Article • Skin cancer identification
Dermatology & AI: The need to quantify skin tones
Although artificial intelligence (AI) tools and smartphone apps that help identify suspicious moles and potential skin cancers are starting to proliferate, dermatology informatics has far to go before becoming a clinically adoptable technology. Many challenges need to be resolved, not least of which is the need for more image data representing people of colour.
Report: Cynthia E. Keen
Image source: Shutterstock/Maridav
Recently, three dermatologists working to advance informatics and the use of AI for dermatology discussed current challenges at the 2021 Society of Imaging Informatics in Medicine (SIIM) annual meeting. The scientific session raised more questions than answers, making it apparent that much more collaborative research needs to be undertaken as a global initiative.
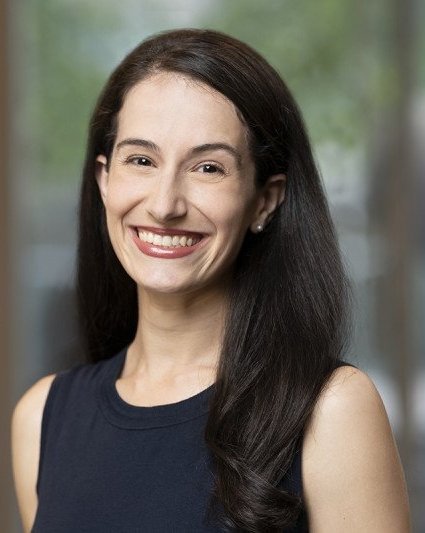
Image source: Memorial Sloan Kettering Cancer Center
The scope of dermatology photographs is broad, encompassing photos taken by patients and their primary care physicians, clinical photos, wound care photos, and histopathology CT scans. All of these need to be managed, labelled and stored appropriately, or else they cannot be used clinically or as reference data, said Veronica Rotemberg, MD PhD, of the Memorial Sloan Kettering Cancer Center in New York City. ‘Standards for image acquisition are challenging. Many imaging standards need to be established, such as setting photography standards, standards for special considerations for sensitive sites, and standards for skin of colour.’
The use of DICOM, the international standard for medical imaging, for dermatological imaging has been limited. The ability of DICOM to encode relevant metadata and enable interoperability with electronic health records and clinical research software has driven the interest in its application to dermatology. A DICOM dermatology working group has developed dermatology-specific Information Object Definitions (IODs) for inclusion in the DICOM standard and future work will be for total body photography and reflectance confocal microscopy. ‘There are numerous issues to resolve,’ Rotemberg pointed out. ‘No consensus exists yet on the specific fields to collect and store for every type of image. Patient level variables, such as ethnicity and skin type, need to be considered. Colour accuracy and fidelity are huge issues. In addition to lighting affecting colour, there is much variability in image sources from smartphones. Each acquisition device may code RGB values differently when capturing and storing images. With respect to standard file formats and colour fidelity, there is a wide variety on how to store images with uncompressed or compressed JPG, and the compression level used is not always encoded.’
Once image capture challenges are resolved, what should be done with the images? Research is underway in the fields of segmentation, wound measurement, and diagnosis using machine learning and AI, Rotemberg said, adding that a 2020 SIIM-ISIC Challenge for melanoma classification outperformed dermatologists, with a sensitivity of 79.3% for AI compared to 71.9% for dermatologists. However, issues remain for clinical implementation of AI for skin cancer screening, such as identifying the appropriate evaluation criteria and appropriate users.
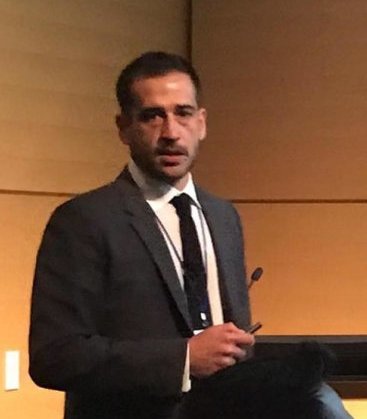
Image storage requirements for dermatology data can be another stumbling block, suggests Konstantinos Liopyris, MD PhD, of the University of Athens Andreas Syggros Hospital of Skin and Venereal Diseases. Dermatology data is massive, including patient demographics, anatomic locations of lesions, biopsy results, treatment modality data, biopsy results, diagnoses, and outcomes. Image data can be clinical, dermoscopic, RCM images, and OTC images for both initial and all follow-up visits.
