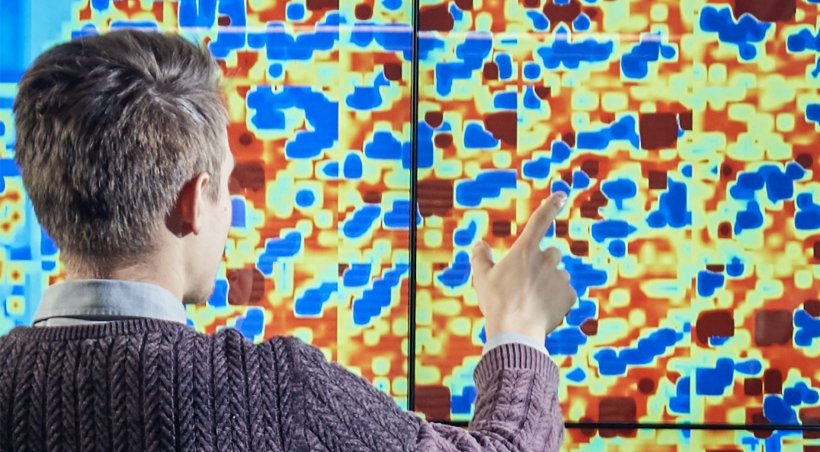
Photo: Markus Pentikaêinen
Article • Applications of machine learning
Training AI to predict outcomes for cancer patients
Predicting cancer outcome could help with a clinical decision regarding a patient’s treatment. In his keynote speech during the online ‘7th Digital Pathology and AI Congress: Europe’, Johan Lundin, Research Director at the Institute for Molecular Medicine Finland (FIMM) at the University of Helsinki and Professor of Medical Technology at Karolinska Institute, discussed ‘Outcome and biomarker supervised deep learning in breast cancer to predict survival and efficacy of adjuvant treatment’, citing his team’s investigation into whether a machine learning algorithm, trained with images of tumour tissue morphology only, can predict breast cancer ERBB2 gene amplification status and whether this prediction is associated with patient outcome.
Report: Mark Nicholls
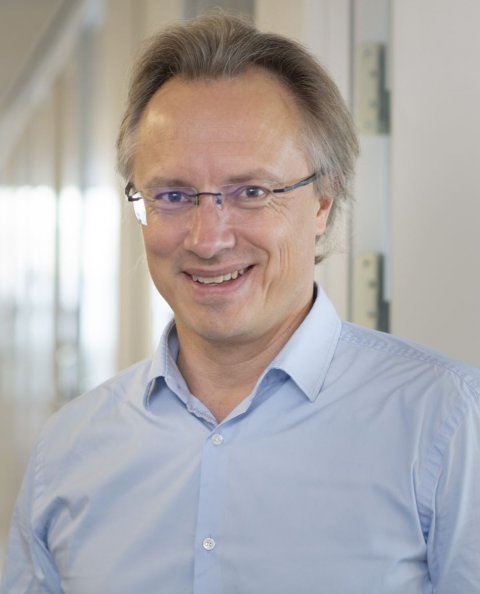
Photo: Ulf Sirborn
‘Our hypothesis is that training a machine learning classifier, supervised by outcome and biomarkers instead of expert-defined entities, has the potential to help discover new prognostic factors,’ Lundin explained. ‘Our results show that morphological features learned by the deep learning algorithm predict survival, molecular status of the tumour and efficacy of ERBB2-targeted therapy in breast cancer.’
While AI promises much in support, assistance, improved speed and accuracy, it will be interesting to see what happens when it goes beyond what the human clinician can do and starts making discoveries, he pointed out. ‘However, while AI and digital pathology can overcome human weakness, we are still far from overwhelming human strengths.’
