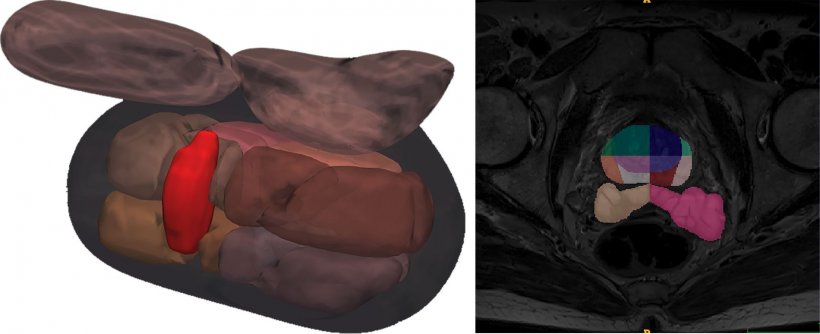
Right: Segmentation masks extracted automatically by Quibim's AI technology after the application of a multi-class CNN (transitional zone, peripheral zone, seminal vesicles) on T2 images trained with a database of more than 9000 samples.
Article • Improving detection accuracy
Fighting prostate cancer with over 1.5 million MRI images
Men die about five years earlier than women across the world. As initiatives to boost awareness of men’s health unfolded in November, an international project is bringing the forefront of AI research to tackle prostate cancer (PC), the second most frequent type of cancer in men and the third most lethal in Europe.
Report: Mélisande Rouger
Over the next four years, the ProCAncer-I consortium will receive €10M EU funding to develop advanced AI models to meet clinical needs in diagnosis, metastases detection and prediction of response to treatment, by creating the world’s largest PC MRI database and validating solid algorithms.
ProstateNet: over 1.5M images to display prostate cancer
15 institutions across the EU, Turkey and the UK will work to gather over 1.5 million prostate cancer images taken in 17,000 multi-parametric MRI examinations into a unique collection called ProstateNet. Building a strong dataset is a must to unleash the power of deep learning in clinical practice, according to Nickolas Papanikolaou, Principal Investigator in Oncologic Imaging at Antonio Champalimaud Foundation, in Lisbon, and Scientific Director of the consortium. ‘The highest dataset that is publicly available, so far, comprises a few hundreds of examinations. But, to provide meaningful outcomes, you need to provide thousands. The reason deep learning fails to provide clinical value now is that you need big datasets,’ he pointed out.
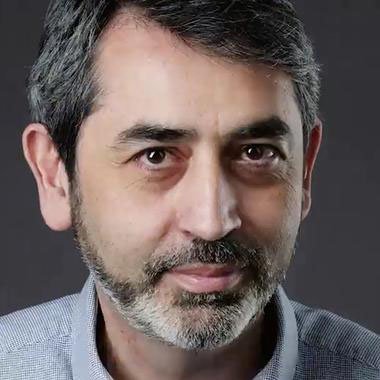
The project will count with the participation of renowned radiologists such as Daniele Regge, from the University of Torino, who serves as Clinical Director, and Emmanuele Neri from the University of Pisa, to name some. The consortium will also develop and maintain a centralised data repository, which will become available for third parties through controlled access. A special governance committee will be formed to examine applications and grant or refuse access.
The third goal is to develop AI models that can harness information contained in these huge amounts of data, to answer clinical unmet needs along eight defined clinical use cases tackling the whole disease continuum - from detection all the way to treatment-related prediction or toxicity. This holistic approach was probably the reason the project received EU support, according to Papanikolaou: ‘We believe we have exhausted all the important pitfalls and current limitations in clinical practice, and we will try to solve these pitfalls through technology use.’
Reducing overdiagnosis by improving detection of aggressive disease
Massive screening by means of specific biomarkers like PSA result in overdiagnosis and overtreatment. ‘Unlike breast cancer, where a screening program can really save lives, overdiagnosis of prostate cancer is the outcome of using PSA as a screening tool,’ he explained.
It’s absolutely crucial to be able to identify these few patients who have the highly aggressive form of cancer, because these are the ones we can save in principle if we treat them early
Nickolas Papanikolaou
PC is one of the most prevalent types of cancer, but most of these prostate cancers that are diagnosed through a biopsy are indolent – either they are very slow or don’t grow, staying confined within the gland. This means the vast majority of patients can live with the disease for two or three decades. The older a man gets, the higher his probability of developing PC. However, only 3% of those patients who are diagnosed with PC have the aggressive, life-threatening type. Being able to differentiate between aggressive and non-aggressive disease is therefore key. It’s absolutely crucial to be able to identify these few patients who have the highly aggressive form of cancer, because these are the ones we can save in principle if we treat them early,’ Papanikolaou pointed out.
