Sponsored • Machine Learning
Finding the right algorithms to tackle big data
Tracy Accardi, Hologic’s Vice President (Global R&D), spoke of the importance of innovation, tomosynthesis, artificial intelligence/deep learning and open dialogue with the radiology community.
Interview: Daniela Zimmermann
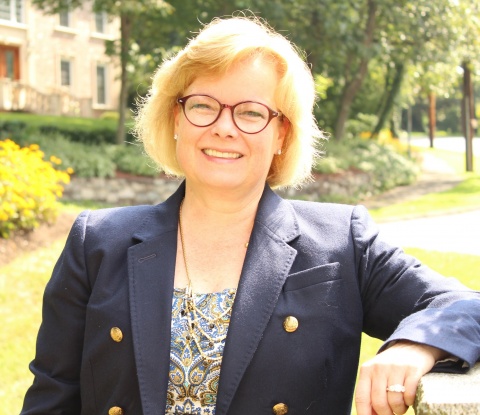
Hologic addresses a broad spectrum of gynaecological, perinatal, aesthetic, skeletal and breast women’s health issues. To enhance this approach, Accardi explained the importance of working closely with radiologists. ‘We are seeing the benefits of the significant level of investment we have been making over the last several years in bringing new products to the market. Within that, everything we do is around improving workflow for the radiologist and the technologist – and improving the experience for the patient. When you think about tomosynthesis, for example, everybody raises concern about the time it takes to read an image. We constantly work on image quality and continue to develop a detection process that takes in more information from a pixel perspective, but does not increase the time it takes to do the scan or read the image. This turns out to be a win for both the patient and the radiologist. When we talk about a focus on workflow improvements, we need to get smarter with how to help radiologists so they are comfortable that they have looked at everything they need to look at with tomosynthesis and are not overwhelmed by the amount of data. We have more than three years invested into looking at how to use deep learning to read the tomosynthesis data and direct radiologists to the things that they should be most concerned about.’
How could deep learning, artificial intelligence and big data work?
‘We make continuous investments to ensure we have broad mammography data sets representing full variations of density and diagnostic outcome. To make deep learning really work, the more data you have, the smarter your algorithms are, so we’re very focused on case collection. We know we will need thousands of known cancers with pathologically confirmed outcomes so that we can teach the algorithm how to find those occurrences in the data. We have developed the ability to grade how well our algorithms are working. I think our competitive edge is that we have developed a lot of knowledge over time about how to interpret mammography images, and have invested in learning how radiologists think and what they need to do deliver the best standard of care. We know that we need to look at all the ways that breast cancer presents itself. We want our algorithms to tell the difference between a mass that the radiologist should worry about and one that can be dismissed.’
Other than wait and see, how do you safely distinguish slow growing masses?
‘There are indications of a fast growing or aggressive cancer, for example the presence of spiculations or unusual vascularization in the image. If a mass is perfectly round and smooth walled it is most likely non-malignant and you can train your algorithms to know the difference. The more three-dimensional your data is – and tomosynthesis is a perfect example – the better your algorithm can be. The radiologist needs to be able to focus on the observations where the algorithm cannot be positive.’
Might deep learning replace the radiologist?
With deep learning, you have to make very sure you are asking the algorithm the right questions, otherwise you don’t get the right answers
Tracy C Accardi
‘From a deep learning perspective, the space was held back for a long time given available tools and limited processing capability. Developments over the last several years have delivered significant improvement at reasonable cost in both areas. I believe we’ll see significant industry investment in delivering the promise of deep learning to the radiologist, and I’m very excited about that. What I’m fascinated about is that, with deep learning, you have to make very sure you are asking the algorithm the right questions, otherwise you don’t get the right answers. It sounds very simple, but you can otherwise fool yourself about what you think the algorithm is telling you. We have invested a lot of time speaking with radiologists around the world about how they want to see deep learning help them with questions that they want to try to answer. Deep learning is not about replacing the radiologist, it’s about making radiologists as efficient and positive as they can be about their diagnoses, and perhaps not having to look at 40-50% of the images that are not going to be a problem. Giving the radiologists the ability to confidently focus on just the cases that are flagged with issues is the primary goal.’
Do you see more adopters, or will process be slow?
‘While capabilities of deep learning are being felt all around us in our daily lives – I anticipate that adoption in the interpretation of mammography will be relatively slow because the radiologist, quite rightly, needs to be extremely confident. At Hologic, we are completely committed to delivering solutions that gain that level of confidence.’
What is the next step?
‘Our focus is on continuously amassing fully validated tomosynthesis images, consulting with radiologists and training our algorithms to establish clinical support and comprehensive claims. We will build toward our future with deep learning. I firmly believe that deep learning is critical to globally transform breast patient care through insight-driven, innovative solutions.’
Profile:
In 2014 Tracy C Accardi MSc (MEng) joined Hologic as Vice President of Global Research and Development at the Breast and Skeletal Health Division, where she leads a globally diverse team of 220 engineers and scientists, to develop systems for 2-D and 3-D mammography, breast diagnosis/biopsy, and bone densitometry. Prior to this appointment, she was Chief Technology Officer at OmniGuide Surgical for two years, leading development and marketing of advanced surgical energy solutions for minimally invasive surgery. Earlier roles include those at MedNest Consulting, Coviedien, Codman & Shurtleff (a division of Johnson & Johnson), Philips Healthcare and General Electric (GE). Among other commitments, Tracy Accardi is on the board of ‘A Better Chance’, a non-profit organisation in New York City committed to the education of academically talented, promising young women from under-served communities.
07.05.2018
- AI (722)
- big data (102)
- breast cancer (586)
- gynaecology (75)
- machine learning (163)
- manufacturers (207)
- medical technology (1501)
- musculoskeletal (114)
- obstetrics (131)
- radiology (696)