What expert-supervised machine learning can do is identify features on a cellular and tissue level, although, once AI gets into the realms of predicting disease outcome and response to treatment, the question will be how much the subjectivity of the expert annotating the samples affects the predictive accuracy. ‘The challenge here,’ Lundin explained, ‘is the ground truth that you provide. For most of the supervised learning in digital pathology today, AI is just as good as the annotator, so the quality of the annotations is crucial.’ Already, there is potential to bypass the subjectivity; he outlined a recent study from his centre where an antibody supervised learning method, using stained samples from thyroid cancer, was used as ground truth instead of annotation performed by an expert. ‘This also allowed us to create high amounts of training data in a very short time and we showed, in a validation set of 250 images, including immune cells, that the accuracy on a pixel level was very high, which shows that this method should work.’ he added.
However, the big question remains as to whether AI is going to be just another subjective expert. ‘How you select and annotate training data is crucial, especially for expert-supervised algorithms,’ Lundin continued. ‘Ground truth is provided by human observations and will always be subjective. To get more reliable ground truth, should we use alternative and more objective endpoints than the expert annotations? ‘Maybe it’s time for a paradigm shift, as most of the algorithms so far created for pathology are based on expert interpretation. The whole idea of performing those are often that we want to make a prediction, a diagnosis, but that could also be a prediction of survival, risk of cancer recurrence and evaluation of the treatment efficacy.’
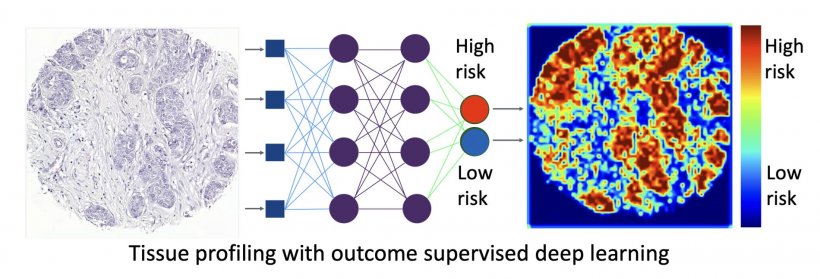
Photo: Lundin group
His question is was whether a middle step of human annotation is needed, or could it be replaced with machine learning, going directly from the sample to the prediction?
Lundin detailed how this has been examined by his team for direct outcome supervised learning with 420 colorectal cancer (CRC) and 1,300 breast cancer patients using microarrays where the long-term outcome was known. Pathologists performed a risk prediction visually before the images were put through a neural network trained to directly predict outcome. High discrimination was achieved using this outcome-supervised learning and in CRC, it was better than the consensus score of three experienced pathologists, demonstrating that valuable information can be extracted from the tissue. Further, the centre’s ERBB2 study of breast cancer patients was based on nationwide data from Finland, and saw the team train a set of 700 samples where they knew the ERBB2 status.
This is among the first studies to show an improved efficacy of a molecularly targeted therapy can also be predicted from the morphology based on features learned by AI
Johan Lundin
Having shown it is possible to predict ERBB2 status from the morphology with supervised learning, which was validated in samples from a randomised trial study on the effect of Trastumuzab treatment. Here, the algorithm could predict ERBB2 status from the morphology and also discriminate the patients who received Trastumuzab into two different prognostically different groups. ‘This is among the first studies to show that an improved efficacy of a molecularly targeted therapy can also be predicted from the morphology based on features learned by AI,’ the professor noted. ‘From these studies, we see that, using morphology and patient status as endpoints, you can clearly predict the survival and do it at the level of a highly-trained expert, and in some circumstances even better.’
Next steps will use deep learning attention maps to better understand what AI has learned in this context. ‘With the breast cancer study I have presented, we have shown that the deep learning algorithm trained on tissue morphology and supervised by molecular status can predict the efficacy of adjuvant therapy in patients with breast cancer, Lundin concluded. ‘We have also shown that it’s possible to extract the predictive information from routine tumour sections performed on every new patient, which means this is an interesting approach that can complement the molecular tests.’
Profile:
Johan Lundin is Research Director at the Institute for Molecular Medicine Finland (FIMM), University of Helsinki, Finland and Professor of Medical Technology at the Department of Global Public Health at the Karolinska Institute, Stockholm, Sweden. He is also co-founder of Aiforia Technologies, a FIMM spin-off company developing a cloud-based platform for AI-supported digital diagnostics within pathology.
15.01.2021