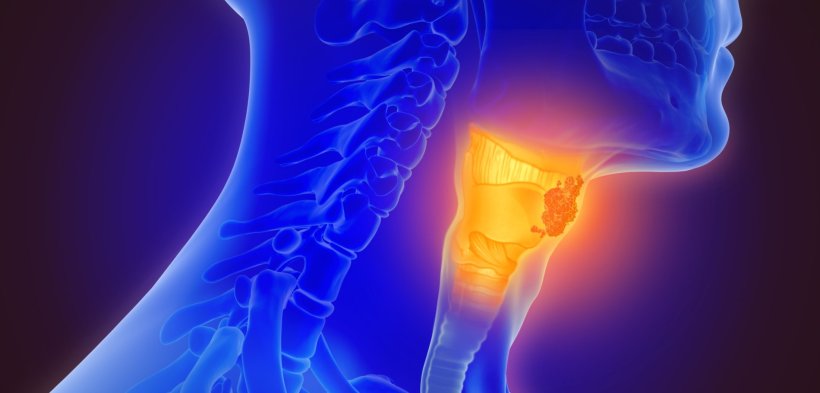
Image source: Adobe Stock/Lars Neumann
Article • Multimodal analysis approach
Novel AI model predicts thyroid cancer from routine ultrasound images
An artificial intelligence (AI) model combining four methods of machine learning (ML) to accurately detect thyroid cancer from routine ultrasound image data has been developed by researchers at Massachusetts General Hospital (MGH) Cancer Center in Boston, US.
Report: Cynthia E. Keen
The model’s ability to predict pathological and genomic outcomes could reduce unnecessary biopsies of suspicious thyroid tumours and become a low-cost, non-invasive option for the screening, staging and personalised treatment planning, Annie W. Chan, MD, told attendees at a plenary scientific session of the 2022 ASTRO/ASCO Multidisciplinary Head and Neck Cancers Symposium held recently in Phoenix, Arizona.
Detection rates of thyroid cancer are on the rise, due to increased availability and technical improvement of ultrasound imaging. Still, the prevalence of thyroid cancers differs significantly among countries. The World Health Organization (WHO) International Agency for Research on Cancer estimates that 586,200 new cases were diagnosed in 2020 – the majority of which in Asia (59.7%), followed by Europe (14,9%), Latin America and the Caribbean (10.8%), and North America (10.6%). ‘Ultrasound is the gold standard to detect and diagnose malignant thyroid tumours,’ said Dr Chan. ‘But radiologists make their diagnoses completely based on perception. They analyse the appearance and shape of nodules. Are they stable, suspicious, worrisome, or concerning? Are they uniformly or heterogenous enhancing? Are they spiculated, infiltrative, or patchy in shape? They manually measure the size. All analysis of ultrasound images is subjective.’
Radiomics, TDA, TI-RADS and deep learning: a diagnostic dream team
Digital technologies could establish more objective criteria to the notoriously user-dependent modality: ‘Digital images can be quantitatively assessed by computers as well as by humans. They are recorded as many numbers, with an image divided into a matrix of pixels, and each pixel represented by a numerical value that can be processed by computers. Humans perceive texture in terms of smoothness, roughness, or bumpiness. In radiomics, texture is a variation of grey-level intensity across an image. Radiomics quantifies how many pixels have similar characteristics and how they are related to each other, and by doing so, radiomics can precisely quantify shape and texture,’ she said.
Dr Chan, director of the MGH Head and Neck Radiation Oncology Research Program, explained that the multidisciplinary team believed that a multimodal AI ultrasound analysis could combine the strengths of distinct AI methods in a complimentary manner that would outperform each individual method. Her team developed and validated an AI ultrasound platform that consists of radiomics, topological data analysis (TDA), Thyroid Imaging Reporting and Data System (TI-RADS) features, and deep learning to predict malignancy and pathological outcome.
Radiomics extracts a large number of quantitative features from the images and represents the local structure of data. TDA analysis assesses the spatial relationship between data points in the images, representing the global structure of data. Deep learning utilises algorithms that run data through multiple layers of an AI neural network to generate predictions. A machine learning (ML) algorithm utilises TI-RADS-defined ultrasound properties as machine learning features.
Potential for staging without the need for surgery
By integrating different AI methods, we were able to capture more data while minimising noise. This allows us to achieve a high level of accuracy in making predictions
Annie W. Chan
Ultrasound exams from 784 patients containing 1,346 thyroid nodule images were used to train and validate the model. These were divided into training and validation data sets. The researchers confirmed malignancies with samples obtained from fine needle biopsy. Pathological staging and mutation status were confirmed from pathology reports and genomic sequencing, respectively. ‘By integrating different AI methods, we were able to capture more data while minimising noise. This allows us to achieve a high level of accuracy in making predictions,’ said Dr Chan. In fact, this model accurately predicted 98.7% of thyroid nodule malignancies, compared to 89% by the radiomics-only model, 87% by the deep learning-only model, 81% by the TDA-only model, and 80% on the machine learning TI-RADS model.
Additionally, a multi-model model comprising radiomics, TDA, and (ML) TI-RADS was able to distinguish the tumour stage, the nodal stage, and the presence or absence of BRAF mutation of malignant tumours. ‘This capability is especially beneficial because it is not possible for a radiologist to stage a detected thyroid cancer from ultrasound images or other existing imaging modalities. Thyroid cancer can only be staged surgically. If we can predict staging without having surgery, surgical planning can be appropriately done. For instance, Stage I tumours can be observed without any surgery, as they usually remain indolent,’ explained Dr Chan. ‘Our model needs additional testing on much larger data sets representing diverse populations’, she added. ‘We are encouraged by its high level of accuracy, predictability, robustness, and reproducibility. The model uses routine ultrasound images and could greatly aid radiologists in making diagnoses and expediting their thyroid cancer screening workflow.’
Profile:
Annie W. Chan, MD, PhD, is an associate professor of radiation oncology at Harvard Medical School. She also is the director of the Head and Neck Radiation Oncology Research Program at Massachusetts General Hospital Cancer Center in Boston. Her translational research is to develop novel imaging technologies to improve cancer diagnosis and outcomes. She conducts clinical research on proton beam dose distribution to improve clinical outcomes and decrease treatment-related morbidity in patients with head and neck and skull base cancers.
16.06.2022