Article • Algorithms
Pattern recognition: Machines are learning fast
Language recognition on the smartphone, spam filters in the e-mail programme, personalised product recommendations by Amazon or Netflix – all share one feature: they are based on an algorithm that recognises patterns in a set of data. This artificial generation of knowledge is called machine learning.
Report: Michael Krassnitzer
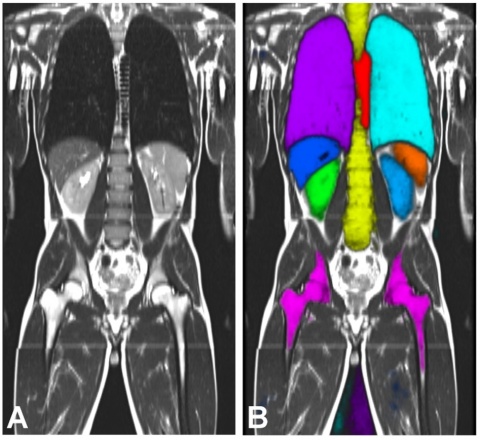
Radiology, in which huge data volumes are produced, is an increasingly important playground for machine learning. The analysis of quantitative image features in large medical databases is meant to allow statistic descriptions of tissue characteristics, diagnoses and course of diseases. At Imperial College, London, for example, a system called MALIBO (MAchine Learning In whole Body Oncology) is being developed that aims to detect tumours in whole-body MR images – without human intervention. So far, the system has looked at whole-body MRI scans of healthy volunteers to learn to identify organs and their components. The ‘teachers’ are radiologists who marked and named the structures to be recognised in the MRI scans. This enabled the systems to identify organs and their components fully automatically in new whole-body MRI scans.
Two different methods of machine learning were tested in this project: the principle of artificial neuronal networks that simulates the interaction of neurons in the brain, and so-called classification forests, i.e. large volumes of parallel decision-making paths. The former method was found to be superior to the latter. In the meantime MALIBO has entered phase 2, the recognition of primary tumours. ‘We have seen some good results in the detection of colorectal cancers,’ Dr Amandeep Sandhu Meng MBBS, a radiologist on the project team, reported.
Training an algorithm using 200 images
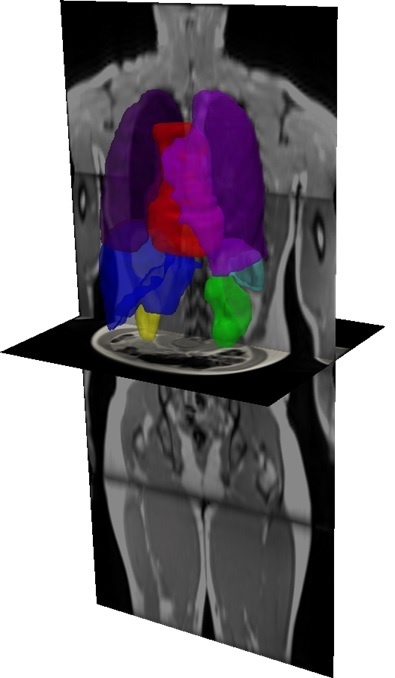
This ambitious research project is but one among many presented in the session on machine learning at the European Congress of Radiology (ECR 2017) in Vienna from 1- 5 March 2017. A team at the University of Valencia (Spain) developed an algorithm that can identify and characterise individual vertebral bodies on CT scans of spinal columns. The algorithm, which was ‘trained’ on 200 images of healthy and diseased spinal columns, assesses 90 percent of scans it has not previously processed correctly. At Bari University (Italy) a research team evaluated a computer-assisted decision-making system (CAD), developed to detect breast cancer lesions. Of 3,735 scans, 192 were considered suspicious, 102 were false positives, and four were false negatives. This promising predictive ratio would allow the CAD system to identify from large volumes of scans those breasts that do not require further examination.
Teams at the University Hospitals of Zurich and Basle, Switzerland, jointly developed an analytical tool that can predict osteoporosis risk from a multitude of radiological images from different sources and varying quality. Working with CT 179 images, dyed by radiologists, from 60 patients, the neuronal network learned to cull quantitative bone data. Moreover, at Basle University Hospital, PACS Crawler was developed – software to predict osteoporotic fractures risk from looking at CT scans Last, but not least, a research team at Mainz University Hospital developed an algorithm to predict liver transplant patient survival based on pre-operative 3-D CT scans. In 80 percent of the cases the algorithm correctly predicted which patients would survive surgery for more than one year. Strangely enough, the researchers don’t know how the algorithm arrived at its decisions. ‘That’s a bit like a crystal ball. We have no idea how it works – but it does work,’ said Dr Daniel Pinto dos Santos, Head of the research team Radiology Image and Data Science (radIDS).
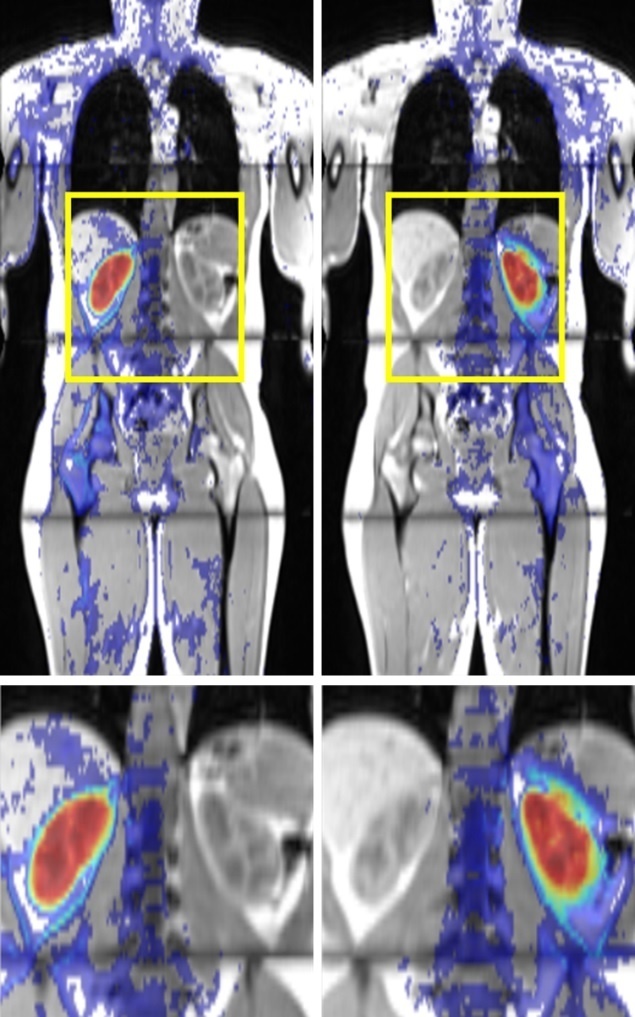
16.06.2017