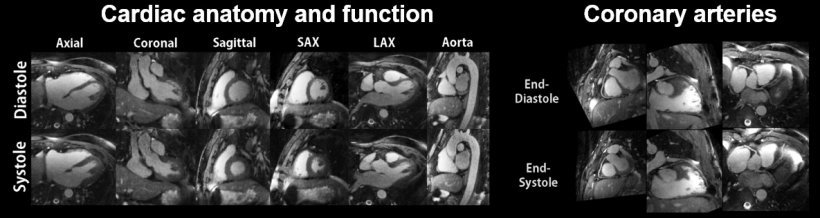
Credits: Li Feng, Ricardo Otazo, Tobias Block, Leon Axel, Matthias Stuber, Daniel Sodickson. Feng L et al, Magnetic Resonance in Medicine 2018 Feb;79(2):826-838, 5D whole-heart sparse MRI.
Article • Advancing radiology
Giving MRI a boost – and a brain
In his talk at the Garmisch Symposium, entitled “MRI in 5 minutes – Dream or Reality?” Dr. Daniel Sodickson of the New York University School of Medicine will give attendees a preview of the MR scanners of the future, which he likens to self-driving cars.
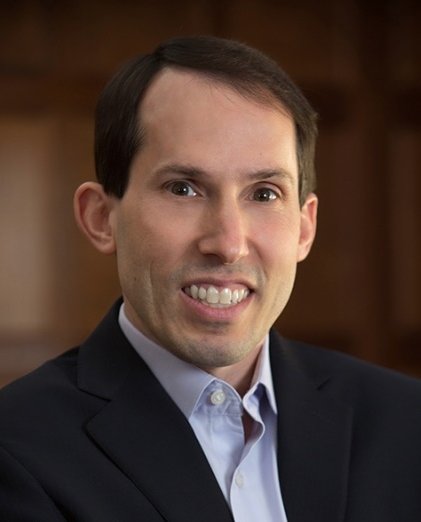
Sodickson — a professor and vice chair for research in the department of radiology at NYU, a principal investigator at the Center for Advanced Imaging Innovation and Research and director of the Bernard & Irene Schwartz Center for Biomedical Imaging — sees a number of changes coming to the imaging modality, including artificial intelligence (AI), that will make MR imaging much faster and more versatile. “I see the 5-minute MRI as sort of a bellwether for trends in imaging today,” Sodickson says. “AI might enable a new parable of ultra-fast MRI that’s going to change the experience of the patient and day-to-day workflow of the radiologist. There will be a number of other changes that go along with AI to make imaging super-fast.”
Sodickson says an alternate title for his talk might be “Imaging in a changing world: the scanners of the future and the future of scanning.” “In the future, MR scanners will move from snapshots to streaming,” Sodickson says.
AI could be the ‘brain’ of MRI
Traditionally, clinicians devote their efforts to one view of the anatomy, or series of slices, or a dynamic view, pausing between shots to perform exam setup. In the future, Sodickson predicts that software will adapt to a more modern paradigm, where the scanner gathers information about the patient continuously and the software then pieces the images together. Sodickson likens it to a “move from emulating the eye to imitating the brain.”
“How do we make sense of all that information?” Sodickson asks. “The model can come from how our own brains work.” All our senses — hearing, sight, smell, touch — are filtered through the brain, which turns them into actionable information. Similarly, AI can take the information from continuously acquired datasets and then can reconstruct full images from partial data.

Credits: Thomas Benkert, Tobias Block, Hersh Chandarana, Daniel Sodickson. Benkert T et al, Magnetic Resonance in Medicine 2017 Aug;78(2):565-576. Free-breathing volumetric fat/water separation by combining radial sampling, compressed sensing, and parallel imaging.
Snapshots are dead, long live streaming
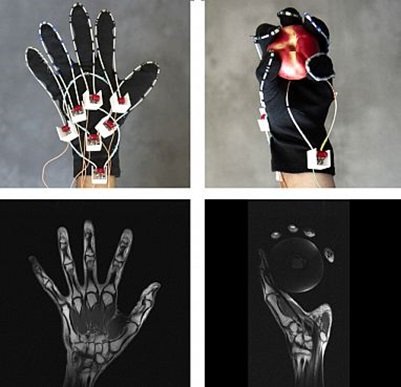
Credits: Bei Zhang, Martijn Cloos, Daniel Sodickson. Zhang B et al, Nature Biomedical Engineering 2018;2(8):570-577. A high-impedance detector-array glove for magnetic resonance imaging of the hand.
As part of his work, Sodickson and the NYU School of Medicine’s department of radiology is collaborating with the Facebook Artificial Intelligence Research (FAIR) group to speed up MRI scans while still acquiring enough data. Sodickson sees the work developing in two phases — using existing technology to gather less data and then innovating scanners to operate differently, almost like a self-driving car with multiple sensors. “The days of the carefully framed snapshot are, if not already over, certainly limited,” Sodickson says. “It behooves everyone to think about how to construct data streams rather than image series. In MRI and imaging more broadly, we’re not just following our eyes anymore, were emulating how we see the world. Stay tuned for imaging devices that start looking and feeling radically different than we’re used to.”
Profile:
Dr. Daniel Sodickson received a BS in Physics and a BA in Humanities from Yale College. He earned his PhD in Medical Physics from MIT and his MD from Harvard Medical School, both as a part of the Harvard-MIT Division of Health Sciences and Technology. He is professor and vice chair for research in the department of radiology at NYU, as well as a principal investigator at the Center for Advanced Imaging Innovation and Research and director of the Bernard & Irene Schwartz Center for Biomedical Imaging. Sodickson’s research is primarily focused on the development of new techniques for biomedical imaging, with the broad aim of seeing what has previously been invisible, in order to improve human health. He is credited with founding the field of parallel imaging, in which distributed arrays of detectors are used to gather magnetic resonance images at heretofore inaccessible speeds.
Session:
Thursday, 17. Jan 2019, 8:30-9:00 a.m.
MRT in 5 Minuten - Traum oder Zukunft?
(The 5 Minute MRI exam - dream or future?)
Dr. Daniel Sodickson (New York)
Session: MRT Innovationen
Cooperation with Facebook
At the end of November 2018, the NYU School of Medicine’s Department of Radiology released the first large-scale MRI dataset of its kind as part of the fastMRI project launched earlier that year with Facebook Artificial Intelligence Research.
While other sets of radiological images have been released previously, this dataset represents the largest public release of raw MRI data to date. The first phase of the project will involve data from knee MRI scans, but future releases will include data from liver and brain scans. “This collaboration focuses on applying the strengths of machine learning to reconstruct high-value images in new ways. Rather than using existing images to train AI algorithms, we will radically change the way medical images are acquired in the first place,” says Sodickson. “Our aim is not merely enhanced data mining with AI, but rather creating new capabilities for medical visualization, to benefit human health.”
16.01.2019