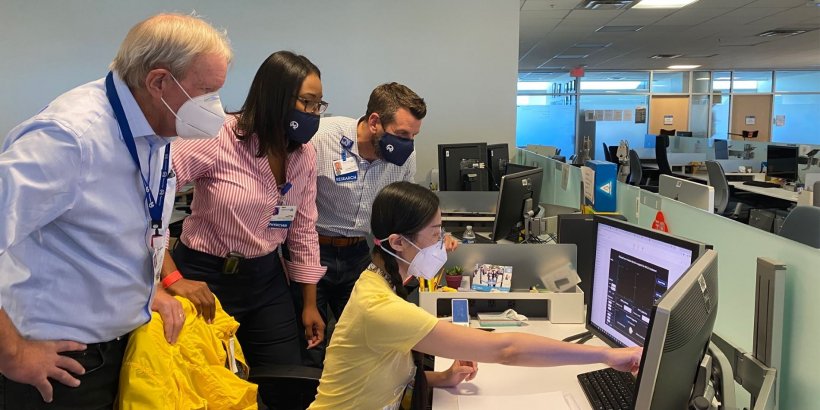
Article • Clinical decision support
AI deep learning of PET/CT images to support NSCLC treatment
A software tool to predict the most effective therapy for non-small cell lung cancer (NSCLC) developed by applying deep learning artificial intelligence (AI) to positron emission tomography/computed tomography (PET/CT) images has been developed by researchers at H. Lee Moffitt Cancer Center and Research Institute in Tampa, Florida. The tool is designed to provide a noninvasive, accurate method to analyze a patient’s tumor mutations and biomarkers and to determine the most effective course of treatment, making modifications as needed.
Report: Cynthia E. Keen
Over two million cases of lung cancer are diagnosed annually, according to the World Health Organization (WHO), approximately 85% of which are NSCLC. Two therapeutic strategies -- epidermal growth factor receptor (EGFR)-targeted tyrosine kinase inhibitors (TKIs) and program-death ligand (PDL-1) targeted immune checkpoint inhibitors (ICIs) are extending survival for patients with advanced NSCLC. Matching patients to the most effective therapy currently requires specimen-based assays. In addition to tissue-based biopsies being an invasive procedure, malignant tumors can be heterogeneous and the biopsied tissue used for testing may not be reflective of the entire tumor. Additionally, tumors can mutate during a course of therapy, rendering the initial treatment ineffective.
While liquid biopsy from blood collections may represent an alternative approach for tissue-based molecular testing, not all patients’ tumor markers can be detected in the blood
Wei Mu
For these reasons, there is a critical need to be able to accurately and noninvasively assess tumor EGFR mutational status to guide the use of TKIs, or PDL-1 expression status to guide the use of ICIs. Notably, patients who respond well to TKIs generally do not respond well to ICIs, and vice-versa. Thus, distinctions between the two are needed. Imaging the uptake of the radiotracer 18F-Fluorodeoxyglucose (18F-FDG) during a PET/CT exam can identify sites of abnormal glucose metabolism and help accurately to characterize tumors. Importantly, FDG PET/CT scans are commonly used for patient staging.
“18F-FDG PET/CT is widely used in determining the staging of patients with NSCLC, and this glucose radiotracer used is also known to be affected by EGFR activation and inflammation,” said Matthew Schabath, Ph.D., of Moffitt’s Cancer Epidemiology Department. “EGFR is a common mutation found in NSCLC patients. EGFR mutation status can be a predictor for treatment, as patients with an active EGFR mutation have better response to TKI treatment.”
“Prior studies have utilized CT-based radiomics as a noninvasive approach to predict EGFR mutation,” added Wei Mu, Ph.D., a postdoctoral fellow in the Cancer Physiology Department. “While liquid biopsy from blood collections may represent an alternative approach for tissue-based molecular testing, not all patients’ tumor markers can be detected in the blood. This creates the need that PET/CT-based radiomics may represent an opportunity,” commented Jhanelle Gray, M.D.,chair and program leader of the Thoracic Oncology Department.
