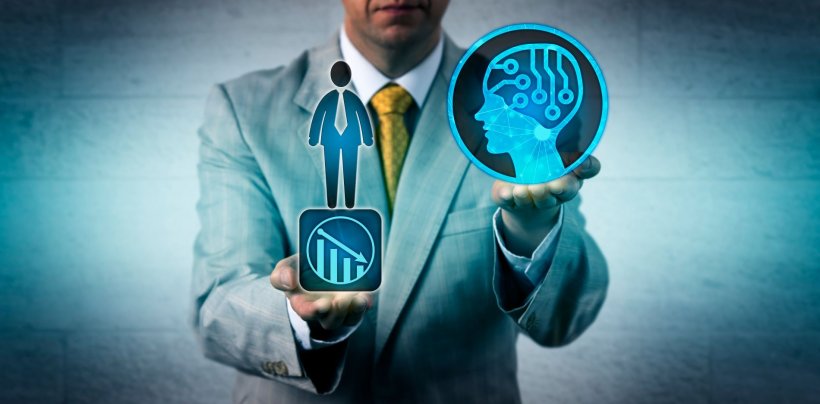
Image source: Shutterstock/LeoWolfert
Article • Heard at SIIM 2021
AI in radiology: unexpected benefits, unintended consequences
Artificial intelligence (AI) could match the impact of PACS on radiology. Covid-19 stimulated the development and testing of AI diagnostic-aiding tools in radiology, an unintended consequence of the pandemic. More image data sets have been created to train AI software – an unexpected benefit for radiology research.
Report: Cynthia E. Keen
The Samuel Dwyer Memorial Lecture at the virtual 2021 Society of Imaging Informatics in Medicine (SIIM) annual meeting, in May, focused on the exponential development of medical AI research and ‘approved’ radiology AI tools, as well as the challenges to achieve expected quality, safety, and performance attributes.
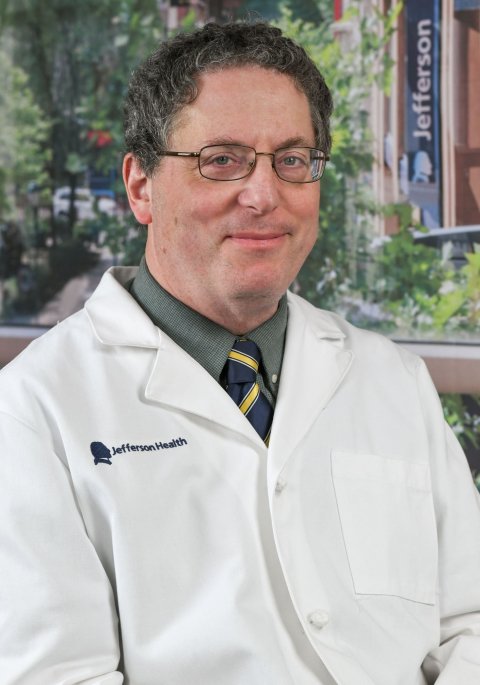
Adam E Flanders MD, Professor of Radiology at Thomas Jefferson University Hospital, Philadelphia, delivered the ‘Serendipity and Unintended Consequences’ lecture, it reflected the visionary perspective of the late PACS pioneer Professor Samuel J Dwyer III, whose seminal research on the retrieval rates of radiology exams formed the basis for PACS storage systems. Flanders likened the surge in AI tool development, and image data set expansion, to the 1960s space race, which launched the digital age, and to the global campaign to end the use of DDT. The latter produced a negative unintended consequence: injury to wildlife was reduced, including mosquitoes, which then caused a large increase in cases of malaria.
This is huge – potentially it could improve diagnostic quality, to efficiently extend access and be a panacea to major cost reductions in medicine. Flanders referenced experts who predict that healthcare AI overall will be a US$36 billion industry by 2025, generating a US$150 billion annual savings in AI-enabled healthcare by 2026. HealthITAnalytics reported, in 2019, that the top three areas of healthcare AI investment were robot-assisted surgery ($40 billion), virtual nursing assistants ($20 billion), and administrative workflow ($18 billion). By comparison, AI for preliminary diagnosis and automated image diagnosis ranked at the bottom of its list, at $5 billion and $3 billion respectively.
AI needs guardrails. It doesn’t know when it doesn’t have enough information when making a decision
Adam E. Flanders
Nonetheless, there has been a tremendous surge in interest in AI in radiology, stimulated in part by the 2016 prediction by Geoffrey Hinton PhD, among the godfathers of deep learning and neural networks, that AI would diagnostically outperform radiologists by 2021 – radiologist’s wake-up call to that AI would impact hugely on their medical specialty. Interest surged in the potential of medical imaging AI. ‘But is diagnostic AI on the right track? Is it happening too fast? Are image databases used to train AI software diverse enough, inclusive enough? Is there enough information?’ Flanders asked. ‘AI needs guardrails. It doesn’t know when it doesn’t have enough information when making a decision.’ Flanders cited an example of a brain CT angiogram, whereby the AI interpretation was a technical error of insufficient contrast material when, in fact, the patient was brain dead. While a radiologist can infer the correct diagnosis in this instance, it underscores the fact that AI is fundamentally limited to entities for which it is trained.
