Article • Digital pathology
VIPR: Deep learning for small cohorts
To investigate rare diseases, applying image-based analytics approaches, including the use of deep learning convolutional neural networks (DL-CNNs), can be a major challenge due to great difficulties in acquiring sufficient numbers of cases and associated digital image sets from the small cohorts typically available.
Report: Mark Nicholls
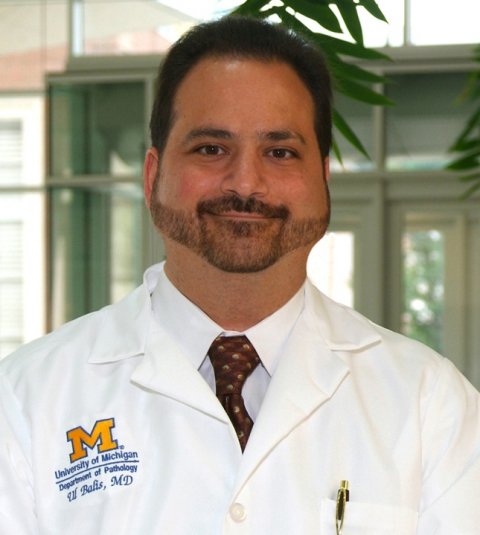
To realise algorithms that are both effective and generalisable, conventional DL-CNN algorithms typically require hundreds to thousands of cases and larger yet numbers of image tile ensembles. In the case of uncommon or rare diagnostic entities, such as encountered in the routine practice of pathology, this data shortfall can be an obstacle. However, Professor Ulysses Balis believes that the use of a particular type of data augmentation technique, as a pre-processing stage to subsequent DL-CNN use, could hold the key and offer a solution to the challenge posed by smaller cohort sizes.
Speaking at the 6th Digital Pathology & AI Congress in London last December, he advocates the use of VIPR (Validated Identification of Pre-screened Regions), a particular type of spatial data augmentation technique which transforms an image’s raw pixels into a local kernel figure-of-merit heat map.
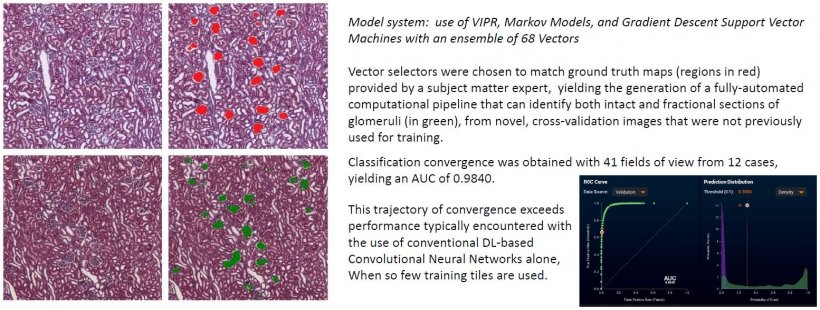
When used as a pre-processing step to subsequent use of DL-CNN approaches, his group has observed that the resulting ensemble-based algorithm can converge to a robust image classification and segmentation solution with far fewer cases and images, than encountered with use of DL-CNNs alone. This combination can provide a workable approach matching the diagnostic cohort sizes as encountered in histopathological entities.
Balis explained how the VIPR-based data augmentation technique spatially prequalifies image regions with a supervised figure of merit, allowing for subsequent deep learning pipeline stages to benefit from enhanced foreground delineation. This image augmentation approach uses an initial set of prototypic local image kernels as the starting point for their subsequent equivalence testing throughout the entire surface are of image sets under interrogation, ultimately yielding a pixel-level heat map indicating all areas that are similar (from textural, luminance and colorimetric aspects) to the initial search predicate(s).
If you have a small cohort of cases, you have the challenge of having the algorithm converge upon a generalised solution
Ulysses Balis
Use of VIPR allows thousands of training images to be reduced to hundreds or even tens, and Balis suggests that, by use of VIPR-based heat maps as an intermediary synthetic image, satisfactory performance from DL-CNNs, including their exhibiting of more rapid algorithm convergence, can be realised on the small image sets that pathology routinely encounters. ‘It’s effective for rare entities where only small cohorts of images or ROIs are available,’ he added. ‘It potentially allows pathologists to operate at their highest credentialed level of practice, given the interactive, real-time nature of the tool, which operates in the spatial domain with an intuitive, exemplar-driven model.’