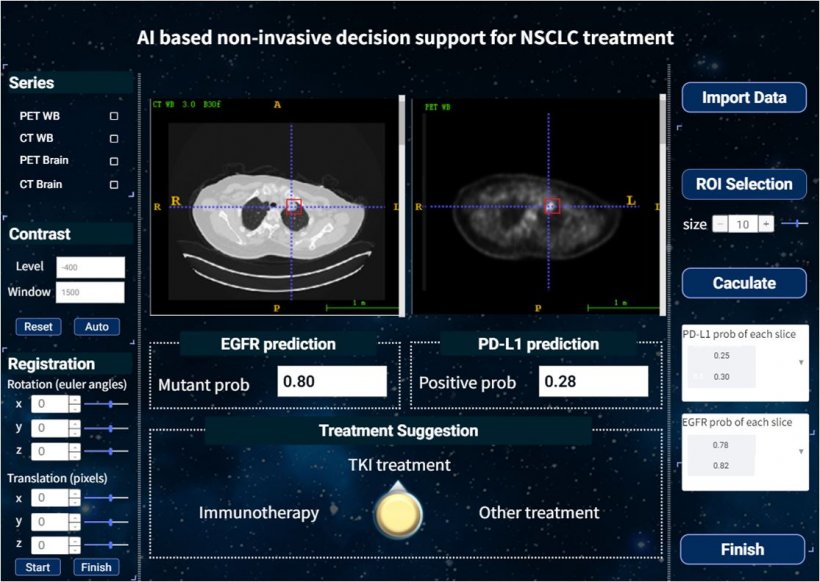
We [...] want to develop an internationally useful tool regardless of ethnicity, so we combined the data from China and the United States for our analysis
Robert J. Gillies
Led by Robert J. Gillies, Ph.D., chair of Cancer Physiology and vice-chair of Radiology, the researchers used data from 681 NSCLC patients treated at the Shanghai Pulmonary Hospital, the Fourth Hospital of Hebei Medical University in Hebei, China, the Fourth Hospital of Harbin Medical University in Harbin, China, and the Moffitt Cancer Center to develop and then validate the model. Dr. Gillies told Healthcare in Europe that data from China was generously provided to the Moffitt researchers as part of an international collaboration. “Recently, the incidence of lung cancer has increased rapidly in China. The mortality rate of lung cancer is also increasing there, while it has decreased gradually in the United States,” he explained. “Thus, there is renewed effort in China to routinely obtain genetic alterations assays in NSCLC patients, allowing us to enroll more valid data from Chinese institutions. Additionally, we also want to develop an internationally useful tool regardless of ethnicity, so we combined the data from China and the United States for our analysis.”
The 18F-FDG PET/CT-based deep learning model, described in Nature Communications, demonstrated high accuracy in EGFR mutation status prediction across all patient cohorts from the different cancer treatment centers. A high EGFR deep learning score was significantly and positively associated with longer progression-free survival in patients treated with EGFR-TKIs, and negative associated with response to ICI. Patients were further analyzed with a similar deep learning model to predict PD-L1 status, and those who scored high in this test had considerably longer progression free survival following ICI treatment than those who received EGFR-TKI treatment. The combination of these two scores can accurately identify the right treatment strategy for individual patients.
“Compared to other studies, our analysis yielded very high accuracy to predict EGFR mutation status and had many advantages. These included training, validating and testing the deep learning score with multiple cohorts from four institutions, which increased its generalizability,” commented Dr. Wu.
The researchers plan to share the model with other institutions for research studies as well as conducting additional studies themselves. “The tool serves as an innovation that can help to match and personalize treatments. We are working closely with Moffitt’s image analysis team to move prospective initiatives forward that will continue to build on these models,” said Dr. Gray. “We hope that our model may serve as a predictive biomarker to identify NSCLC patients sensitive to EGFR-TKI treatment and to identify patients most likely to benefit from ICI treatment. Due to the advantage of routine acquisition and lack of sampling bias of 18F-FDG PET/CT images, we prudently propose this model as a future clinical decision support tool for different treatments pending in larger and prospective trials,” the researchers concluded.
01.12.2020