MRI backed by AI can help detect, characterise and stratify those cancers into clinically significant or non-clinically significant. MRI is mandatory before prostate biopsy, because the modality helps improve prostate cancer detection and eases biopsy guidance. But’ Papanikolaou explained, ‘if there is only a radiologist to look at MRI images to evaluate PC, subjective variability remains a problem that AI can help to overcome. Radiologists don’t have the same diagnosis for the same patients and even disagree with themselves if you show them MRI images multiple times. Based on what radiologists say, a focused biopsy will be planned and the patient will be operated on. But radiologists now cannot significantly differentiate between non-significant disease and significant disease, and cannot really differentiate it biological-wise,’ Papanikolaou said.
Clinical validation and requirements
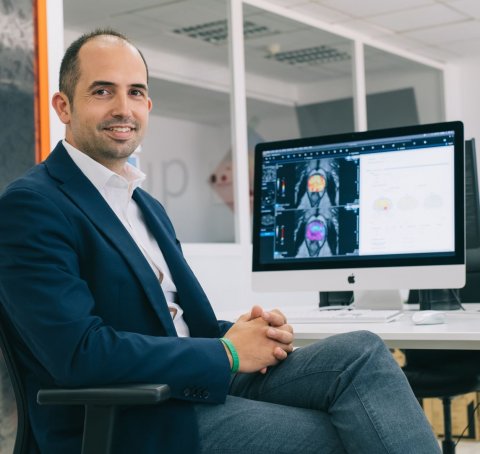
Photo: Kike Taberner
Clinical validation will be a critical stage of ProCancer I to make the results marketable. Medical imaging AI company Quibim will introduce its FDA-approved segmentation engines in the project dataset and provide risk management. ‘ProCAncer-I is a significant step forward in PC management,’ according to Angel Alberich-Bayarri, CEO and co-founder of the company. ‘We will use our experience from generalising our AI models to four important MRI manufacturers in hundreds of biopsy-proven cases.’
Quibim’s algorithm uses non-supervised AI models that can process bi-parametric and multi-parametric MRI acquisitions, taking the T2, DWI and DCE as inputs. The solution uses automated AI-based segmentation and then cluster-ises voxels of the transitional zone, peripheral zone and seminal vesicles. Clustering is based on T2 hypointensities/textures, ADC values and Ktrans values, if available. The output is a nosologic map, where the suspicious regions are highlighted and their aggressiveness scored.
ProCancer-I will benefit from Quibims newest product, to be launched in January, to accelerate reporting of prostate MRI analysis and make it more accessible to radiologists, by providing detailed segmentation of prostate anatomy and seminal vesicles combined with the automated detection of lesions, Alberich-Bayarri said. ‘Prostate MRI scans are growing dramatically but few radiologists per department are at the top of the learning curve. Our solution will have a direct impact in shortening the learning curve of radiologists and also improving their accuracy in detection of cancer, to better guide biopsies and avoid those that are unnecessary,’ he concluded.
Profiles:
Nickolas Papanikolaou is Principal Investigator in cancer imaging at the Antonio Champalimaud Foundation in Lisbon, Portugal, where he works on the development of radiomics. He is affiliated researcher at the Institute of Computer Science (ICS), one of six institutes in the Foundation for Research and Technology - Hellas (FORTH), a major national research centre in Greece. Papanikolaou trained in biochemical engineering at the Technological Educational Institute of Athens, and has worked extensively in medical imaging and has lectured in radiology conferences for over 20 years. (Papanikolaou also gained a Diploma in Enterprise and Entrepreneurship from the London School of Business and Finance.) An experienced researcher, he also works in higher education, and is currently a visiting researcher at the Karolinska Institute.
Ángel Alberich-Bayarri is CEO & co-founder of Quibim (Quantitative Imaging Biomarkers in Medicine), a spin-off dedicated to the advanced analysis of medical images using AI in Valencia, Spain. A telecommunications engineer who specialised in electronics, in 2010 he earned his PhD in biomedical engineering from the Polytechnic University of Valencia for his research on the application of advanced image processing techniques to MRI. He previously was Scientific-Technical Director of the Biomedical Imaging Research Group at La Fe Health Research Institute and R&D engineer and coordinator of biomedical engineering for Grupo Hospitalario Quirón, the leading private healthcare provider in Spain.
03.12.2020