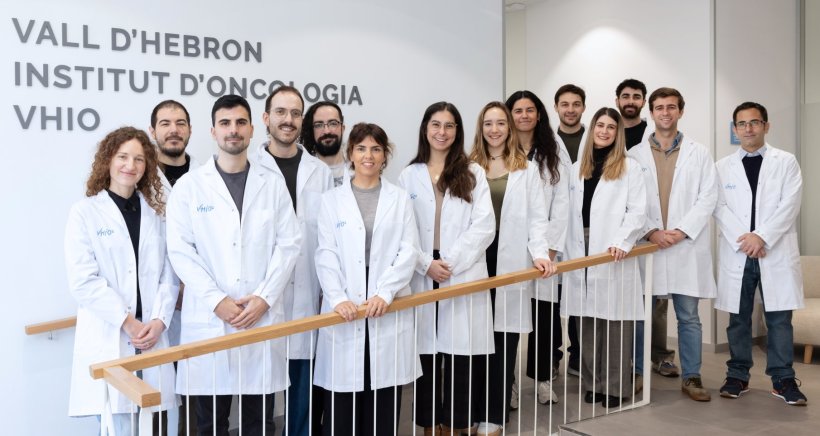
Image source: VHIO
News • CT-based deep learning-driven tool
Liver cancer segmentation and detection with 'SALSA'
Investigators of the Vall d’Hebron Institute of Oncology’s (VHIO) Radiomics Group, led by Raquel Perez-Lopez, have developed SALSA (System for Automatic Liver tumor Segmentation And detection), a fully automated deep learning-driven tool for the precise and completely automated detection and monitoring of liver tumors (hepatocellular carcinoma).
Results of this work have been published as an open access article in Cell Reports Medicine.
Medical images including computed tomography (CT) scans, provide professionals in oncology with essential information for cancer detection, treatment planning, and response evaluation. “However, the precise delineation of tumors for volume analysis—tumor contouring—poses practical challenges and often causes a bottleneck in research projects and clinical applications involving volumetric disease assessment. This task is not only time consuming but also prone to variability between and among different observers,” said Raquel Perez-Lopez, corresponding author of the paper.
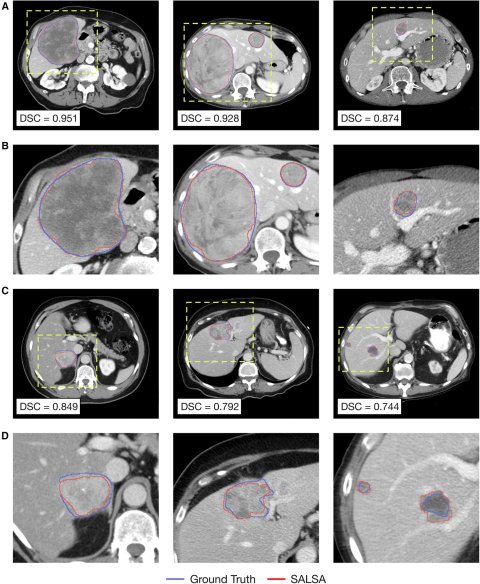
Image source: Balaguer-Montero M, Marcos Morales A, Ligero M et al., Cell Reports Medicine 2025 (CC BY-NC-ND 4.0)
Primary liver cancers such as hepatocellular carcinoma (HCC) are often diagnosed in advanced stages, with limited treatment options available and an unfavorable prognosis. Furthermore, the liver is a common organ for metastases originating from other primary cancer, significantly impacting treatment outcomes. Aimed at addressing these clinical challenges, VHIO investigators have developed SALSA, an AI-aided tool that works directly on medical images, automatically detecting and delineating liver tumors, both primary and metastatic. “For the development and training of SALSA, we used the existing nnU-Net segmentation method and introduced data obtained from 1598 computed tomography scans of 4908 primary or metastatic liver tumors,” explained Maria Balaguer-Montero, a PhD Student of VHIO’s Radiomics Group and first author of the study.
Showing superior accuracy for cancer detection and precise quantification of tumor burden, surpassing state-of-the-art models and the radiologists’ inter-agreement, SALSA could potentially enhance cancer detection, treatment planning, and response evaluation. “SALSA demonstrated high accuracy tumor detection with precision of over 99% at the patient level, and a lesion-by-lesion detection precision of almost 82% in the external validation cohort,” added Balaguer-Montero.
“This novel deep learning-driven tool has shown precise and automated identification and delineation of liver cancer on CT images, facilitating a more precise quantification of tumor burden —a crucial factor in cancer prognosis and treatment—with no prior manual prompt requirements. Our validation across several test and external cohorts highlights SALSA’s effectiveness and reliability, matching, and often surpassing, the accuracy of expert radiologists,” observed Perez-Lopez.
A key objective of VHIO’s Radiomics Groups is to develop medical imaging tools based on imaging biomarkers including tumor volume, density, and texture. Emerging technologies with artificial intelligence and machine learning techniques are rapidly advancing the field of personalized oncology by providing a more precise evaluation of treatment response in individual patients. “Current clinical response criteria are limited. Tumor diameter is measured in 2D without considering volume and, in the case of metastases, only two tumors per organ and a maximum of five lesions per patient are evaluated,” said Raquel Pérez-López. “SALSA could be useful for the management of patients with liver cancer, enabling the measurement of parameters such as total tumor volume, density, or texture to assess response to therapy and support therapeutic decision-making to enhance patient outcomes,” she concluded.
This work has been possible thanks to the support received from the CRIS Cancer Foundation, FERO Foundation, and the “la Caixa” Foundation, that provide funding for various research projects led by VHIO’s Radiomics Group.
Source: Vall d’Hebron Institute of Oncology
08.05.2025