During his presentation ‘Augmented Deep-Learning Pipelines with Use of the VIPR Algorithm to Realise Histology Image Segmentation/Classification in the Setting of Smaller Training Image Sets,’ Balis explained: ‘If you have a small cohort of cases, you have the challenge of having the algorithm converge upon a generalised solution. Many contemporary AI approaches that address image classification make use of some variant of deep learning, but deep learning typically requires many images or image tiles to allow for convergence upon a robust and generalisable solution.’
With many diagnostic entities in pathology offering case cohort sizes that are too small in number to qualify for direct application of deep learning techniques alone, Balis believes the use of a pre-processing stage at the pixel level – with VIPR being one such example will help overcome that. He feels that image data augmentation techniques, in general, and VIPR specifically, can serve as the vehicle by which smaller numbers of images can be suitably magnified in their representational content of foreground subject matter of interest, as a means of extending the stochastic likelihood that a DL-CNN-based classifier will recognise the intended features of interest.
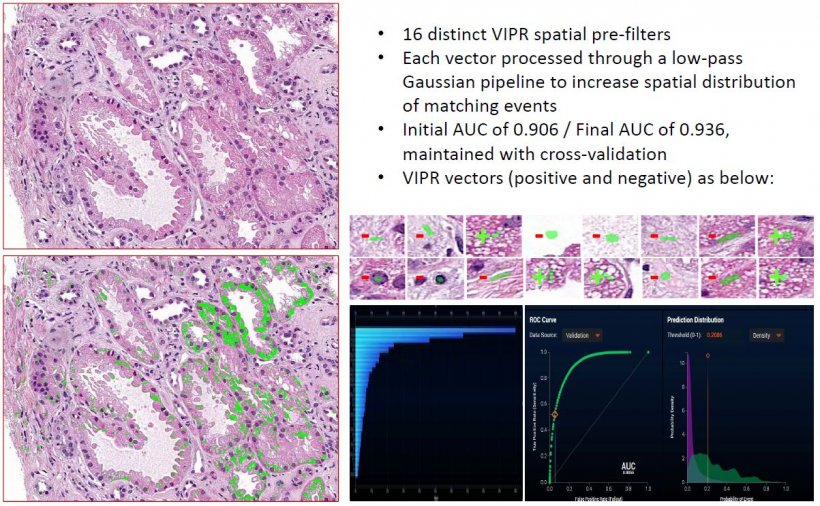
Deep learning pipelines with pre-processing steps purposefully designed to operate within the constraints of the known small cohort sizes of histopathology entities are compelling
Ulysses Balis
This approach effectively shifts deep learning’s unsupervised learning mode to a supervised learning mode, but without the typically associated heavy burden of manually generating hand-drawn ground truth segmentation maps. ‘That’s VIPR’s role,’ he said, adding: ‘We can transform a raw histology image into a spatial roadmap where individual pixels are transformed from merely conveying local luminance information, to their representing a local domain goodness-of-fit for one or more bespoke image features, thus realising a powerful image pre-processing step.’
In 97% of instances tested so far (over 850 predicates), he said VIPR will converge on a solution with AUC >0.90, with fewer than 10 vectors. ‘This overall approach appears to exhibit robust performance, even for novel and cognitively challenging annotation exercises, and even in the setting of small cohorts of cases and image set sizes,’ Balis said. ‘Deep learning pipelines with pre-processing steps purposefully designed to operate within the constraints of the known small cohort sizes of histopathology entities are compelling, in that they can offer the possibility of [algorithm] classification convergence in more instances than with the use of deep learning approaches alone.’
Profile:
Ulysses Balis is Professor of Pathology and Director of the Division of Pathology Informatics at the University of Michigan. His long-standing interest lies in the intersection of engineering, computational approaches and the practice of medicine. He has research interests in several areas of pathology and medical informatics including machine learning and the use of encoded data, image-based analytics, machine vision tools for histopathology and image-based search algorithms.
13.07.2020