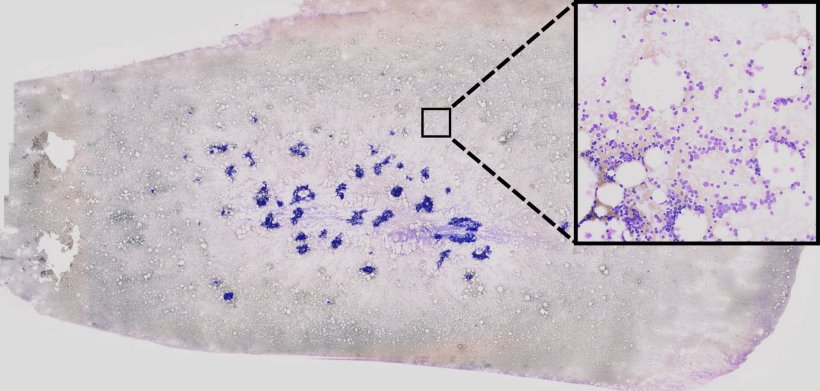
© AG Risse
News • Finding therapy-relevant genetics
Leukaemia: AI provides support in diagnostics
IT specialists and physicians develop new method for recognising genetic aberrations
Decisions on treatment for patients with acute myeloid leukemia (AML) – a highly aggressive form of leukemia – are based, among other things, on a series of certain genetic features of the disease; but at the time when a diagnosis is made, this information is not available. However, evidence of these genetic anomalies is crucial in providing targeted treatment for patients at an early stage. As genetic testing is expensive and time-consuming, there is a great need for inexpensive, fast and broadly accessible tests to predict such anomalies. A team of IT specialists and physicians at the University of Münster and the University Hospital Münster has now published a study showing how a method based on artificial intelligence (AI) can be used to predict various genetic features on the basis of high-resolution microscopic images of bone marrow smears. As a result, decisions on a more precise treatment can be made in future directly on the day of the diagnosis, without the need to wait for genetic analyses. The results have been published in the journal “Blood Advances”.
Interestingly, several patterns recognized by the algorithm can not be identified by human observers. This is for example because the patterns may be too faint or because extremely fine textures are involved which remain hidden to the human eye
Benjamin Risse
In this new method, the genetic aberrations were extracted directly from extremely high-resolution multi-gigabyte scans from whole bone marrow smears taken from more than 400 AML patients. The scans had a resolution of 270,000 times 135,000 pixels on average, with one image being several gigabytes in size. Proceeding from this enormous dataset, it was possible to extract more than two million single-cell images. “We developed a new type of Deep Learning method, fully automatic, which was trained for a complex task by means of machine learning technology,” explains Prof. Benjamin Risse, who headed the work on algorithmic developments on the IT side. “In our case, the basic algorithm can automatically recognise the genetic features and the very fine patterns in big cytological images. The method then filtered the single-cell images into categories of different cell types, and it also showed any genetic aberrations. Interestingly, several patterns recognized by the algorithm can not be identified by human observers. This is for example because the patterns may be too faint or because extremely fine textures are involved which remain hidden to the human eye, despite excellent imaging,” says Risse.
One key advantage of the method presented is in the end-to-end AI pipeline which enables monitoring of the (interim) results and reduces to a minimum the manual preliminary work often necessary for machine learning. This is made possible by a combination of so-called unsupervised, self-supervised and supervised learning processes. The first two processes require no manual data selection at all but try to extract relevant content automatically from the image data instead. “Using a so-called incremental approach, we carried out intermediary steps with a human expert to examine the images. This is necessary for example in cell images categorised as problematic,” says Dr. Linus Angenendt, who heads the Personalised Cancer Therapy and Digital Medicine working group at Münster University Hospital. Problematic cell images can occur as a result of incorrect staining, for example. The model trained was subsequently evaluated on an independent dataset relating to a further 70 patients with over 440,000 single-cell images – as a test cohort.
Although the new method cannot replace genetic analyses, it nevertheless helps at a very early stage in the diagnostic clarification process for a leukaemia patient, by providing an idea of which genetic aberrations might underlie the disease. This would be especially helpful in the case of aggressive diseases when there is no time to wait for the complete genetic analyses.
The researchers are confident that in future digital methods and artificial intelligence will become increasingly important for large medical datasets when it is a question of making personalised recommendations for treatment for patients with malignant diseases. This study contributes an important basis for this, for example in the development of similar approaches for other bone marrow diseases.
The work received funding from the European Union and the German Research Foundation.
Source: University of Münster
10.01.2024