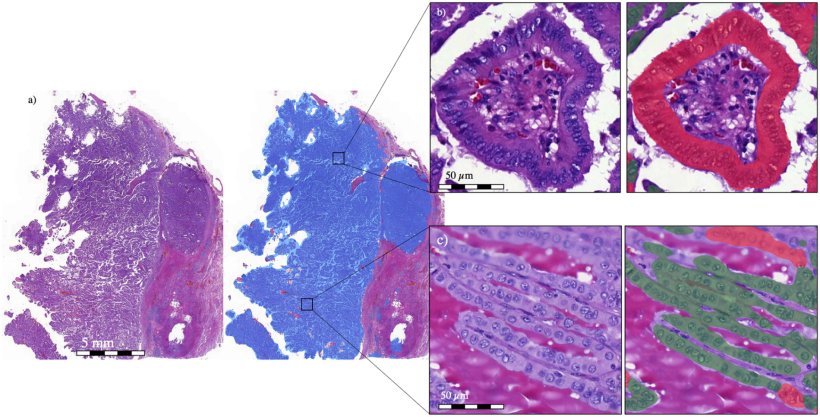
Image source: Stenman et al., PLOS One 2022 (CC BY 4.0)
Supervised learning approach
A new deep learning-based algorithm to predict relapse-free survival in papillary thyroid carcinoma
According to the World Health Organization, the tall cell variant (TCV) is an aggressive subtype of papillary thyroid carcinoma (PTC) featuring at least 30% epithelial cells two to three times longer than they are wide. In practice, applying this distinction is difficult, leading to substantial variations between observers.
‘That’s why we are developing and training a deep learning algorithm using supervised learning to detect and quantify the proportion of tall cells (TCs) in papillary thyroid carcinoma,’ explained Sebastian Stenman, researcher from the Institute for Molecular Medicine, and the Department of Pathology at the University of Helsinki, Finland.
We showed that the DL-based algorithm was better than the human observer in identifying tall cell variants
Sebastian Stenman
In summer 2022, his research team is testing it on an independent data set, and further validating it on an independent set of 90 papillary thyroid carcinoma samples from patients treated at the Hospital District of Helsinki and Uusimaa (HUS) between 2003 and 2013. The Finnish scientist compared the algorithm-based tall cell ratio to independent scoring by a human investigator and looked at how those scores were associated with disease outcomes.
The results published revealed that the deep learning algorithm detected tall cells with a sensitivity of 93.7% and a specificity of 94.5%. In the validation set, the deep learning algorithm tall cell scores correlated with a diminished relapse-free survival. ‘We showed that the DL-based algorithm was better than the human observer in identifying tall cell variants,’ said Stenman. The algorithm could prove useful as a clinical tool for pathologists when evaluating PTC samples and could potentially significantly improve the consistency of TCV case assessment. So far, no such algorithm has been described.
20.10.2022