Universal and reproducible surface anatomy terminology is essential. In 2020, Liopyris participated in an initiative by the International Skin Imaging Collaboration to establish a standardised set of 513 terms to describe clinically relevant human surface anatomy for dermatologic documentation. More work is still needed, he said.
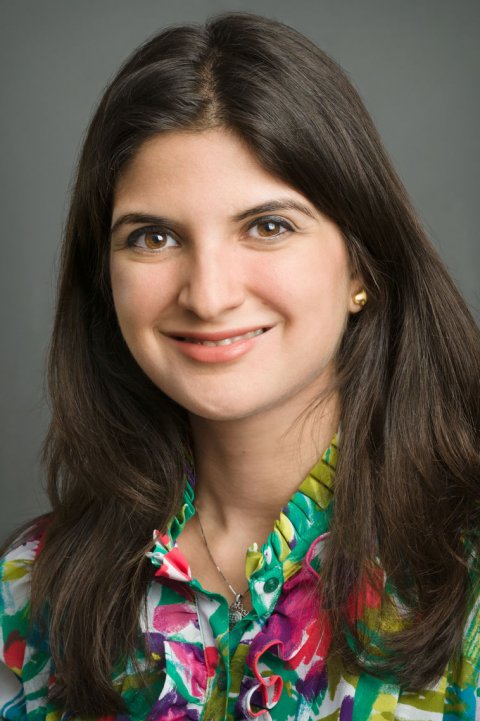
Image source: Stanford School of Medicine
A major roadblock in developing AI tools for dermatology is that dermatology datasets have focused on Caucasian ‘white’ skin, said Roxana Daneshjou, MD PhD, of Stanford University School of Medicine. It is imperative to address biases in image-based dermatology, which for decades has underrepresented darker skin tones. Without appropriate representation of all human skin colours, disparities can get exacerbated when transferred to AI datasets, Daneshjou explained. ‘Bias exists at every stage of algorithm development. We are working in a ”black box” about whether a training algorithm for an AI tool has enough representation of range of skin tone. Not knowing this means there is no way to determine how well an AI algorithm works in darker skin colours.’ She agreed that colour balancing of photography has favoured lighter skin, and that digital cameras may still have differences in colour balancing that can affect skin tones, creating a discordance between real life and the colour captured. Because natural light, room light and overhead light can also change colours, the lighting in a clinic can affect how a colour is represented and what is captured as a dermatologic image.
What should be done? The experts concurred that more representative samples are needed to develop AI tools and researchers need to understand the technical challenges with photography and image capture. How to quantify skin tones to include the total range of human skin colour Is essential. As the experts summed up, ‘There’s lots of work to do and lots of work being done right now to make this a better reality.’
Profiles:
Roxana Daneshjou, MD PhD, is a clinical scholar in dermatology and a postdoctoral research fellow in biomedical data sciences at Stanford University School of Medicine in Redwood City, California. She is interested in bridging new technologies such as genomics and machine learning with clinical medicine and in the use of Twitter for scientific communication and medical education.
Dermatologist Konstantinos Liopyris, MD PhD, specialises in skin cancer at the University of Athens Andreas Syggros Hospital of Skin and Venereal Diseases, in Greece. Also a research fellow at the Memorial Sloan Kettering Cancer Center in New York City, he specialises in medical image processing AI in dermatology.
Dermatologist Veronica Rotemberg, MD PhD, specialises in skin cancer and directs the imaging informatics program at the Memorial Sloan Kettering Cancer Center (MSKCC), in New York City. A biomedical engineer, her research interests include dermatology imaging standards, high resolution skin imaging, clinical photograph workflows and AI for diagnosis.
02.09.2021