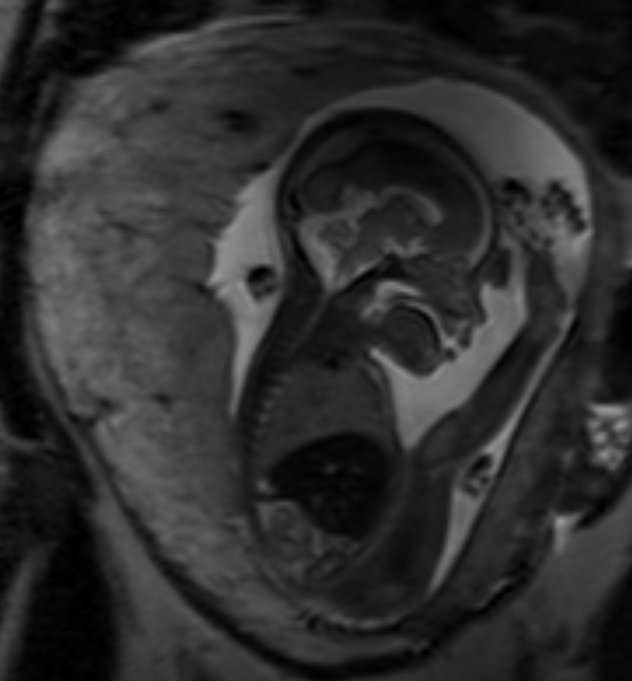
Article • Pregnancy imaging
Machine learning predicts placenta health from MRI scans
Machine learning methods are being used to predict the health of the placenta from a 30-second MRI scan. Researchers hope the approach will offer an insight into the health of expectant mothers and unborn babies by detecting the early signs of dangerous conditions such as pre-eclampsia.
Report: Mark Nicholls
Researchers from the School of Biomedical Engineering & Imaging Sciences at King’s College London (KCL) have been working jointly with the women’s health department at the city’s St Thomas’ Hospital on the study, which may also be able to highlight other conditions associated with pregnancy.
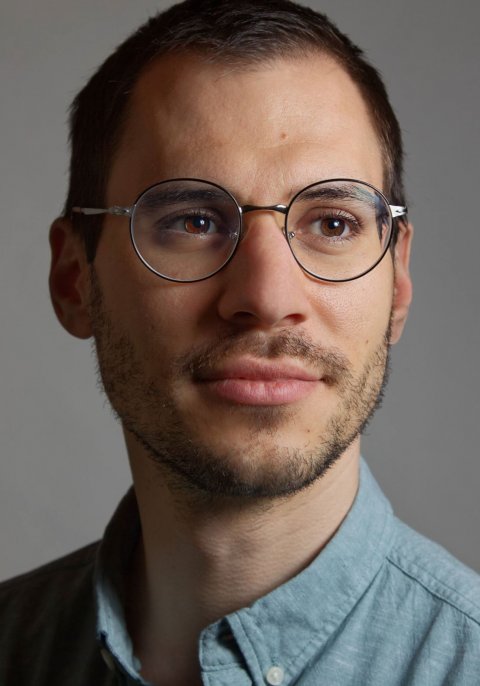
From routine MRI scans, a machine learning algorithm models the normal distribution of placental tissue properties which can be used to screen for deviations from normal placental ageing and signs of pregnancy complications. Patients are scanned with a T2* sequence MRI with the imaging data fed into the neural network pipeline which rapidly performs automatic segmentation before a regression model delivers a placental health score. The scan, combined with the automatic pipeline, can be included as part of any fetal MRI examination but has the benefit of acquiring additional information not available under routine obstetric screening using ultrasound.
Lead researcher Dr Maximilian Pietsch said the method is most sensitive early in the second half of pregnancy which is also the typical time of onset of pre-eclampsia, allowing early detection and monitoring of this complication. The method develops a clinical marker and automates its extraction which would otherwise be prohibitively time-consuming in clinical practice.
