Article • Tools for practitioners
Computational pathology: Heading for personalised medicine
Computational pathology has increased applications for diagnosis, prediction of prognosis and therapy response, facilitating the movement of healthcare towards personalised medicine.
Report: Mark Nicholls
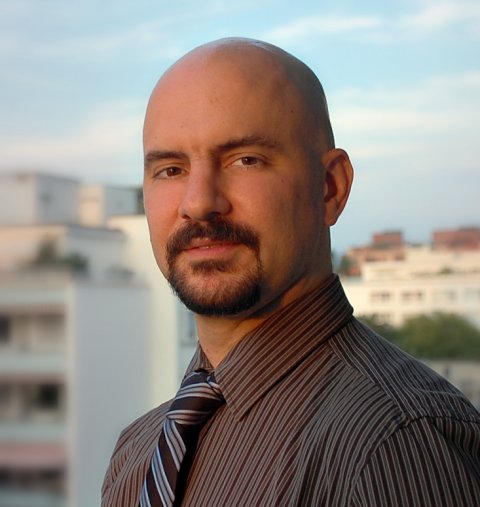
Coupled with deep learning, such tools are ever more efficient and robust within research and clinical settings. The growing role of computational pathology was highlighted by Professor Andrew Janowczyk at the Digital Pathology and AI congress in London last December. In his presentation, ‘Computational Pathology: Towards Precision Medicine’, Janowczyk – an Assistant Research Professor in The Centre of Computational Imaging and Personalised Diagnostics (CCIPD), Case Western Reserve University in Cleveland, Ohio – outlined results from recent research employing digital pathology in the domains of oncology, cardiac care, and nephrology as well as development of user-friendly open-source tools for practitioners.
This will not put pathologists out of business but will transition them to a different type of more integrative work
Andrew Janowczyk
Computer-aided diagnosis (CAD) – using algorithms to help clinicians analyse clinical data – has become increasingly useful in improving the efficiency and robustness of medical diagnosis because it is fast and reproducible and can leverage vast amounts of data that already exists, Janowczyk explained. Notably, with the increase in the number of digital slide scanners, more data is being created daily, at an ever-increasing rate, further fuelling these approaches. This data, he said, can be used to perform data mining to identify trends; identify subtle image patterns which may not be visually discernible; and build systems to aid – not replace – doctors through decision support. ‘This will not put pathologists out of business,’ he stressed, ‘but will transition them to a different type of more integrative work.’
CAD can be divided into clinical applications and research, Janowczyk pointed out. Clinical applications include the ability to recapitulate and automate existing processes; support cancer detection, grading, counting and area estimation tasks; deliver improvements through quantification and reproducibility, and disease definitions, and help with visual confirmation. For research, algorithms can be used to develop novel features and metrics enabling: sub-type discovery, biological elucidation, and deliver improvements through augmenting current clinical knowledge via new insights. However, he suggested that CAD research and clinical applications are separated by at least a decade.
He used breast cancer risk recurrence score as an example. With a 21-panel gene-expression assay, costing $4,600 a test, taking two weeks to complete and resulting in tissue destruction, he compared that with an image-based risk score based on routine slides that would be expected to cost two orders of magnitude less, be available within minutes, performed locally and be non-tissue destructive. This is realistic, Janowczyk suggested, given that the individual components of the current clinical grading scheme (Nottingham histologic score) are already prognostic and the increased precision afforded by leveraging computation algorithms will only add to their performance.
There are a number of reasons, he added, to use deep learning because it is significantly faster to implement compared to the development of hand-crafted features, and shows ‘great robustness’ because it can examine more cases than a typical developer could. With deep learning, the robustness of many common image analysis tasks in digital pathology (e.g. cell counting, area estimation) is greatly improved.
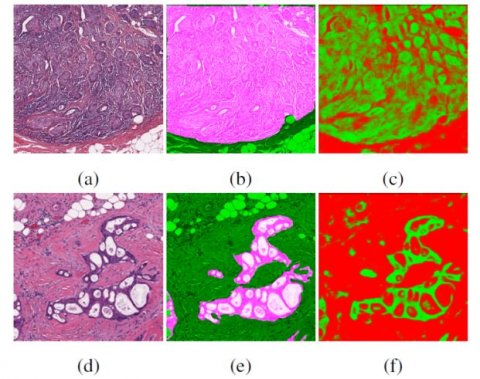
Creating algorithms that perform well can carry out labour and time intensive tasks for which the pathologist manpower is not available to do (e.g. segmenting individual epithelial regions). In addition, algorithms may be able to identify and validate patterns which humans are not capable of quantifying easily, such as slight differences in textures or morphologies. However, he acknowledged that CAD is patient-based and thus it can take years to find patients with rare diseases. Janowczyk also stressed that improved experimental design, and thorough validation will be needed with new generation tools, because concerns raised by the black-box nature of deep learning are warranted.
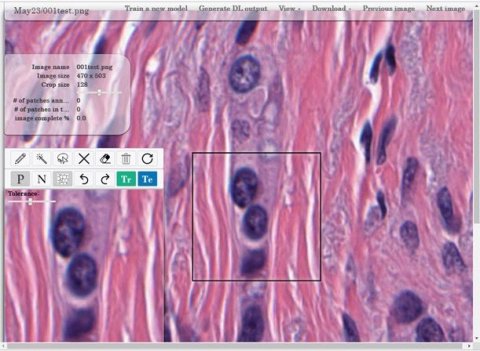
Finally, he focused on tools produced by the CCIPD to support precision medicine advances. These open-source tools include the HistoQC, which addresses the unmet need for quality control by identifying and visualising slides of poor quality, thus enabling them to be recut and rescanned immediately before getting into a workflow to a pathologist. He added that HistoAnno, a tool which employs deep learning-based active learning for rapid annotation generation, is expected to be released in early 2020.
Profile:
Dr Andrew Janowczyk is an Assistant Research Professor at the Centre of Computational Imaging and Personalised Diagnostics at Case Western Reserve University, and a senior research scientist in the Department of Personalised Oncology at CHUV (Switzerland). He is also a Senior Bioinformatician with the Swiss Institute of Bioinformatics. His research focuses on applying machine learning and computer visions algorithms to digital pathology images for disease detection, and the prediction of prognosis and therapy response.
23.04.2020