News • AI & Deep Learning
How to escape from data silos
Artificial Intelligence (AI) and machine learning are poised to transform healthcare, potentially freeing practitioners across many disciplines from routine tasks and saving lives through efficient early detection.
Source: Pixabay/PublicDomainPictures
Offering insight into the health of both individuals and populations, these ’deep learning‘ algorithms have the potential to process vast amounts of data and identify warning signs, tumours and other medical issues at an early stage. Provided, of course, that they have access to the right data.
In a recent issue of European Hospital, Hologic Vice President Tracy Accardi addressed the huge potential for AI and deep learning algorithms to help radiologists, for example, by searching tomosynthesis data for signs of malignant tumours. However, she added: “With deep learning, you have to make very sure you’re asking the algorithm the right questions, otherwise you don’t get the right answers.”
Recommended article
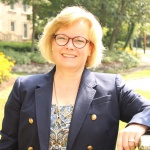
Sponsored • Machine Learning
Finding the right algorithms to tackle big data
Tracy Accardi, Hologic’s Vice President (Global R&D), spoke of the importance of innovation, tomosynthesis, artificial intelligence/deep learning and open dialogue with the radiology community. Hologic addresses a broad spectrum of gynaecological, perinatal, aesthetic, skeletal and breast women’s health issues. To enhance this approach, Accardi, explained the importance of working closely…
That will be a challenge for those designing the AI systems set to help clinicians. The answers these machine learning applications provide will also depend on the data they can access, and if that is limited to just one department, then the efficacy of the whole process will fall short.
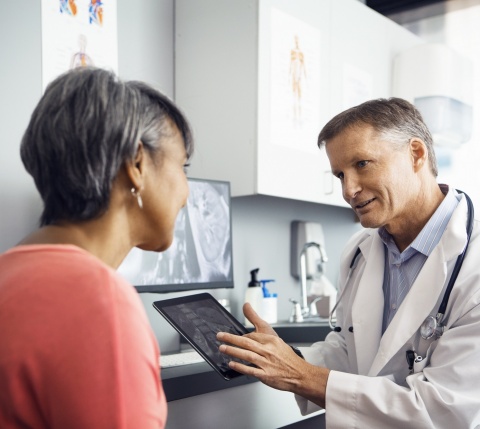
Obviously, AI, machine/deep learning, analytics and population health tools are still in the very early stages of adoption. However, whether you’re feeding these applications with data for training purposes or to yield actual results to drive outcome improvements, the datasets leveraged must be as comprehensive as possible. Furthermore, the healthcare organisation must have full ownership and control of the data being processed. Applying partial or inaccurate datasets to AI and machine learning will yield less than optimal results and the unfortunate truth is the clinical data that resides in most health systems today is woefully incomplete and disjointed.
The problem is that even in hospitals with the most advanced systems, many departments are still grappling with a mixture of paper records and proprietary medical image and health data storage systems. All of this information, which isn’t available in enterprise-wide core clinical systems, is effectively trapped in ’silos’ which can’t be accessed by an algorithm or AI programme. These data silos are a significant barrier to progress, not only in the area of content management, but also AI and machine learning — all of which are vital to delivering patient-centred care that improves clinical decision making and outcomes.
The key is to start with the areas that offer the best potential for immediate clinical benefits and build on the platform from there
Perhaps the single biggest source of disconnected and siloed clinical content that needs addressed is medical images. The PACS (Picture Archiving and Communications Systems) images used by most healthcare organisations today are designed to manage large volumes of medical images based on the DICOM standard (e.g. MRIs, CT scans, X-rays, etc). These systems have served radiology and cardiology departments well for the better part of two decades and, until recent years, have encompassed the vast majority of imaging assets within a healthcare organisation. The problem with a PACS-only strategy in today’s healthcare environment is the growing number of images that aren’t based on the DICOM standard. These assets include surgical video, endoscopy, dermatology photos, wound images, pathology studies and more, whose native formats range from JPEGs to TIFFs to MPEG-4s.