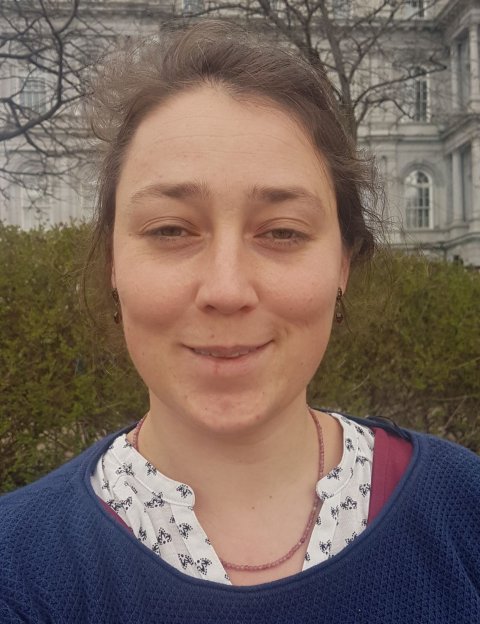
Research Fellow Dr Jana Hutter said the approach has the potential to provide additional information to ultrasound, which cannot acquire information from inside the placenta. “We use MRI as it has no ionizing radiation, so is completely safe to use in pregnancy, and the T2* imaging technique allows us to look at oxygenation in the placenta,” she added.
With chronic hypertension in pregnancy becoming increasingly common, there is a raised chance of women developing pre-eclampsia. “This technique allows us to look for this before any clinical symptoms start in this high-risk population,” said Dr Hutter. “This gives obstetricians additional valuable information to closely monitor the mother and baby to optimize the time point of the birth - late enough for the baby but early enough to avoid any effects to the mother.”
The technique has the potential to assess the health of the unborn child and mother introduce interventions to prevent possible complications from getting more serious. Other applications could be to look for evidence of fetal birth restriction, where the baby does not grow as big as it could; late stillbirth; and other complications like gestational diabetes.
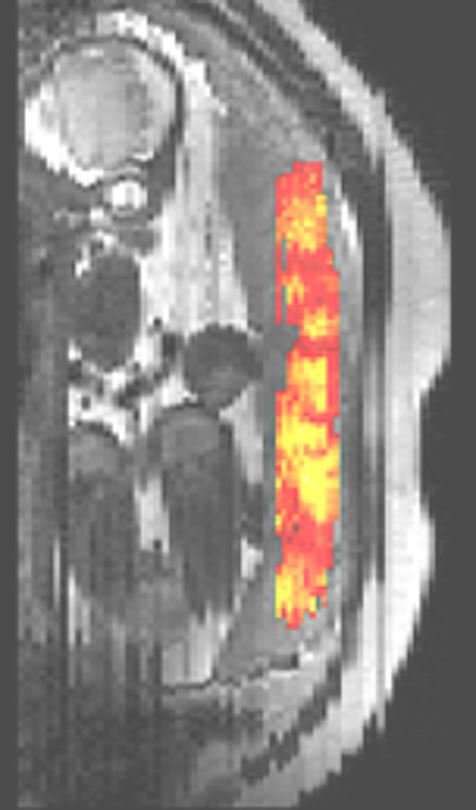
Dr Pietsch said the technique was devised via a multi-disciplinary approach in a hospital setting, working in close collaboration with obstetricians, physicists, radiologists, midwives, engineers and pathologists.
A next step is to recruit targeted groups to test the machine learning algorithm for other conditions and add technical improvements. But he emphasised: “This solves a clinical need in a way that is feasible to achieve in standard clinical care. Time requirement is drastically reduced to obtain the information, which before would have to be manually segmented and extract this information. “This is a measure that seems to be very useful in determining the health of the placenta. The data flow is quite simple and the results are immediately intuitively interpretable.” He said that whereas a lot of medical machine learning research is somewhat detached from clinical needs, this has a strong immediate application in a hospital setting.
Profiles:
Dr Maximilian Pietsch is a Research Associate in KCL’s Institute of Psychiatry, Psychology, and Neuroscience (IoPPN) and conducts research in a clinical setting at St Thomas’s Hospital. His research interests involve using machine learning in combination with advanced diffusion MRI data to study the developing brain.
Dr Jana Hutter is a Senior Research Fellow at KCL and UKRI Future Leaders Fellow based at St Thomas’s Hospital. After finishing her PhD in Erlangen, Germany, she moved to KCL where she now leads her own research focusing on the topic of functional fetal and neonatal MR.
30.09.2021