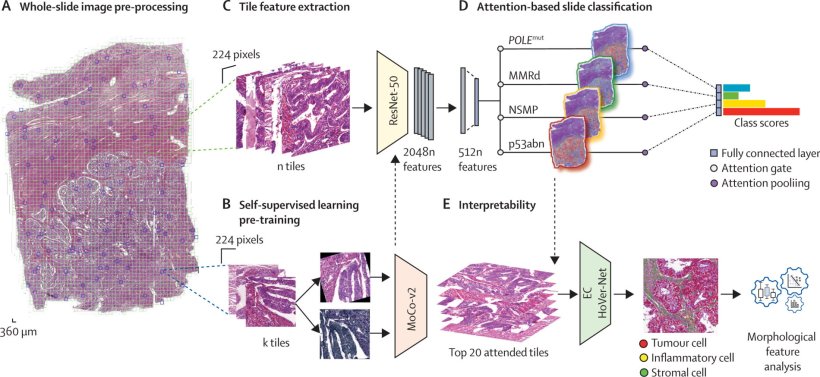
Image source: Fremond et al., Lancet Digital Health 2022 (CC BY 4.0)
News • Application in pathology
Endometrial cancer diagnostics: AI gives new insights
Research at the Leiden University Medical Center (LUMC) Department of Pathology shows the power of artificial intelligence (AI) applied to endometrial carcinoma microscopy images.
The group of Dr. Tjalling Bosse offers novel insights that could improve diagnosis and treatment of uterine cancer. Their findings have been published in The Lancet Digital Health.
Endometrial carcinoma is the most common cancer of the gynecologic tract. At the LUMC both clinical trials and translational research is conducted to improve the care for these patients. In the past years, the LUMC has had a leading role in the development of a novel tumor classification system based on molecular alterations, resulting in four endometrial cancer subtypes. Would it be possible to predict these molecular classes, based on microscopy-images alone?
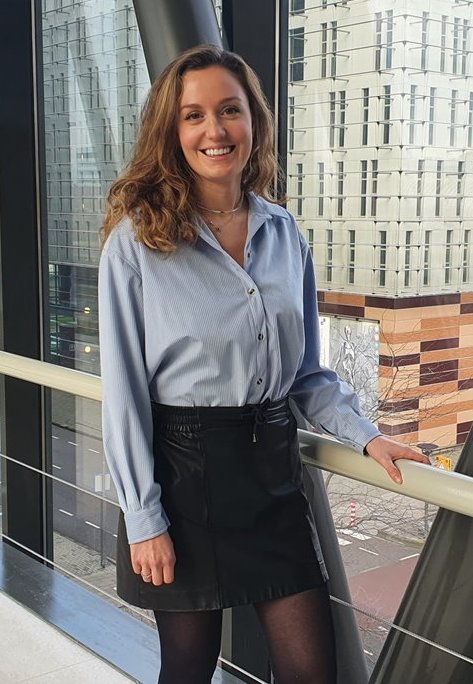
Image source: LUMC
Bosse and colleagues applied artificial intelligence on microscopy images of thousands of endometrial carcinoma images from patients that participated in the PORTEC-1 trials. His team developed a model that robustly predicts the four molecular classes of endometrial carcinomas based on one (hematoxylin and eosin)-stained microscopy slide image, which is the standard histological stain used in diagnostics for assessment of tumour grading and histological subtyping. This model was not “a black-box”, but through reverse-engineering the researchers were able to show which image-features were relevant for its predictions. The model provided the team with important novel insights that can be utilized in future studies to further improve diagnostics, prognostication, and management of endometrial cancer patients. “The application of AI in pathology is emerging. In this project we studied the morphology of tumors that shared the same molecular alteration to better understand the effect these changes have on the appearance of the tumor. With this work, the computer model has directed us to areas in- and outside the tumor that are important,” Bosse notes.
“In cancer diagnostics, the number of variables (molecular, tumour morphology, patient data) has increased exponentially and has complexified patient prognosis prediction. Through training unbiased AI models, AI predictions can also teach pathologists in return by, for instance, identifying novel morphological details on microscopy slide images with prognostic value,” says Sarah Fremond, first author of the new publication.
Source: Leiden University Medical Center
20.12.2022