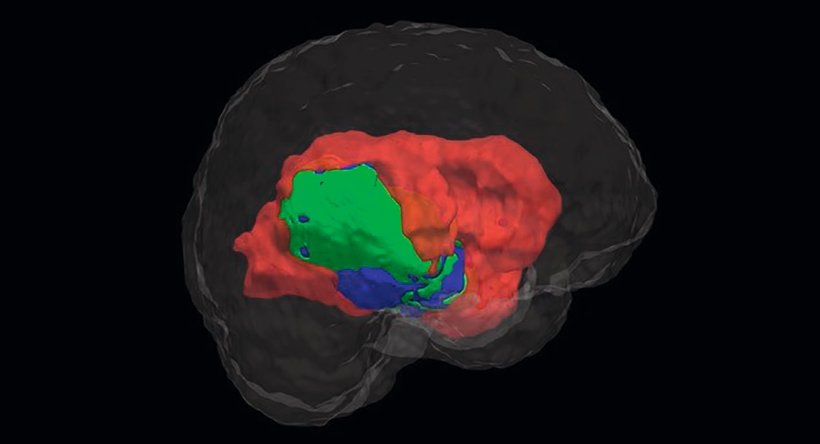
© University of Lübeck
Article • AI-assisted image analysis
More accuracy in brain tumour segmentation
Diagnosing cancer and managing a patient’s respective treatment path requires a precise segmentation of the affected anatomical structures. Defining the different semantic objects in an image such as disease patterns, lesions, biomarkers, organs, tissues etc. is at the core of this. Such a segmentation enables radiologists to distinguish the three subcategories of a tumour – the active core, the necrotic disintegrated area and edemas – among others. A range of medical decisions are based on this classification: e.g., radiologists determine the volume of a tumour, monitor its development, design the concept of a personalised radiation therapy and subsequently administer it. In the realm of surgery, image segmentation is used for planning and navigating operations.
Article: Cornelia Wels-Maug
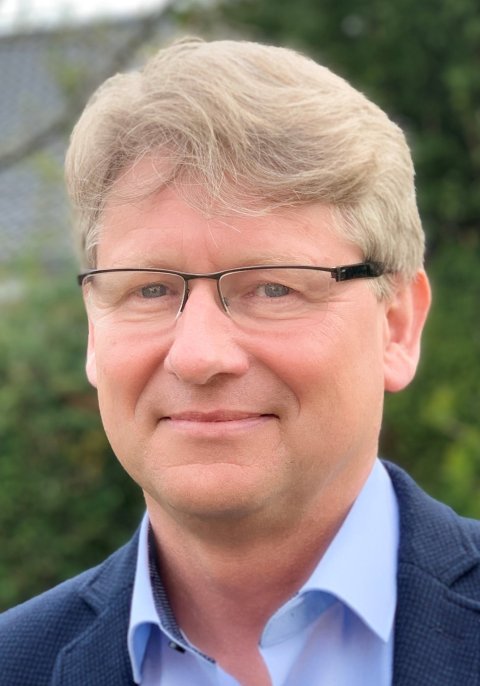
Image source: DKFI
To best support patients, clinicians need this segmentation to be as accurate as possible. However, segmenting medical images is a key challenge of medical image processing. The Research Department Artificial Intelligence in Medical Image and Signal Processing (AIMedI) at the Lübeck site of the German Research Center for Artificial Intelligence (Deutsches Forschungszentrum für Künstliche Intelligenz or DFKI) has been working on the development of a high-precision three-dimensional viewer software for medical image data. Using AI and deep learning methods, the software analyses medical image data, bio signals along with other patient data and automatically interprets them. In this way, it can delineate pathologically altered image areas and detect diseases.
Prof Dr Heinz Handels, Director of the Institute of Medical Informatics, University of Lübeck, and Head of AIMedI explains: “Our aim is to support clinicians with the classification of cancer tissue of malignant brain tumours such as glioblastoma. This also helps with the volumetric measurement of a brain tumour for which we need to be able to define which pixels form an object.”
Annotated medical data in short supply
Methods to measure the volume of tumours already exist, but their accuracy is still wanting. To this end, the research group around Handels, AIMedI, developed a procedure that allows to automatically segment and recognise objects such as tumours at an exact pixel-level in the brain. It uses deep learning methods tailored to image processing, which deliver a higher accuracy of the segmentation. These deep learning methods autonomously learn from training data. To facilitate this, the algorithm requires original images that must be manually marked by an expert to create a so-called annotated image.
This approach has already been successfully used in many areas of public life, such as for the analysis of traffic, where non-specialists perform the annotation of the images. Nevertheless, this is different in the field of medicine where an expert is required to do the annotation. As it takes several hours to annotate a 3D dataset, only few exist: “I reckon that in my research community there is globally only a limited number of such annotated datasets, which are all part of a global initiative. The data is held on central servers so that the images can be accessed from everywhere. This allows to compare generated images to those annotated by experts”, says Handels.
However, deep learning algorithms deliver more reliable results, the more data is available.
Using U-Nets for optimised results
In our research, we investigate to use only synthetic data for the training of neural networks. This will be of enormous value for research purposes as this data will be freely available
Heinz Handels
To overcome the constraints of limited manually annotated training data, scientists in the AIMedI group have trained a U-Net, a convolutional neural network developed for image segmentation. The U-Net architecture is suited to work with fewer (annotated) training images whilst achieving a more precise segmentation. Furthermore, the researchers investigate the safety and possible explanations of the generated results.
The evaluation of the methods and systems developed is still ongoing and done in close cooperation with medical co-operation partners based on practical applications.
The future: Generating annotated synthetic image data
At the heart of this endeavour is the aspiration to generate annotated synthetic brain tumor images to free up experts’ time, which they anyhow only unwillingly bestow on annotating 3D datasets. Handels explains: “We aim to generate new data, so-called synthetic image data, where the labeled data is automatically generated, using generated adversarial networks (GANs) or diffusion models.” However, this type of network poses its own challenges like its substantial storage requirements.
Furthermore, synthetic data would circumnavigate data protection requirements as mandated per the General Data Protection Regulation. “In our research, we investigate to use only synthetic data for the training of neural networks. This will be of enormous value for research purposes as this data will be freely available”, concludes Handels. This is a relatively new research field, and more work is needed.
05.12.2024