutations that are due to DNA repair defects and drugs like immunotherapies can be used to target tumours with specific DNA repair defects.’
Tools to manage the “data deluge”
We hope that our tools will facilitate clinicians in a future where cancer genomics is a routine part of cancer care
Serena Nik-Zainal
In order to derive maximum understanding of mutagenesis, her team combines experimental work with computational approaches. ‘Ultimately, we want to use the new knowledge from the experimental data and big cancer datasets to develop clinical computational tools that can help us to manage cancer more effectively.’ From there, the team applies statistical methods – such as machine-learning models – to create algorithmic tools. ‘The purpose of these tools is to enable clinicians to quickly identify patients that may have sensitivities to particular drugs or to help inform management in some way,’ added Professor Nik-Zainal. ‘We hope that our tools will facilitate clinicians in a future where cancer genomics is a routine part of cancer care.’
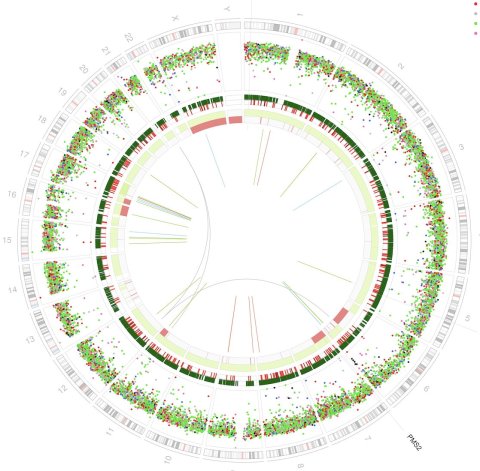
Image source: Prof Nik-Zainal
Given the current “deluge of data”, there are few easy ways to analyse and interpret it, so her team is looking to create apps to support clinicians in cancer genome interpretation. One example for this is an algorithm developed through machine-learning called HRDetect to identify tumours that have an abnormality in the BRCA1/BRCA2-dependent DNA repair pathway based on their mutational signatures.
Creating the algorithm, however, was simply the first step, and subsequently the team had to demonstrate that HRDetect could distinguish breast cancers with good from bad clinical outcomes. This saw collaboration with colleagues in Sweden, and later with those in London and with pharmaceutical company Clovis to show that HRDetect was predictive of sensitivity to a drug. ‘We collaborated to show that our algorithm did have early predictive capabilities and that breast cancers with high HRDetect scores showed activity to treatment with a PARP-inhibitor drug,’ said Professor Nik-Zainal.
Preparing for a data-driven future
The next step is a Phase III trial with Genomics England – the national whole genome sequencing endeavour – to see whether their clinical algorithmic tools can be implemented into the NHS bioinformatics pipeline. ‘The next stage of our work is to get our clinical algorithms to patients as soon as possible and get to a Phase III randomised controlled trial to show that our algorithms really help inform patient care,’ she said. ‘Ultimately, genomics will become another tool in the everyday management of cancer. Beyond creating individual algorithms for genomic interpretation, there is a large piece of work involved in creating holistic clinical decision support tools and educating the next generation on how to use these tools so that they are equipped for a data-driven future.’
Profile:
Professor Serena Nik-Zainal is Professor of Genomic Medicine and Bioinformatics and an NIHR Research Professor at the University of Cambridge, UK. She is also an Honorary Consultant in Clinical Genetics at Cambridge University Hospitals NHS Foundation Trust. Having been heavily involved in the development of the whole genome sequencing (WGS) using next-generation sequencing (NGS) technology, she now leads the Genomic Medicine theme at the NIHR Cambridge Biomedical Research Campus.
05.05.2022