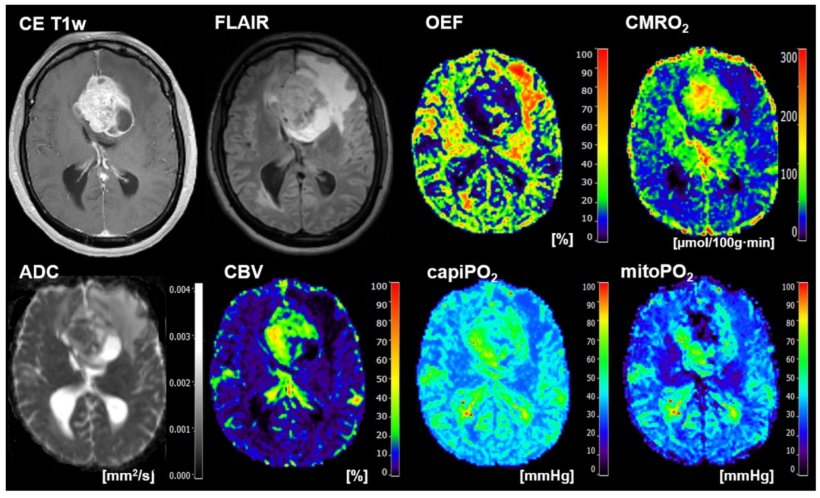
Image source: Stadlbauer et al., Metabolites 2022 (CC BY 4.0)
News • Primary tumor or metastasis?
Deep learning and radiomics for precise differentiation in brain tumors
The distinction between primary tumors and metastases can be made quickly and accurately in brain tumors using radiomics and deep learning algorithms. This is the key message of a study from Karl Landsteiner University of Health Sciences (KL Krems).
The study, which was now published in Metabolites, shows that magnetic resonance-based radiological data of tumor O2 metabolism provide an excellent basis for discrimination using neural networks. This combination of so-called "oxygen metabolic radiomics" with analyses by special artificial intelligence was clearly superior to evaluations by human experts in all essential criteria. This is all the more impressive because essential oxygen values did not differ significantly between tumor types – and neuronal networks were nevertheless able to make clear distinctions on the basis of these values.
Glioblastoma, a primary tumor, and brain metastases are the most common types of brain tumors in adults. Their treatment must be fundamentally different, and a rapid and clear diagnosis therefore influences clinical outcome. However, their differentiation is difficult, as they are hardly distinguishable on classical magnetic resonance (MR) images. This is different with so-called physio-metabolic MR, which can record metabolic processes in tumor tissue. However, this provides such large amounts of data that its use in routine diagnostics would require evaluations by artificial intelligence. Their reliability is now being demonstrated by a team led by Prof. Andreas Stadlbauer of KL Krems using a specially developed deep learning algorithm and MR-based data on the O2 metabolism of the two tumor types.
"In fact, our approach succeeded in achieving better distinctions between the tumor types than human experts were able to achieve in comparison," said Prof. Stadlbauer, summarizing the results of the international study. The medical physicist at the Central Institute for Medical Radiology Diagnostics at St. Pölten University Hospital, teaching and research site of KL Krems continues: "In all important differentiation criteria such as accuracy, sensitivity, specificity and precision, the evaluation of MR-based oxygen data by our special neural network was superior to radiologists. This method was also better than the human evaluations in statistical evaluations such as the F-values and the AUROC."
Interestingly, neither the mean nor the median of these two parameters differed significantly between the two tumor types – but nevertheless, our CNN managed to clearly differentiate the two tumor types
Andreas Stadlbauer
The measurements were based on a so-called "Convolutional Neural Network" (CNN) specially developed by the team. This is a special form of artificial neural network that is designed for machine learning and the processing of image or audio data and mimics parts of biological processes. As part of the study, the CNN was then trained using tumor data from the extensive database at St. Pölten University Hospital and subsequently used to analyze MR-based oxygen levels of new patients.
The oxygen values collected during the study included cerebral oxygen turnover (CMRO2) and mitochondrial oxygen saturation (mitoPO2), which provides information about cellular energy turnover. "Interestingly," said Prof. Stadlbauer, "neither the mean nor the median of these two parameters differed significantly between the two tumor types – but nevertheless, our CNN managed to clearly differentiate the two tumor types."
The study shows the great diagnostic potential of combining the two methods. In fact, however, radiological data of O2 metabolism are still only used to a very limited extent in everyday clinical practice. Prof. Stadlbauer and his team would like to change this and are therefore already planning a more extensive study that will not only confirm the data now collected, but will also use methods that are even closer to clinical routine. Prof. Stadlbauer explains: "In the current study, some manual steps were still necessary to prepare the data analysis. This is too time-consuming for clinical routine and also limits comparability between different institutions. We therefore plan to use CNN in this phase as well."
Source: Karl Landsteiner University of Health Sciences
22.01.2023