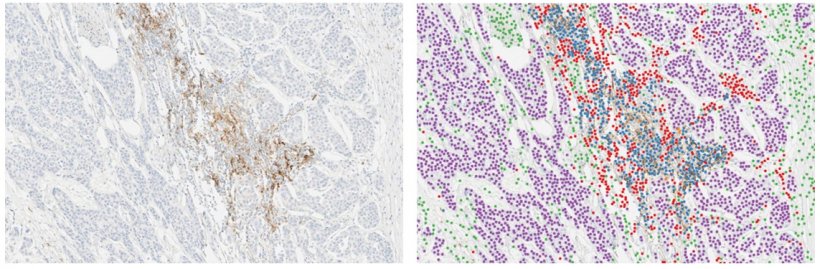
Article • Urothelial carcinoma
AI assists in PD-L1 scoring
A new deep learning algorithm, which demonstrates the potential of artificial Intelligence (AI) to support pathologists, has been developed for PD-L1 scoring in tumour cells and immune cells in urothelial carcinoma samples.
Report: Mark Nicholls
Speaking at the Digital Pathology and Artificial Intelligence Congress in London last December, Dr Michel Vandenberghe, from AstraZeneca, outlined how PD-L1 expression level assessment by pathologists can be used to help predict response to PD-L1/PD-1 targeted monotherapy. With the new deep learning algorithm, developed as part of AstraZeneca’s PostDoc programme, scientists there conducted experiments to establish whether AI can count the right cells in PD-L1 immunochemistry images and whether that information could provide some assistance to pathologists when assessing PD-L1 expression level. AI, he said, has potential to add value to tissue-based companion diagnostics (CDx), assisting pathologists in their objective of biomarker expressions, particularly in ambiguous cases.
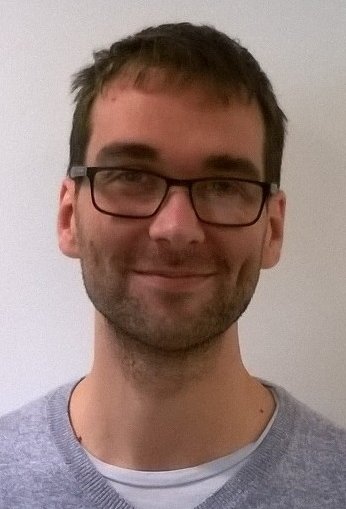
‘AI could alleviate some challenges in pathology, such as inter-pathologist variability and the predicted shortage of pathologists,’ Vandenberghe explained. ‘AI has the potential to assist pathologists to assess biomarker expression reproducibly, to reduce diagnostic delays and to increase access to testing, as well as to leverage big data to improve patient selection.’ Noting how deep learning has been transformational in image analysis and has enabled AI to be at least as good as humans at performing certain visual tasks and, in some cases, to become better than humans, he cited examples where AI was demonstrated to accurately diagnose skin cancer from photographs and to detect lymph node metastasis in breast cancer tissue slide images.
The congress heard more detail about the development and analytical validation of a deep learning algorithm for automated scoring of PD-L1 expression in urothelial carcinoma samples processed with the VENTANA PD-L1 (SP263) Assay. The approach that was implemented is based on an algorithm initially developed for interpretation of street scene images with applications for autonomous driving. ‘That posed the question,’ he explained, ‘of whether the same deep learning model could be trained for a different application, such as to detect and classify cells in immunohistochemistry images.’
The study demonstrates that the deep learning algorithm counts the right cells and is highly reproducible
Michel Vandenberghe
To train and evaluate the algorithm accuracy to count cells, the investigators manually annotated over 30,000 cells, which were used as ground-truth. ‘There is good-to-excellent correlation between automated cell counting using the AI system and manual counting across all the defined cell types,’ the scientist noted. The algorithm was then scaled up to count cells in whole slide images (WSI) to assess whether a given tumour sample is PD-L1 High or PD-L1 Low/Negative. AstraZeneca then conducted experiments to assess the analytical performance of the deep learning algorithm and demonstrated high reproducibility and repeatability of the algorithm for PD-L1 scoring in tumour cells and in immune cells.
The last experiment looked at the correlation between the AI system and a pathologist, where there was a strong correlation between the pathologist and the AI system for scoring PD-L1 in tumour cells and a weaker correlation when scoring PD-L1 in immune cells. Vandenberghe suggests the study contributes to building evidence for the use of deep learning to assist pathologists in the interpretation of PD-L1 expression for patient selection. ‘The study demonstrates that the deep learning algorithm counts the right cells and is highly reproducible,’ he said. ‘There was an 84% overall agreement between AI and pathologist-based scoring but the question mark remains over whether the pathologist or AI was right in the 16% of discordant cases, and whether AI can improve the scoring of difficult cases in routine diagnosis.’
Profile:
Michel Vandenberghe is Senior Scientist in the Precision Medicine Laboratories, Tissue Diagnostics, with AstraZeneca, and currently works on developing algorithms to analyse immunohistochemistry biomarkers.
20.06.2019