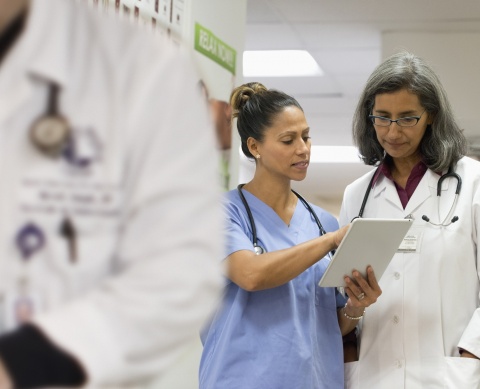
Creating a comprehensive, longitudinal medical image history for a patient requires consolidating and centralizing all of these image types and studies using an enterprise imaging strategy. This approach consists not only of PACS, but includes a mix of VNA (Vendor Neutral Archive), enterprise viewing and image connectivity solutions as well. The goal should be to standardise the medical imaging data, removing the proprietary code sets that trap these assets in specific systems and allow them to be securely (but freely) accessed by clinicians throughout the enterprise — when and where they are needed. Beyond medical images, healthcare organisations should also seek to identify other primary sources of “dark” or “rouge” data. Most often this siloed data is unstructured (i.e. data that is not organised in a pre-defined manner). This information can include clinical documents, case notes, emails, faxes and photos that may be stored in paper format or electronically in any number of ECM (Enterprise Content Management) systems. The goal should be to digitise and standardise as much of this information as possible and integrate it with the structured data stored in core clinical systems as well as all medical images.
Creating an enterprise platform for managing unstructured and semi-structured information, such as medical images, isn’t easy. On the contrary, it will likely be a long journey that requires several departments and repositories to be integrated incrementally. The key is to start with the areas that offer the best potential for immediate clinical benefits and build on the platform from there. The platform solution sought should have modern APIs based on standards and interoperability specifications like DICOMweb and XDS, as well as powerful de-identification functionality. Since machine and deep learning applications are always starved for accurate data and require large data sets, the platform shall be capable of producing very large training batches in a scalable way and without impacting the performance and availability of other production systems.
If done correctly, a comprehensive platform that includes both unstructured and structured information in the context of the patient will do more than feed AI applications with rich, accurate patient data. It will also improve continuity of care, reduce administrative costs and improve data security.
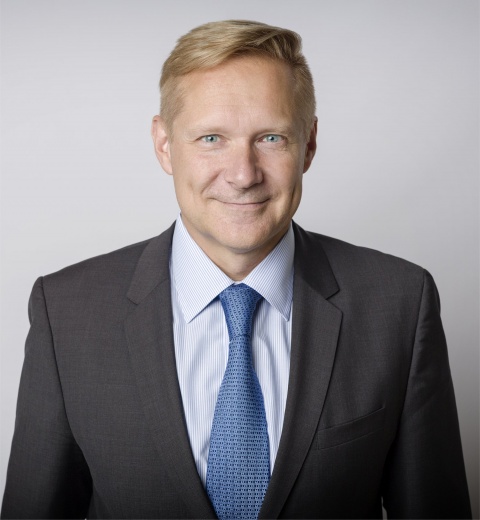
About the Author:
Razvan Atanasiu is Chief Technology Officer, Enterprise Imaging, Hyland Healthcare. An accomplished leader in healthcare IT, with over 20 years of R&D and management experience, Raz is a recognised authority in enterprise imaging and vendor neutral archive (VNA) architectures. He is highly regarded for his leadership, commitment to interoperability and standards, technical excellence, and expertise in post-merger software product integration. Raz is responsible for the delivery and future direction of Hyland Healthcare’s medical imaging portfolio, including Acuo VNA, NilRead Enterprise Viewer and PACSGEAR suite. Prior to Hyland, Raz held executive positions with Lexmark Healthcare, Acuo Technologies and Emageon. Raz has an academic background and was an early researcher in the areas of DICOM Web Services and Grid Technologies.
04.07.2018