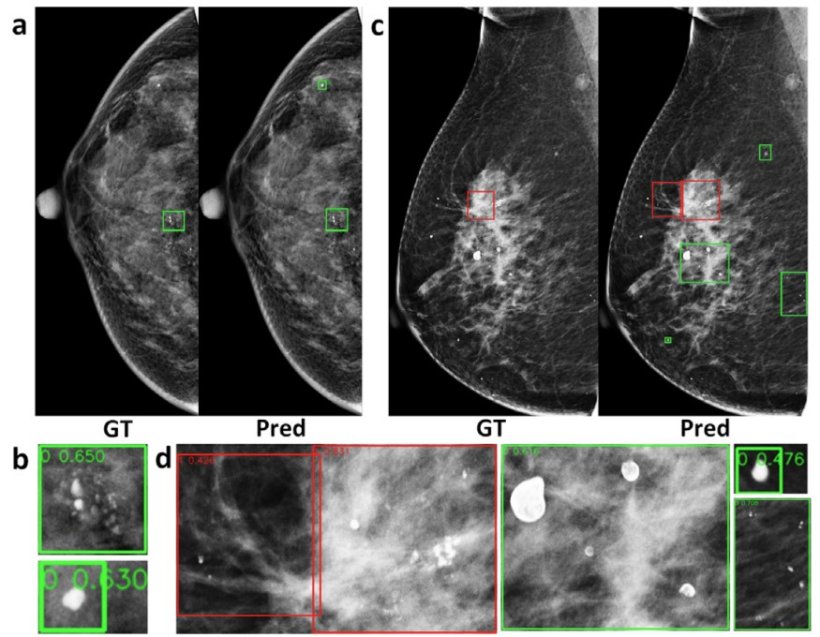
Image source: Lin Q, Tan WM, Ge JY, et al., Fundamental Research 2025 (CC BY-NC-ND 4.0)
News • Breast cancer diagnosis
AI classifies mammography microcalcifications
Each year, millions of women undergo mammography to screen for breast cancer, yet tiny calcium specks—known as microcalcifications—often evade detection or are misread, leading to delayed diagnoses or unnecessary biopsies.
Conventional computer-aided tools rely on hand-crafted rules and struggle with the sheer variety of imaging devices and lesion patterns.
In a recent study led by Dr. Ke-Da Yu from Fudan University Shanghai Cancer Center, a novel deep-learning approach that automatically finds and classifies microcalcifications across different machines and patient populations was developed—bringing both accuracy and consistency to breast-cancer screening. “Microcalcifications can be just a few pixels wide. Hence, spotting them amid normal tissue is like finding a needle in a haystack,” explains Dr. Yu. “We wanted a system that adapts to any mammogram and never overlooks early warning signs.”
By pre-marking suspicious regions on each mammogram, it enables radiologists to quickly focus on areas of concern, significantly reducing both missed diagnoses and unnecessary biopsies
Ke-Da Yu
The team’s approach rests on two key advances:
- Adaptive, multi-scale detection: By integrating a faster region-based convolutional neural network (R-CNN) model with a feature-pyramid network (FPN), the pipeline fuses features at multiple resolutions—enabling it to localize both coarse clusters and individual specks without any manually tuned thresholds.
- Robust, multi-center training: Trained on 4,810 biopsy-confirmed mammograms (6,663 lesions evenly split between benign and malignant) from three hospitals, the system automatically standardizes each image, so it works seamlessly across different scanners and clinical settings.
In blind testing, the pipeline processed each mammogram, achieving approximately 75% overall accuracy at the microcalcification-lesion level with 76% sensitivity for malignant lesions and about 72% accuracy at the breast level. “This solution can be deployed directly on standard radiology workstations,” adds Dr. Yu. “By pre-marking suspicious regions on each mammogram, it enables radiologists to quickly focus on areas of concern, significantly reducing both missed diagnoses and unnecessary biopsies—thereby easing patient discomfort and lowering healthcare costs.”
The research team has open-sourced the code, and their next steps will focus on integrating the system into clinical workflows, with the aim of offering a reliable AI-driven tool for more widespread breast-cancer screening.
Source: KeAi Communications Co., Ltd.
09.05.2025