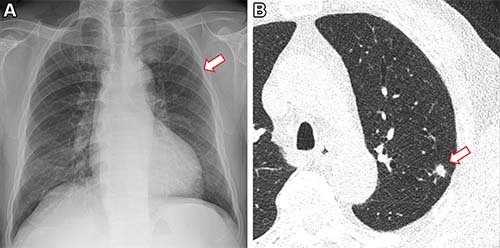
Image credit: RSNA/Anika S. Walia, B.A.
News • Opportunistic screening using chest X-rays
AI identifies non-smokers at high risk for lung cancer
Using a routine chest X-ray image, an artificial intelligence (AI) tool can identify non-smokers who are at high risk for lung cancer, according to a study being presented next week at the annual meeting of the Radiological Society of North America (RSNA).
Lung cancer is the most common cause of cancer death. The American Cancer Society estimates about 238,340 new cases of lung cancer in the United States this year and 127,070 lung cancer deaths. Approximately 10-20% of lung cancers occur in “never-smokers” – people who have never smoked cigarettes or smoked fewer than 100 cigarettes in their lifetime.
The United States Preventive Services Task Force (USPSTF) currently recommends lung cancer screening with low-dose CT for adults between the ages of 50 and 80 who have at least a 20 pack-year smoking history and currently smoke or have quit within the past 15 years. The USPSTF does not recommend screening for individuals who have never smoked or who have smoked very little. However, incidence of lung cancer among never-smokers is on the rise, and—without early detection through screening—when discovered, these cancers tend to be more advanced than those found in smokers.
Since cigarette smoking rates are declining, approaches to detect lung cancer early in those who do not smoke are going to be increasingly important
Michael T. Lu
“Current Medicare and USPSTF guidelines recommend lung cancer screening CT only for individuals with a substantial smoking history,” said the study’s lead author, Anika S. Walia, B.A., a medical student at Boston University School of Medicine and researcher at the Cardiovascular Imaging Research Center (CIRC) at Massachusetts General Hospital (MGH) and Harvard Medical School in Boston. “However, lung cancer is increasingly common in never-smokers and often presents at an advanced stage.”
One reason federal guidelines exclude never-smokers from screening recommendations is because it is difficult to predict lung cancer risk in this population. Existing lung cancer risk scores require information that is not readily available for most individuals, such as family history of lung cancer, pulmonary function testing or serum biomarkers.
For the study, CIRC researchers set out to improve lung cancer risk prediction in never-smokers by testing whether a deep learning model could identify never-smokers at high risk for lung cancer, based on their chest X-rays from the electronic medical record. Deep learning is an advanced type of AI that can be trained to search X-ray images to find patterns associated with disease. “A major advantage to our approach is that it only requires a single chest-X-ray image, which is one of the most common tests in medicine and widely available in the electronic medical record,” Walia said.
The “CXR-Lung-Risk” model was developed using 147,497 chest X-rays of 40,643 asymptomatic smokers and never-smokers from the Prostate, Lung, Colorectal, and Ovarian (PLCO) cancer screening trial to predict lung-related mortality risk, based on a single chest X-ray image as input.
The researchers externally validated the model in a separate group of never-smokers having routine outpatient chest X-rays from 2013 to 2014. The primary outcome was six-year incident lung cancer, identified using International Classification of Disease codes. Risk scores were then converted to low, moderate and high-risk groups based on externally derived risk thresholds. Of 17,407 patients (mean age 63 years) included in the study, 28% were deemed high risk by the deep learning model, and 2.9% of these patients later had a diagnosis of lung cancer. The high-risk group well exceeded the 1.3% six-year risk threshold where lung cancer screening CT is recommended by National Comprehensive Cancer Network guidelines. After adjusting for age, sex, race, previous lower respiratory tract infection and prevalent chronic obstructive pulmonary disease, there was still a 2.1 times greater risk of developing lung cancer in the high-risk group compared to the low-risk group.
“This AI tool opens the door for opportunistic screening for never-smokers at high risk of lung cancer, using existing chest X-rays in the electronic medical record,” said senior author Michael T. Lu, M.D., M.P.H., director of artificial intelligence and co-director of CIRC at MGH. “Since cigarette smoking rates are declining, approaches to detect lung cancer early in those who do not smoke are going to be increasingly important.”
Additional co-authors are Saman Doroodgar Jorshery, M.D., Ph.D., and Vineet K. Raghu, Ph.D. The researchers report support from the Boston University School of Medicine Student Committee on Medical School Affairs, National Academy of Medicine/Johnson & Johnson Innovation Quickfire Challenge, and the Risk Management Corporation of the Harvard Medical Institutions Incorporated.
Source: Radiological Society of North America
22.11.2023