Image source: Shutterstock/LuckyStep
Article • Improving the role of radiology
Value-based healthcare: AI reveals the bigger picture
Value-based healthcare is gaining momentum and radiologists must increasingly show their contribution in improving patient care. Artificial intelligence (AI) can help them to do so and brings a series of new opportunities, according to Charles E Kahn, Professor and Vice Chairman of Radiology at the University of Pennsylvania, speaking at a meeting in Madrid in January.
Report: Mélisande Rouger
AI can do a lot to improve radiology’s role in healthcare, from choosing the right procedure to predicting diseases, Kahn told delegates at Triángulo, a meeting organised by prominent Spanish radiologists including Luis Donoso Bach (Barcelona), Luis Martí-Bonmatí (Valencia) and Eduardo Fraile Moreno (Madrid).
Improving procedure selection and findings
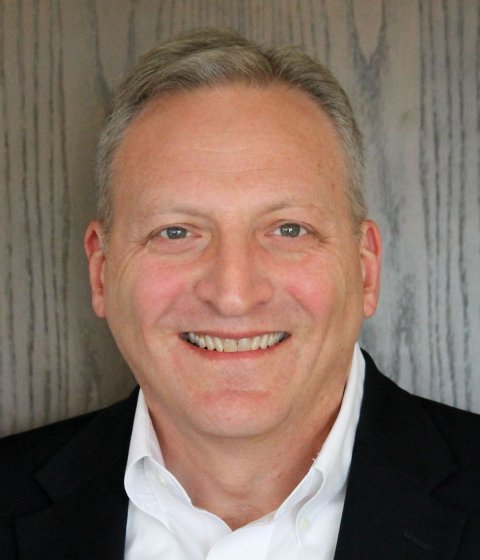
First, AI can improve procedure selection, a process in which radiologists are not often involved, although ‘that may be the most important part of the job in radiology,’ Kahn pointed out. Deep learning (DL) systems, for example, could extract information from electronic health records to look at patterns of previous patients with previous diseases, to know which procedures worked best for them. Based on that information, algorithms could be created that will help select imaging procedures more precisely. For protocol selection with contrast examinations, DL could help chose how contrast should be administered – intravenously or orally – and determine scan parameters, to better answer clinical questions.
The way radiologists view studies in their PACS is not optimal right now and AI could help improve image display based on previous preferences, Kahn suggested. ‘DL could help automatically to arrange image display by using previous patterns and identify image series that are likely to be useful. Some PACS vendors are developing intelligent ways of mapping images that watch what you do when you select the images,’ he said. DL algorithms can also help reconstruct MRI images from k-space data and provide an alternative to iterative reconstruction for CT, to further reduce radiation dose.
Many efforts have been put into improving findings to advance image segmentation to determine the extent of disease; assess diagnosis, staging and imaging phenotypes; and monitor disease. AI segmentation tools could alleviate the radiologists’ workload and improve their performance, by tracking and measuring lesions to adjust therapies in follow-up CT scans in cancer patients. Systems using deep convolutional neural networks have been developed for automated real-time triaging of adult chest radiographs on the basis of the urgency of imaging appearances. Such systems have notably helped sort out patients in chest radiograph triage in the UK, reducing average reporting delay from 11.2 to 2.7 days for critical imaging findings, a study published last year in Radiology showed.
Matching results with pathology and following up recommendations
Opportunistic screening uses AI tools to search images routinely for conditions that suggest a health risk
Charles E. Kahn
Hospital electronic systems contain large amounts of written information, which can be extracted and fed to AI systems for training purposes. Combining machine learning (ML) and natural language processing (NLP) to categorise tumour response in radiology reports can be an interesting solution. At Penn Medicine, those reports all feature a code that indicates tumour growth or regression, to enable to extract information that is relevant for patient management.
Matching radiology data with pathology information can be key to show value of imaging studies in the healthcare continuum. ‘An ideal system would link pathology results with radiology procedures so that radiologists could be sure to know the outcomes of their biopsies,’ Kahn said. AI reveals the bigger picture and enables things to be done at a scale not possible before, he added.
Predicting disease is part of that wider scheme. One way to predict disease is by taking measurements with DL during opportunistic screening, a strategy that is gaining momentum. ‘Opportunistic screening uses AI tools to search images routinely for conditions that suggest a health risk. For example, AI can measure coronary artery calcification on chest CTs to assess a patient’s risk of heart disease,’ he said. ‘Having the information that early means being able to provide better patient prognosis.’ Opportunistic screening with AI may also prove useful in other conditions, such as osteoporosis, abdominal aortic aneurysm, atherosclerosis, emphysema and cirrhosis.
The Centre for Practice Transformation (CPX) in Penn Medicine’s Radiology Department unites efforts in patient management, IT, and quality improvement initiatives and innovation. Some of their projects include the implementation of an imaging clinical decision support; fit-for-purpose exams; patient-oriented reports; radiology-pathology integration. On the disease front, the Centre is tackling lung cancer screening and nodule tracking; multiple sclerosis plaque analysis; abdominal organ volumetry and fat analysis. The centre also promotes report text analysis using NLP for recommendation tracking. ‘We know that a large proportion of our recommendations are never acted on. We want to ensure that patients are getting the care they need,’ Kahn concluded.
Profile:
Charles E Kahn is Professor and Vice Chairman of Radiology at the University of Pennsylvania. He is also the Editor of Radiology: Artificial Intelligence, an official journal of the Radiological Society of North America (RSNA).
19.08.2020