As of 2021, 222 AI medical devices were cleared by the FDA. Of these, 129 were for radiology applications, with 77% manufactured by small companies. But there is no agreed definition of an AI device or specific regulatory pathway, either in the USA or Europe. Is there enough rigorous evaluation? Flanders thinks not, nor do many other radiologists now speaking out publicly. Researchers from Radboud University Medical Centre in Nijmegen, the Netherlands, evaluated 100 CE-marked AI software products for clinical radiology from 54 vendors listed in www.aiforradiology.com. They reported in European Radiology that 64 products had no peer-reviewed evidence of efficacy, and that only 18 of the 100 had evidence of efficacy rated as 3/6 or higher, validating impact on diagnostic decision-making, patient outcome or cost.
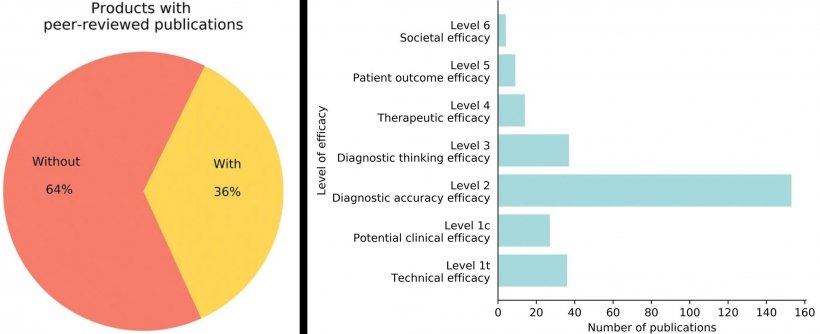
Image source: van Leeuwen et al., European Radiology 2021 (CC BY 4.0)
Between January and October 2020, 320 published papers described new machine learning-based models to detect Covid-19 in chest CT images and chest radiographs. Writing in Nature Machine Intelligence, researchers from Cambridge University reported that none of these models were of potential clinical use due to methodological flaws and/or underlying biases. Most failed to describe a reproducible methodology or failed to follow best practice for ML model development, and/or failed to show sufficient external validation to justify wider applicability of the method.
Covid-19 is the initial research use case, but there is every reason to believe that this infrastructure can be expanded to support medical image AI research for many other conditions
Adam E. Flanders
Flanders said a recent editorial in Radiology ‘Artificial Intelligence of Covid-19 Imaging: A Hammer in Search of a Nail’ was even blunter. ‘To a large extent, the large quantity and rapid publication of articles on AI for Covid-19 are emblematic of current trends in other areas of radiology AI. It’s now so much easier to design and conduct a radiology AI experiment. The only prerequisite seems to be possession of a large data set,’ observed Ronald M Summers MD PhD, of the National Institutes of Health Clinical Center’s Imaging Biomarkers and Computer-Aided Diagnosis Laboratory of Bethesda, Maryland. ‘International standards to enable meaningful comparisons of the performance are needed for AI software, emphasised Flanders. ‘Prospective outcomes studies are necessary to determine whether use of AI leads to changes in patient care, shortened hospitalisations, and reduced morbidity and mortality.’
Access to data is critical, but has historically been monolithic, isolated, and difficult. Governments are now starting to support image data collaboration. Covid-19 brought a huge opportunity, because significant R&D funds became available to facilitate image data sharing and collaboration. One example is the Medical Imaging and Data Resource Center (MIDRC), a consortium formed by the American College of Radiology (ACR), the Radiological society of North America (RSNA), and the American Association of Physics in Medicine (AAPM) for image collection, curation and distribution of Covid-19 related imaging data for AI research.
MIDRC supports 12 internal Covid-related research projects. Its repository is expected to be the largest open database of anonymised Covid-19 medical images and associated clinical data globally. ‘There’s no organ system in the body not affected by Covid-19. I predict this image repository, which welcomes image data contributions from throughout the world, will be of tremendous benefit to AI research and innovations for radiology,’ Flanders told Healthcare in Europe. ‘Covid-19 is the initial research use case, but there is every reason to believe that this infrastructure can be expanded to support medical image AI research for many other conditions.’
Flanders advises contribution of data to public data repositories to mitigate bias and strength diversity in AI research and development. He recommends promotion of new international evaluation checklists for testing, and radiologists should retain an appropriate level of scepticism when assessing products for clinical use, and when purchasing radiology AI tools, mandate vendors to provide a complete account of performance. ‘Most importantly, remain engaged in this very exciting, accelerating, and ever-changing process. Just as radiologists’ input helped to perfect PACS to be the utilitarian technology it now is, most likely they will do the same for radiology AI tools as they become ubiquitous.’
Profile:
Adam E Flanders MD, Professor of Radiology and Rehabilitation Medicine at Thomas Jefferson University Hospital in Philadelphia, is co-director of neuroradiology/ENT radiology and vice chair of imaging informatics, also chairing the Imaging Informatics Council for the Enterprise Radiology and Imaging Service Line for Jefferson. He has led a multi-centre collaborative project to examine imaging features, pathology, and genomics of human gliomas from the Cancer Genome Project.
15.07.2021