Article • AI use in clinical diagnosis
Deep learning tool predicts tumour expression from whole slide images
A deep learning model to predict RNA-Seq expression of tumours from whole slide images was among the industry innovations outlined at the 7th Digital Pathology and AI Congress for Europe. Created by French-American start-up Owkin, the detail of how the company’s HE2RNA model provides virtual spatialization of gene expression was detailed to online delegates by senior translational scientist Alberto Romagnoni who highlighted its use in clinical diagnosis. During his presentation, delegates heard how Owkin has collaborated with doctors, hospitals and academic institutions to develop the tool.
Report: Mark Nicholls
The company says deep learning methods for digital pathology analysis are an effective way to address multiple clinical questions, from diagnosis to prediction of treatment outcomes. While these methods have also been used to predict gene mutations from pathology images, they suggest there has been little comprehensive evaluation of their potential for extracting molecular features from histology slides. Their findings show how the HE2RNA model, based on the integration of multiple data modes, can be trained to systematically predict RNA-Seq profiles from whole slide images WSI alone, without expert annotation.
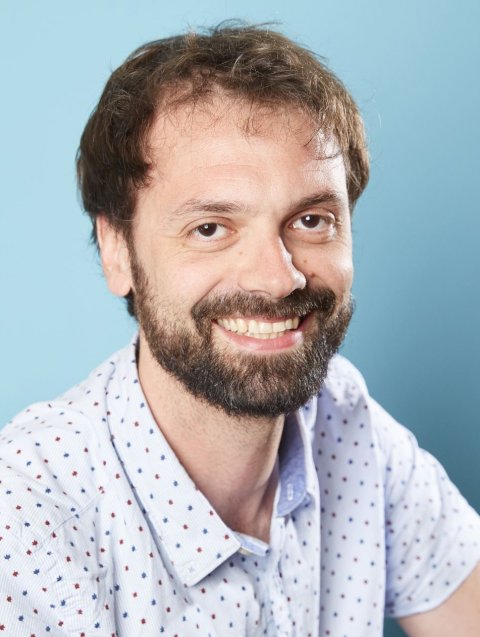
Dr Romagnoni, from Owkin’s translational research department, explained that the tool connects WSI with molecular data, and more specifically, transcriptomic data. He said: “Histology data of tumour biopsy sections are important tools in oncology, providing a high-resolution map of the tumour that helps pathologists determine diagnosis and grade. On the genomics side, massive changes in gene expression are known to occur in many cancers, secondary to mutations, or epigenetic modifications.”
However, with sophisticated methods such as next generation sequencing still not routinely used in a clinical setting, he suggested that prediction gene expression from WSIs could “greatly facilitate” patient diagnosis, prediction of response to treatment, and survival outcome. He said that HE2RNA has a multiplicity of applications with the model able to predict RNA-seq profiles from WSI without annotations to spatialize this information on the slide.
With the WSI divided into small tiles, features are extracted at tile level, while another neural network assigns a score to each tile for each gene in the genomic profiling. The patient level gene expression is calculated by aggregating the tile level score. Immunology related genes are particularly well predicted. Visual spatialization for each coding or non-coding gene can generate a heat map of slide level gene predictions. Examples of this include predicting MKI67 gene expression in liver cancer and epithelium-associated genes in prostate adenocarcinoma, with significant correlation.
The transcriptomic features extracted by HE2RNA are transferable to novel datasets and complement, and thus increase the performance of machine learning models, especially when trained on small cohorts
Alberto Romagnoni
Validation on MKI67, a well-known marker of cell proliferation, saw the model applied on an independent data set 369 slides from 194 patients with liver carcinoma and compared with tiling annotations performed by a pathologist. Higher MKI67 with tumour growth rate labelling index confers a fast progression and poor prognosis. “Tiles with a high expression of MKI67 were almost always located in tumoral regions,” said Dr Romagnoni “Among 10,000 tiles with the highest predictions, 94% were found in the tumoral areas. The model can also be used to improve predictive performance on other tasks in a transfer learning setting.
Questions and problems that can be address with HE2RNA, he continued, include genomic data augmentation, biomarker discovery, improved slide analysis with virtual staining, and increased performance on a variety of tasks such as learning to predict a transcriptomic representation on a cohort with a specific topic. “HE2RNA can provide insights into the local expression of genes without requiring expensive procedures and manipulation,” he added. “The transcriptomic features extracted by HE2RNA are transferable to novel datasets and complement, and thus increase the performance of machine learning models, especially when trained on small cohorts.”
Profile:
Dr Alberto Romagnoni is a Senior Translational Scientist with Owkin, a French-American startup, founded in 2016, that deploys Federated Learning to accelerate medical research. It works to empower researchers in hospitals, universities, and pharmaceutical companies to understand why drug efficacy varies from patient to patient; enhance the drug development process; and identify the best drug for the right patient to improve treatment outcomes.
15.01.2021