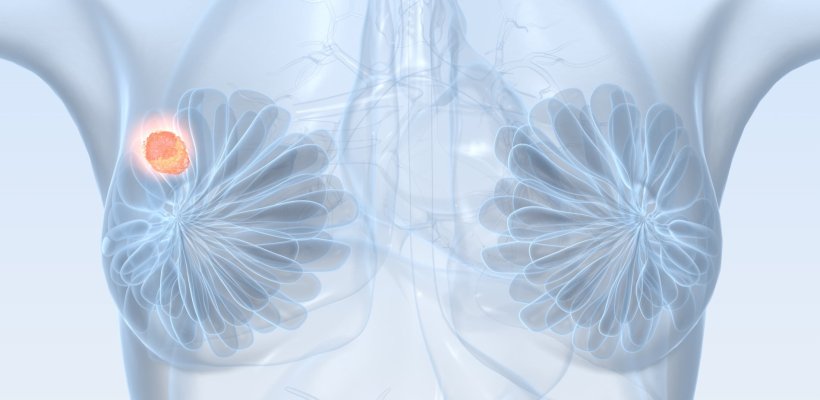
© Axel Kock – stock.adobe.com
News • Machine learning analysis
Breast cancer: AI predicts metastasis from recurrence
Machine learning can accurately predict the type of local breast cancer recurrence after initial treatment and assess the likelihood of distant metastases, a new study by Semmelweis University shows.
By analysing data from 154 patients, researchers found that the longer the time between initial diagnosis and local tumour recurrence, the lower the risk of metastasis. The findings, published in Scientific Reports, represent an essential step towards developing personalised treatment strategies.
Applying four different mathematical models, the researchers aimed to determine whether machine learning could predict the exact location of tumour recurrence and estimate the likelihood of metastasis in breast cancer patients. Local recurrence differs from metastasis and can occur in the remaining breast tissue, chest wall, skin, or surgical scar. They analysed clinical and pathological data from 154 women, with an average follow-up time of 11 years (133.16 months) after their initial diagnosis. Data were available for both their primary tumour and local recurrence. The best-performing model predicted recurrence in the remaining breast tissue with 77% accuracy, while recurrence in surgical scars was predicted with 69% accuracy.
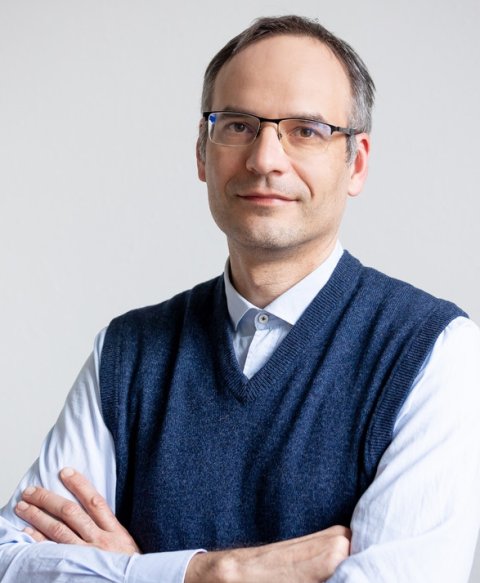
Image source: Semmelweis University; photo: Boglarka Zellei
The most significant predictive factors included the type of surgery (breast-conserving or mastectomy), the patient’s age at diagnosis, the proliferative activity of tumour cells as indicated by Ki67 protein levels, and progesterone receptor status (low levels or absence of progesterone receptors negatively affect the outcome of the disease). Another key objective was to predict the risk of distant metastases after local recurrence. Machine learning models achieved 78% accuracy in this task.
“These models linked metastasis formation primarily to the recurrence location, the time elapsed between initial diagnosis and recurrence, and the treatment received after recurrence”, explains Dr Kristóf Attila Kovács, an assistant lecturer at Semmelweis University’s Department of Pathology, Forensic and Insurance Medicine and the first author of the study.
Metastasis risk was lower when the tumour recurred in the remaining breast tissue. In contrast, recurrence in the chest wall significantly increased the likelihood of the tumour spreading to other organs.
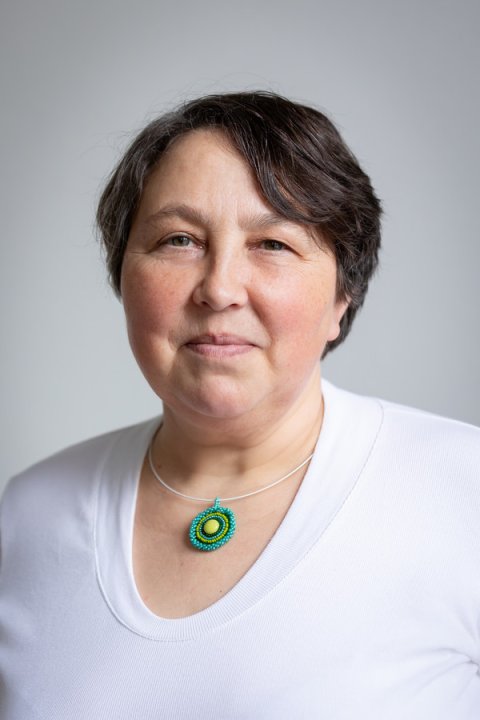
Image source: Semmelweis University; photo: Boglarka Zellei
The study also found that tumours recurring within two years of the initial diagnosis were much more likely to lead to metastases. In contrast, local recurrences after five years were typically associated with hormone-sensitive tumours with a better prognosis. One-third of the patients experienced multiple recurrences, further increasing the risk of metastases.
“Unfortunately, even in cases of locally recurring breast cancer treated with chemotherapy, the likelihood of distant metastases remains significant. This suggests that in such aggressive cases, alternative targeted therapies may be needed, even for secondary tumours near the surgical site”, says Dr Anna Mária Tőkés, senior researcher at Semmelweis University’s Department of Pathology, Forensic and Insurance Medicine and the study’s corresponding author.
In 2022, 2.3 million women were living with breast cancer, and 670,000 died from the disease worldwide, according to the World Health Organization (WHO). Although in developed countries survival rates for breast cancer patients have significantly improved in the last decades, the tumour can return in 5–20% of cases even after successful initial treatment, the researchers warn. “These local recurrences vary significantly – some are more aggressive than others – so they may require different treatment approaches. However, current guidelines recommend mostly similar treatment for all of them. Tailoring treatment based on risk factors could improve survival outcomes, which is why understanding the prognosis of different recurrence types is crucial”, adds Dr Kovács.
The researchers emphasise that further studies involving larger patient populations and genetic tumour analysis could refine prediction models. Combining machine learning with traditional diagnostic methods could help identify high-risk patients and guide targeted treatment strategies at the right time – an approach that could significantly improve treatment effectiveness and survival outcomes.
Among the 154 patients analysed, 33 experienced recurrence after 10 years, and one patient’s breast cancer returned after 23 years. Many patients stop attending follow-up appointments after being declared cancer-free. Yet, regular check-ups remain lifesaving, even decades after treatment.
Source: Semmelweis University; text: Angelika Erdélyi
28.03.2025