Image: Shutterstock/anyaivanova
Article • Seeking the right questions
Developing algorithms to assist routine pathology
Artificial Intelligence (AI) is destined for a significant role in assessing histology data but the key to developing the necessary algorithms lies in data quality – rather than the quantity, according to Professor Jens Rittscher.
Report: Mark Nicholls
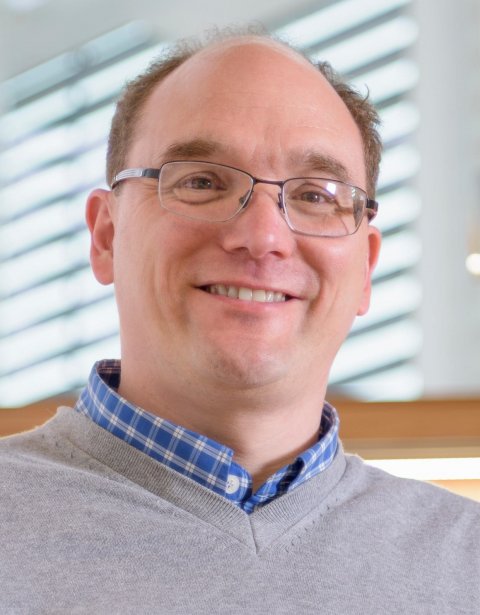
He also warns that we are some distance from seeing AI replacing human pathologists in this scenario, primarily because presently the risks of automated decision-making are not understood. The core to developing the necessary algorithms to drive AI to support better data assessment, he said, is the availability of annotated data for training, validation, and testing.
Rittscher, Professor of Engineering Science at the University of Oxford, explained that certain efforts focus on collecting a very large number of cases to develop algorithms to assist routine pathology. However, we should not underestimate the importance of ‘highly-curated data’ that are being collected and annotated with a specific clinical question in mind. ‘It’s the precision of the question you ask and then the quality and depth of the data,’ he said. ‘As we develop predictive models, I think it will be necessary to set up better prospective cohorts to validate these methods going forward, because there is only so much you can learn from retrospective data.’
This is where the human will be overwhelmed by the information and need guidance by a computer
Jens Rittscher
He pointed to three key ways that AI will assist histology data assessment: workflow improvements; helping to build a bridge between molecular tests and clinical practice, and applying new imaging approaches. ‘With workflow improvements, if we can predict what other molecular tests should be run on a specific case or, in the case of referral to a specialised centre, which histopathology would be helpful to assess the risk profile, then automated reporting is going to be important.’ This will be of particular relevance when a specialist is not available, when time is of the essence, or there is a need to order more complicated tests.
Today, it is difficult for oncologists to extract information from a pathology report. Including a visual summary of certain key histological features would dramatically improve the accessibility of the pathology report and help to close the gap between the two disciplines, he said.
Rittscher’s team recently conducted new research showing molecular subtypes of colorectal cancer can be predicted using histology alone. ‘This is one of the prototypical examples where you can now add information that pathologists could not really provide before,’ he said.
A third area is the application of new imaging methods to pathology images and looking at multiple protein markers in the same tissue section. ‘This is where the human will be overwhelmed by the information and need guidance by a computer,’ he continued. ‘Broadly, it will look at computer assisted forms of diagnostics for pathologists and this, I think, could dramatically enhance the role of pathologists.'
Recommended article
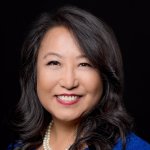
Article • Digital pathology & AI
Unleashing the power of digital pathology for precision medicine
Digital pathology, combined with the power of Artificial Intelligence (AI), is one of the most promising fields for the delivery of precision medicine. In the first keynote address for the 5th Digital Pathology & AI Congress (Europe) held in London last December, Professor of Pathology, Marilyn Bui, focused on how digital pathology is impacting on precision medicine. During her address,…
AI will complement pathologists’ input
However, Rittscher is adamant that such technology will not replace human input, but will complement it. ‘I think the potential that AI can replace human pathologists is overblown and partially unfounded because we do not really understand the risk of automated decision-making, such as whether a patient will accept it, or liability issues,’ he said. ‘But where AI is good is in providing quantitative information and adding a quantitative aspect to pathology that humans cannot assess reliably today.
‘I think that is essentially what will drive the evolution of pathology and the convergence of further technologies in pathology – digitisation of slides, the quantitative information and the wider visibility of in vitro diagnostics – holds the potential to change how the different clinical disciplines work together today and give pathologists a completely new role.’
‘However, he added, ‘This also has broad implications for the education and training of pathologists, he added.
A ‘bottleneck’ in any advances remains because of the limited availability of experts, particularly in terms of the time needed to curate and annotate large data sets. ‘It’s a labour-intensive task to curate these and annotate them to the quality and specificity that you can actually use them later on,’ he pointed out. To optimise experts’ time, computer assisted tools need to be developed that effectively support the generation of validated image level annotations. He believes the future will see a strengthening in the capability of pathology departments to find and deliver better routes into molecular or in vitro tests and to make the data in the pathology report more accessible to other clinical disciplines, such as referring physicians or oncologists.
Profile:
Jens Rittscher is Professor of Engineering Science at the University of Oxford, an appointment held between the Institute of Biomedical Engineering and the Nuffield Department of Medicine. He is a group leader at the Big Data Institute and affiliated to the Ludwig Institute of Cancer Research. Previously, he was a senior research scientist and manager at GE Global Research in America. His research focus is on enabling biomedical imaging via the development of new algorithms and novel computational platforms, with a current focus to improve mechanistic understanding of cancer and patient care through quantitative analysis of image data.
06.08.2019